Model of baddeleyite recovery from dump products of an apatite-baddeleyite processing plant using a CVD6 concentrator
Abstract
The paper is devoted to developing a model of baddeleyite recovery from dump products of an apatite-baddeleyite processing plant using centrifugal concentrators. The relevance of the work arises from the acquisition of new knowledge on the optimization of technological parameters of centrifugal concentrators using Knelson CVD (continuous variable discharge) technology – in particular, setting the frequency of valve opening and the duration of valves remaining open. The purpose of the research was to assess the applicability of CVD technology in the treatment of various dump products of the processing plant and to build a model of dependencies between the concentrate and tailings yields and the adjustable parameters, which will allow to perform preliminary calculations of the efficiency of implementing this technology at processing plants. The research objects are middling and main separation tailings of the coarse-grained stream and combined product of main and recleaner separation tailings of the fine-grained stream. The study uses general methods of mathematical statistics: methods of regression analysis, aimed at building statistically significant models, describing dependence of a particular variable on a set of regressors; group method of data handling, the main idea of which is to build a set of models of a given class and choose the optimal one among them. Authors proposed an algorithm for processing experiment results based on classical regression analysis and formulated an original criterion for model selection. Models of dependencies between the concentrate and tailings yields and the adjustable parameters were built, which allowed to establish a relationship between the concentrate yield and the valve opening time, as well as a relationship between the tailings yield and the G-force of the installation.
Introduction
The Kola region is one of the oldest mining regions in Russia. In the Soviet period, it became a key producer of several strategic minerals (iron ore, nickel, copper, apatite, zirconium) and has retained its role to this day [4]. The Kola region has one of the richest resource bases in Russia: 64 chemical elements were found in industrial concentrations, more than 30 of them are being extracted by mining plants. Paper [13] describes the state of the region's mineral resource base in more detail.
The presence of a large number of valuable components leads to construction and operation of mining and processing plants (MPPs). More than 10 plants are located on the Kola Peninsula: Kola Mining and Metallurgical Company, Severonikel, Lovozersky MPP, Olenegorsky MPP “Olkon”, Apatit, Kovdorsky MPP, etc.
It is known from open literary sources [7, 13, 16] that the operation of mining and processing plants, including the ones located in the Murmansk region, leads to accumulation of technogenic mineral raw materials. Normally such products can contain valuable components, which with the use of modern technologies can be extracted with a great economic effect. An example of technological research on the processing of tailings containing a significant amount of valuable components is presented in paper [1]. In addition, processing of technogenic raw materials reduces negative impact on the environment [9, 11, 14]. An example of scientific research that takes into account climatic characteristics of the region is presented in paper [15].
One of the largest enterprises in the Kola region is Kovdorsky Mining and Processing Plant. The interest in this plant is based on the rationality of processing baddeleyite-apatite-magnetite ores with a complex mineral composition to obtain the respective concentrates [3]. JSC “Kovdorsky Mining and Processing Plant” is the second largest producer of apatite concentrate in Russia and the only producer of baddeleyite in the world. Moreover, the plant is one of the few enterprises, actively involved in the processing of tailings for the purpose of increasing economic efficiency of the company.
From the end of 1990s, according to the technology, developed at the Mining Institute of the Kola Science Center of the RAS, JSC “Kovdorsky MMP” began a large-scale development of the technogenic deposit I (mature tailings from the field I of the tailing dump), formed by the processing waste, accumulated in the previous years of magnetite processing plant operation, with the release of high-quality apatite and baddeleyite concentrates [2, 5, 6]. Presently, the enterprise has initiated laboratory research on the treatment of dump products from the apatite-baddeleyite processing plant (ABPP) [8] – in particular, on the additional recovery of baddeleyite – at the premises of TOMS Institute (Irkutsk), which became the foundation for the expanded tests. One of the main operations in the proposed technology was the use of centrifugal separation.
Ongoing work on setting up and optimizing operation of mining and processing equipment, including centrifugal separators, is quite expensive; therefore, obtaining values and statistical dependencies allows to perform preliminary calculations of the efficiency of implementing new technologies at such plants [10].
The purpose of the research was to assess the applicability of CVD technology in the treatment of various dump products of the processing plant and to build a model of dependencies between the concentrate and tailings yields and the adjustable parameters.
Research objectives included conducting semi-industrial tests on various ABPP products using the process of centrifugal separation, obtaining dependencies between the recovery of valuable components and technological parameters of the equipment, developing an algorithm for processing test results based on classical regression analysis.
Research methods
The experiment was carried out using a semi-industrial installation on the basis of KC-CVD6 concentrator. Three technological streams were prepared for semi-industrial tests at ABPP: middling separation tailings of the coarse-grained stream; main separation tailings of the coarse-grained stream; combined product of the main and recleaner separation tailings of the fine-grained stream.
The test procedure was based on several stages, one general (preparatory) stage and three stages for each technological stream.
The first, preparatory, stage was carried out regardless the feed flow. The main task of this stage was to select the optimal opening time of the pinch valves, i.e. the period during which the valves in the concentration ring would open, close and completely discharge the accumulated concentrate. At the same time, a minimum amount of barren unprocessed rock, simultaneously passing through the discharge ring of the concentrator, should go into the concentrate.
The optimal time cannot be the same for every test at different plants. This is explained by the fact that even hundredths of a second in an open state significantly affect quantitative characteristics of a single discharge [12]. Opening and closing time depends on multiple factors: temperature of ambient air and water, air pressure in the system, state of the valve rubber, rotor speed, as well as technical condition of the system as a whole [12]. Therefore, at the first stage a number of experiments were carried out to adjust the time and add these parameters to the control system.
Further tests for each technological flow consisted of four stages:
- fluidization water flow rate – adjusting the parameters of fluidization water flow rate based on estimating the grade of the valuable component in the concentrator products;
- adjustment of G-force – selecting the parameters of gravitational acceleration G (adjustment of rotor speed) based on estimating the grade of the valuable component in the concentrator products;
- plotting a separability curve in the course of experiments with different concentrate yield using data from two previous research stages in order to gradually reduce concentrate yield and improve its quality;
- selecting optimal operation modes for the concentrator, verifying the indicators in the course of semi-industrial processing – performing long-running tests (3-6 h) in order to confirm the values, obtained in previous tests.
The research methodology was based on theoretical foundations of mineral processing. Material composition of the concentration products was studied using atomic emission spectrometry; mineral composition was examined by optical methods in polished and transparent thin sections.
Test on the middling separation tailings of the course-grained stream
During the tests, the results of processing the tailings of the middling line with the circulation of concentration products from the shaking tables were obtained. In the first two stages, the mode of fluidization water supply (25 l/m) and the rotor speed (G-forces of 75 and 90) were selected. Feed density was regulated in the range of 20-35 % solids. The processing of ore was efficient – at a concentrate yield of 10 %, the recovery was more than 50 %, the degree of concentration was 4-5. Based on the test results, a recovery curve was plotted (Fig.1).
In the course of the experiment, it was decided to change the rotor speed, and, accordingly, the G-force; as a result, recovery curves were obtained at 75G and 90G (Fig.2).
It was estimated that the concentrate yield and recovery were higher at 90G acceleration. The grade of the concentrate and the degree of concentration were inversely proportional to the concentrate yield. In a single operation, the maximum degree of concentration reached 6.19, while the grade of the concentrate amounted to 1.3-1.5 % of ZrO2.
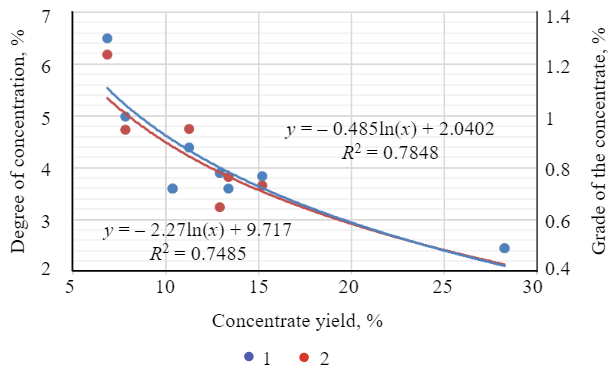
Fig.1. Dependence between the degree of concentration/grade of the concentrate and the product yield 1 – degree of concentration; 2 – grade of the concentrate
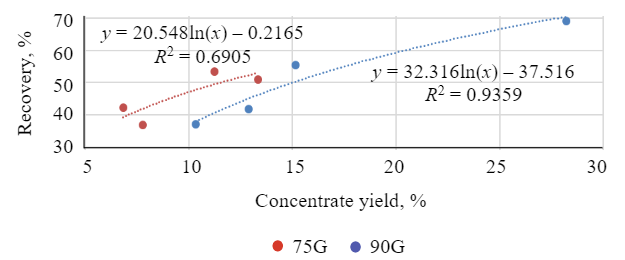
Fig.2. Recovery curve for the middling separation tailings of the coarse-grained stream
Additional tests were performed to obtain the concentrate for subsequent recleaning. Analysis of the grade of the valuable component showed that the bulk of baddeleyite in the feed was concentrated in the fine classes. The concentrator effectively extracted baddeleyite from the classes of –0.071 and –0.044 mm. The highest concentration ratio and recovery rate were recorded in the fraction
–0.044 mm, which was probably associated with the maximum liberation of the mineral in this class.
Tailings of the middling coarse-grained stream proved to be a promising material for baddeleyite recovery using centrifugal separation. The bulk of the mineral is concentrated in the fine classes: 90-95 % of ZrO2 in the class of –0.20 mm, 65-70 % of ZrO2 in the class of –0.071 mm. Since the recovery in the coarse classes is insignificant, it can be assumed that the valuable mineral in these classes is associated with barren rock and requires additional operations for breaking the raw material.
Test on the main separation tailings of the coarse-grained stream
In the first two stages, the mode of fluidization water supply and the rotor speed (G-forces of 75) were selected.
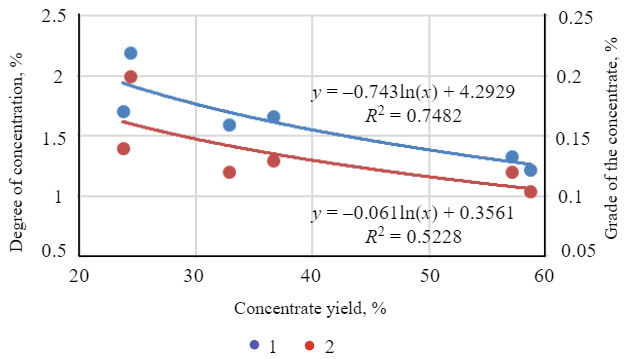
Fig.3. Dependence between the degree of concentration and the product yield 1 – degree of concentration; 2 – grade of the concentrate
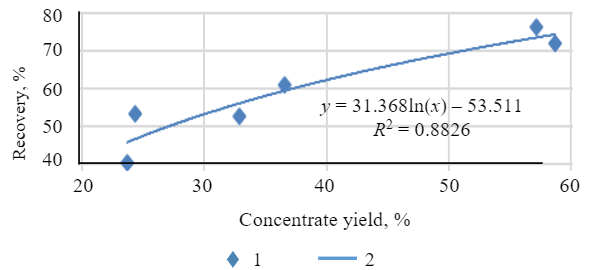
Fig.4. Dependence between baddeleyite recovery and concentrate yield 1 – recovery curve; 2 – logarithmic recovery curve
Based on the results obtained, it was identified that the main separation tai-lings of the coarse-grained stream were processed less effectively and had a lower degree of concentration, and although the recovery remained high, the degree of concentration roughly equaled 2 (Fig.3). The plot (Fig.4) shows a reco-very curve.
In a single operation, the maximum degree of concentration reached 2.2, while the grade of the concentrate amounted to 0.2 % of ZrO2. Similarly to the first stage, particle size distribution of the valuable component was analyzed. It was estimated that the bulk of baddeleyite (over 50 %) was concentrated in the fine classes (–0.071 mm). The main increase in the recovery and grade of the valuable component was also observed in the fine classes of –0.071 mm.
Tailings of the main coarse-grained separation are difficult to process in a centrifugal separator. The cause probably lies in a low concentration of the valuable component in the original product feed, coarser particle size distribution and a greater number of mineral aggregates. The bulk is concentrated in the fine classes: 85-90 % of ZrO2 in the class of –0.20 mm, 60 % of ZrO2 in the class of –0.071 mm; the major share of the valuable component is recovered from these classes. Coarser fractions are probably represented by intergrowths, hence the recovery and concentration in them are minimal.
Test on the combined tailings of main and recleaner fine-grained separation
Performance of semi-industrial tests on this feed flow was complicated by the problems of setting up a stable feed supply, impossibility of achieving the required density and low mass fraction of the feed.
In the first two stages, the mode of fluidization water supply (25 l/m) and the rotor speed (G-forces of 75 and 90) were selected.
The processing of baddeleyite is characterized by poor recovery (Fig.5); however, with a decrease in the concentrate yield, its quality increases more noticeably than in the main separation tailings of the coarse-grained stream.
It was estimated that in a single operation the maximum degree of concentration reached 2.5 %, while the grade of the concentrate amounted to 0.35 % of ZrO2 (Fig.6).
Based on the studies that estimated particle size distribution of the valuable component, it was identified that, similar to the previous tests, the main concentration of the mineral was in the fine classes. However, this feed product of the concentrator was characterized by a finer particle size distribution, and the major share of the valuable mineral (up to 75 %) was concentrated in the class of 0.044 mm.
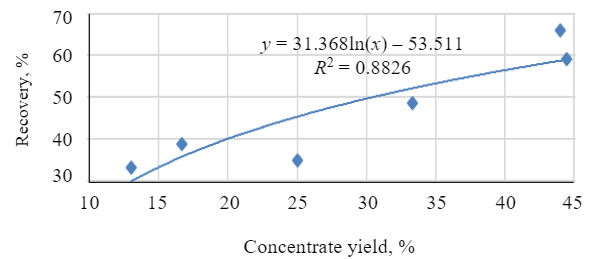
Fig.5. Dependence between baddeleyite recovery and concentrate yield For the legend, see Fig.4
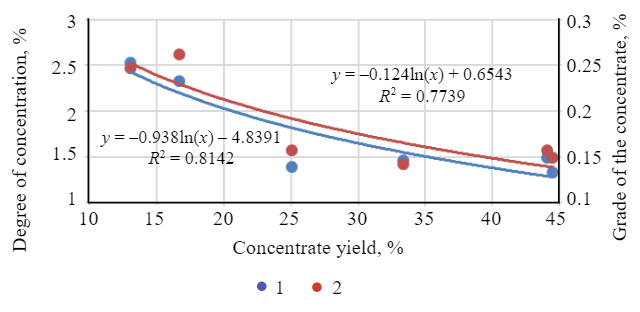
Fig.6. Dependence between the degree of concentration and the product yield 1 – degree of concentration; 2 – grade of the concentrate
Based on performed studies of processing various products, it can be argued that fine-grained separation tailings are the product that is the hardest to process using centrifugal concentration. The bulk of the valuable component is concentrated in the class of –0.044 mm, but the yield in the concentrate does not exceed 20 %. Probably, large particles of barren rock exert a negative influence on the movement of the fine fraction, which contains the major share of the valuable component in the concentrator bed. This is the reason behind the fact that in this product it is rather difficult to raise the degree of concentration above 2.5 in a single operation.
Results
The treatment of ABPP dump products shows that, despite the fact that the products under the conditions of a centrifugal field are processed in different ways, with varying degrees of concentration and have different particle size distributions, their common trait is that the bulk of the valuable component is recovered to the concentrate from the fine classes.
Grain size analysis of the material and the obtained products demonstrates that up to 90 % of the valuable component in the combined tailings product in the liberated form is concentrated in the class of –0.1 mm; hence, limiting the class of the feed to –0.1 mm can increase the grade of the valuable component in the concentrator feed, which will allow to obtain a higher-quality concentrate under the same degree of concentration. Limiting the grain size will allow to reduce the influence of coarse barren rock particles on the quality of ZrO2 separation.
Considering this issue from a practical point of view, under the current product supply of 1,000 t/h in the –0.1 mm class, the screening of the stream will require significant capital costs and probably will not achieve the desired efficiency. Evaluation of centrifugal separation performance in the primary processing of tailings allows to draw a conclusion that with a total concentrate yield of 20-25 %, about 40-50 % of ZrO2 can be recovered to the primary concentrate across all streams.
The indicators of ZrO2 recovery, obtained as a result of industrial tests of the KC-CVD6 installation at Kovdorsky MPP, can be processed using mathematical statistics – in particular, regression analysis methods – in order to build mathematical models that can adequately describe recovery of the valuable component.
As a first step, it is necessary to define designations for further use in the discussion. The most important output parameters of the ore treatment process are the grade of the valuable component in the concentrate (у1) and in the tailings (у2). The input parameters for this installation include feed volume (V) and mass (Q), solids content, test duration (T), ZrO2 grade in the feed, G-force (G) and valve closing time (t); these input parameters are denoted as xi, where i = 1 ... 7. The only adjustable parameters are G-force, test duration and valve closing time; other input parameters assume random values. All the test results are summarized in Table 1.
To draw correct conclusions about the quantitative influence of a certain input parameter хi on the output yi, the data normalization procedure is carried out. Then, the method of least squares is applied to the obtained data, which allows to determine a linear regression equation, which is optimal in the sense of minimizing errors.
Before directly applying the least squares method to obtain a regression model, the resulting models should be validated in order to choose the most adequate one. To do this, a random experiment needs to be selected from each test to form a validation sample. Then it is required to perform a correlation analysis of the obtained data in order to separate the variables that have the most significant effect on the tailings and concentrate yield.
Table 1
Results of performed tests
Test No.* |
Feed |
Feed |
Solids |
Test |
ZrO2 |
G-force |
Valve closing timeх7 |
ZrO2 |
ZrO2 |
1 |
1.98 |
0.838 |
1 |
33 |
0.2 |
75 |
8 |
0.49 |
0.086 |
1 |
1.872 |
0.887 |
1 |
36 |
0.21 |
75 |
12 |
0.77 |
0.11 |
1 |
2.232 |
0.81 |
1 |
30 |
0.2 |
75 |
24 |
0.72 |
0.14 |
1 |
2.16 |
0.837 |
1 |
30 |
0.24 |
75 |
16 |
0.78 |
0.16 |
1 |
2.88 |
0.992 |
1 |
28 |
0.22 |
90 |
32 |
0.72 |
0.21 |
1 |
2.81 |
0.884 |
1 |
26 |
0.23 |
90 |
48 |
0.88 |
0.13 |
1 |
3.24 |
0.926 |
1 |
24 |
0.21 |
90 |
64 |
1 |
0.11 |
1 |
2.95 |
0.885 |
2 |
25 |
0.21 |
90 |
120 |
1.3 |
0.13 |
1 |
2.81 |
0.843 |
5 |
25 |
0.19 |
90 |
64 |
0.9 |
0.13 |
2 |
2.16 |
0.67 |
1 |
24 |
0.09 |
75 |
10 |
0.12 |
0.05 |
2 |
1.91 |
0.572 |
1 |
25 |
0.085 |
75 |
16 |
0.104 |
0.058 |
2 |
1.62 |
0.395 |
1 |
21 |
0.075 |
75 |
24 |
0.12 |
0.053 |
2 |
2.09 |
0.628 |
1 |
25 |
0.078 |
75 |
32 |
0.13 |
0.048 |
2 |
2.23 |
0.709 |
1 |
26 |
0.091 |
75 |
48 |
0.2 |
0.056 |
2 |
2.05 |
0.53 |
1 |
22 |
0.082 |
75 |
64 |
0.14 |
0.064 |
2 |
1.72 |
0.541 |
6.5 |
26 |
0.095 |
75 |
32 |
0.15 |
0.071 |
3 |
2.88 |
0.309 |
1 |
10 |
0.15 |
90 |
16 |
0.2 |
0.11 |
3 |
2.16 |
0.387 |
1 |
16 |
0.13 |
90 |
24 |
0.19 |
0.1 |
3 |
1.8 |
0.193 |
1 |
10 |
0.14 |
90 |
32 |
0.21 |
0.085 |
3 |
1.8 |
0.193 |
1 |
10 |
0.13 |
90 |
48 |
0.33 |
0.1 |
3 |
2.16 |
0.231 |
1 |
10 |
0.15 |
90 |
64 |
0.35 |
0.11 |
3 |
2.44 |
0.337 |
6.5 |
13 |
0.15 |
90 |
32 |
0.21 |
0.13 |
Note. *Test No.: 1 – test on the middling separation tailings of the coarse-grained stream; 2 – test on the main separation tailings of the coarse-grained stream; 3 – test on the combined tailings of the main and recleaner fine-grained separation.
The algorithm of processing the obtained data will be the following:
- Random selection of one experiment from each test and creation of a validation sample.
- Calculation of paired correlation coefficients between independent variables х1, …х7 and output parameters у1 and у2.
- Selection of independent variables to build a regression model taking into account paired correlation coefficients.
- Development of a regression model from the selected variables.
- Calculation of regression model coefficients using the least squares method.
- Evaluation of the obtained models and selection of the most adequate one.
Evaluation of the obtained models should be guided by the following considerations: the model should describe the initial sample as well as possible, which makes it necessary to use the coefficient of determination R2; it is also necessary that the model demonstrates good results on the validation sample, for which it is required to calculate the sum of squared differences between the predicted y values and the actual ones, taken from the validation sample. It should be taken into account that R2 ∈ [0; 1], so the criterion should include the value of 1/R2. Thus, a criterion for evaluating the resulting regression models is formulated as follows:
where α, β are weight coefficients, α + β = 1; is the value, calculated for the validation sample data based on the model; ya is the actual value of the parameter from the validation sample.
Assignment of different values to a and b coefficients allows to change the quality of the model, either increasing its predictive power or increasing its ability to take into account new incoming data but sacrificing the quality of approximation. In the described case, both a and b coefficients are equal to 0.5.
According to the presented algorithm, which requires the use of determination coefficient R2, in the next step it is necessary to calculate the paired correlation coefficients rxy between independent variables х1, …х7 and output parameters у1 and у2 (Table 2).
Table 2
Paired correlation coefficients
ZrO2 grade |
Feed volume х1 |
Feed mass х2 |
Solids content х3 |
Test duration х4 |
ZrO2 grade |
G-force х6 |
Valve closing time х7 |
In the concentrate, y1 |
0.65 |
0.72 |
– 0.03 |
0.44 |
0.87 |
0.33 |
0.51 |
In the tailings, y2 |
0.57 |
0.40 |
0.08 |
0.12 |
0.84 |
0.51 |
0.15 |
After considering all the models obtained by combining different values of feed volume and mass, ZrO2 grade in the feed and valve closing time, the general formula of this model can be derived as simplified Wiener series:
where n, m, l define the order of the model.
Due to a limited number of experiments and the fact that the number of observations only slightly exceeds the number of parameters, the maximum order of the model should be limited to one. By applying standard methods of regression analysis to such problems [10], a regression equation of the ZrO2 grade in the concentrate was obtained for n = 0; m = 0; l = 0 (linear regression):
In this case, the coefficient of determination R2 for this model equals 0.95, which corresponds to high predictive power of the developed model. As a next step, using formula (1) the value of quality criterion is calculated, for this model I = 0.5493.
According to the presented algorithm for all the possible combinations of variables, the regression equation with the minimal value of the criterion I = 0.5493 is the following:
In this case R2 = 0.968, which indicates high quality of the obtained model. Moreover, the model contains only six terms given 19 observations, which means that the equation actually describes the processes taking place in the system.
The significance of regression coefficients was verified. The probabilities for the coefficients of regression equation (2), estimated with the help of t-statistics, equaled 8.46 ∙10–13; 0.09; 8.50 ∙10–5; 3.96 ∙10–7; 0.0002; 0.02, respectively. Clearly, all the obtained coefficients were statistically significant.
Thus, it was determined that the concentrate yield had practically no dependence on test duration and a weak dependence on G-force. The greatest impact on this parameter was made by ZrO2 grade in the feed, followed by the feed mass and the opening time of the pinch valves. At the same time, it was revealed what influence was exerted by the product of the valuable component grade in the feed and the opening time of the pinch valves. It was also identified that the feed volume V was included in the final equation with a negative coefficient.
Analysis of equation (2) and consideration of the fact that
In the examination of the tailings yield dependence according to the same algorithm used for the concentrate yield and based on the correlation analysis, the following variables are used to build regression models: feed volume x1; ZrO2 grade in the feed x5; G-force x6. Similarly, the models are developed in the form of Wiener series, and it turns out that the minimum value of criterion (1)
I = 0.7242 is obtained with the following equation:
For this model, the coefficient of determination R2 = 0.694, which, according to the Chaddock scale, indicates low predictive power of the model and means that it can only be used to obtain preliminary conclusions and estimations. The probabilities, determined for this equation by calculating t-statistics, show that none of the coefficients correspond to the given level of significance, except for the coefficient before x6. Therefore, it can only be said that an increase in G-force is directly proportional to the tailings yield.
This result for the tailings is in good agreement with other studies that the authors performed on the same installation with another ore material [10]. This allows to assert that in order to build a mathematical model for the tailings, it is necessary to examine additional factors, affecting this parameter.
Conclusion
Semi-industrial tests demonstrate that baddeleyite is effectively processed using the CVD technology (centrifugal separation with continuous variable discharge). Various ABPP tailings were processed, from which the concentrator allowed to efficiently extract the mineral with a 5 % degree of concentration in the primary operation.
Semi-industrial and laboratory studies were carried out under various conditions and on the material with excellent granulometric characteristics; however, output analysis allows to say that in general the results obtained on a semi-industrial installation correlated with laboratory data obtained by the TOMS Institute.
The grade of the valuable component in all streams was different, but the fact that the main recovery occurred from the fine classes, including the –0.044 mm class, remained unchanged, which indicated the efficiency of concentrator operation on liberated grains that are ready for processing.
The concentration of ZrO2 in the fine classes after primary centrifugal separation shows that narrowing the class has a positive effect on increasing the concentration of the valuable mineral and the efficiency of subsequent processing. Narrowing in the class of 0.28 mm allows to increase the concentration by 1.2-1.5 times, and in the class of 0.1 mm – by 2-5 times.
Centrifugal separation is an effective process for primary concentration of the valuable product from the ABPP tailings; however, subsequent recleaning to the concentration values, which are minimally required for the separation of the ABPP finishing, should be carried by other means, most likely using shaking tables.
As a result of the study, authors proposed an algorithm for processing test results, based on classical regression analysis, and formulated an original criterion for model selection. Models of dependencies between the concentrate and tailings yields and the adjustable parameters were built, which allowed to establish a relationship between the concentrate yield and the opening time of the pinch valves, as well as a relationship between the tailings yield and the G-force of the installation.
References
- Fedotov P.K., Petukhov V.I., Fedotov K.V., Burdonov A.E. The Dzhidinsky tungsten-and-molybdenum mining and concentration complex aged dump tailings processing trends analysis. Obogashcheniye rud. 2016. N 1 (361), p. 40-46.
DOI: 10.17580/or.2016.01.07 (in Russian). - Andronov G.P., Perunkova T.N. Production of secondary products from mining-induced ore processing waste from the Kovdor deposit. Vestnik Kolskogo nauchnogo centra RAN. 2018. Vol. 10. N 4, p. 68-73. DOI: 10.25702/KSC.2307-5228.2018.10.4.68-72
(in Russian). - Arkhipov A.V. Determining physical-mechanical properties of complex ores concentration tailings in “Kovdorsky GOK” JSC. Gornyi informatsionno-analiticheskii byulleten. 2014. N 7, p. 83-90 (in Russian).
- Lukichev S.V., Zhirov D.V., Churkin O.E. Mineral reserves and mineral resources of the Murmansk Region: Current conditions and prospects. Gornyi Zhurnal. 2019. N 6, p. 19-24. DOI: 10.17580/gzh.2019.06.01 (in Russian).
- Petrik A.I., Bykhovets A.N., Sokharev V.A. et al. Modernization of mineral raw materials base in the long-term development strategy of kovdor ore dressing and processing enterprise. Gornyi Zhurnal. 2012. N 10, p. 12-18 (in Russian).
- Danilkin A.A., Ivanovsky S.V., Semkin S.V. et al. The main results and realization tasks of the innovation project of large-scale development of the technogenic deposit of the wastes (tails) from concentration production. Gornyi Zhurnal. 2012. N 10,
p. 40-45 (in Russian). - Usikov V.I., Lipina L.N., Aleksandrov A.V., Korneeva S.I. Evaluation of environmental impact of mining waste using GIS technologies. Gornyi informatsionno-analiticheskii byulleten. 2019. N 12, p. 114-126. DOI: 10.25018/0236-1493-2019-12-0-114-126
(in Russian). - Gromov E.V., Opalev A.S., Ivanova V.A., Khokhulya M.S. Efficiency evaluation of technogenic raw materials processing of the Kola mining complex ore deposits. Sever i rynok: formirovanie ekonomiceskogo poryadka. 2018. N 3(59), p. 77-90.
DOI: 10.25702/KSC.2220-802X.3.2018.59.77-90 (in Russian). - Fedotov P.K., Petukhov V.I., Zelinskaya E.V., Burdonov A.E. The Dzhidinsky tungsten-and-molybdenum mining and concentration complex dump tailings processing with a view to produce gold-containing marketable product. Obogashcheniye rud. 2016. N 6 (366), p. 44-50. DOI: 10.17580/or.2016.06.08 (in Russian).
- Pelikh V.V., Salov V.M., Burdonov A.E., Lukyanov N.D. Establishment of technological dependence of KC-CVD6 concentrator operation by means of the argument group accounting method. Bulletin of the Tomsk Polytechnic University. Geo Assets Engineering. 2020. Vol. 331. N 2, p. 139-150. DOI: 10.18799/24131830/2020/2/2500 (in Russian).
- Shadrunova I.V., Gorlova O.E., Galyamov V.Sh., Frolov V.S. Concentration tailings storage using rheology modifiers.
Obogashchenie Rud. 2018. N 2, p. 48-54. DOI: 10.17580/or.2018.02.09 (in Russian). - Fedotov K.V., Tyutyunin V.V. Performance Optimization of Centrifugal Concentrators. Gornyi informatsionno-analiticheskii byulleten. 2013. N 1, p. 208-215.
- Korchak E.A. Sustainable development of the Russian Arctic: The role of labor potential. Proceeding of the International Science and Technology Conference “FarEastСon 2019”, October, 2019, Vladivostok, Russia. Springer, 2020. Vol. 172, p. 713-724. DOI: 10.1007/978-981-15-2244-4_67
- Vinhal J.T., Húngaro R.C., Coleti J.L., Espinosa D.C.R. Iron recovery from zinc mine tailings by magnetic separation followed by carbothermal reduction of self-reducing briquettes. Canadian Journal of Chemical Engineering. 2021. Vol. 99. Iss. 1, p. 166-177. DOI: 10.1002/cjce.23845
- Redwan M.,Bamousa A.O. Characterization and environmental impact assessment of gold mine tailings in arid regions: A case study of Barramiya gold mine area, Eastern Desert, Egypt. Journal of African Earth Sciences. 2019. Vol. 160.
N 103644. DOI: 10.1016/j.jafrearsci.2019.103644 - Gill G., Mittal R.K., Dandautiya R., Purohit N. Sustainable utilization of waste tire-chips reinforced copper tailings as structural fill. Environment, Development and Sustainability. 2020. Vol. 22, p. 4845-4865. DOI: 10.1007/s10668-019-00408-2