An innovative approach to injury prevention in mining companies through human factor management
- 1 — Ph.D. Associate Professor Empress Catherine II Saint Petersburg Mining University ▪ Orcid ▪ Elibrary ▪ Scopus ▪ ResearcherID
- 2 — Ph.D. Associate Professor Empress Catherine II Saint Petersburg Mining University ▪ Orcid ▪ Elibrary ▪ Scopus ▪ ResearcherID
- 3 — Development Director AO SUEK-Kuzbass ▪ Orcid
- 4 — Ph.D. Head of Department Military Institute of Physical Education ▪ Orcid
Abstract
This study argues that human error has an effect on occupational injury risks in mining companies. It shows through an analysis of existing approaches to occupational risk assessment that it is necessary to develop a quantitative assessment method factoring in individual psychophysiological attributes in order to analyze injury risks posed to miners. The article presents the results of a comprehensive analysis of how workers’ psychophysiological attributes influence their susceptibility to occupational injuries in underground mining conditions. By utilizing statistical data processing methods, such as discriminant and regression analysis, the study develops models to forecast personal injury risks among miners. These quantitative models underlie the proposed method for assessing miners’ susceptibility to injuries. The study outlines an algorithm for the practical application of this method and shows how the method was validated using a training sample. It provides recommendations for managing the human factor, incorporating the results of the proposed method, and emphasizes the importance of implementing a series of protective measures to mitigate the risk of occupational injuries in underground mining operations.
Introduction
The mining industry stands out as one of the most hazardous sectors in terms of occupational risks. Data from the Social Insurance Fund and the State Labor Inspectorate in Russia reveal that this sector accounts for 9 % of all fatal accidents, with 55 % of workplaces being hazardous and unsafe. The problem of high injury rates in today’s mining companies is exacerbated by the fact that in the course of their modernization, businesses introduce more productive and technically intricate equipment that is likely to cause injuries. This puts strain on miners, resulting in an increase in both the severity of work-related injuries and the duration of the recovery period. In such a context, managing the human factor has become increasingly crucial, which is evidenced by the growing proportion of accidents caused directly by the victims themselves. According to various estimates [1, 2], between 76 and 95 % of accidents in the mineral resources sector occur primarily due to mistakes at work. This trend is explained by the fact that as technical systems advance, the level of dangers associated with process operations grows faster than the rate at which an individual’s psychophysiological capabilities can adapt to changes [3]. The individual has become a limiting factor in the fight against accidents, and taking measures to minimize equipment failures without simultaneously reducing the human factor seems to be ineffective [4, 5].
The variability in personal qualities among miners results in disparities in abilities, propensity for risk-taking, and susceptibility to work-related injuries. Differences manifest themselves not only among individuals in similar conditions but also within the same individual at different periods of time. Previous studies [6] have demonstrated that accidents within work groups are unevenly distributed among workers: a minority of workers account for the majority of injuries. This implies that a miner’s individual qualities determine his susceptibility to accidents or, conversely, his protection against them. As it is difficult to modify a worker’s psychophysiological attributes, it is prudent to refrain from assigning hazardous work to individuals whose qualities do not inherently provide sufficient protection against industrial injuries. Thus, early assessment of candidates for miner positions regarding their susceptibility to injuries can enhance the efficiency of the employee selection process [6, 7]. Such an assessment enables a more nuanced approach to human factor management, pinpointing specific qualities in miners that require improvement to enhance their protection against work-related injuries [8, 9].
The development of a risk-based approach has yielded several tools for assessing injury risks that factor in individual psychophysiological attributes, including methods such as CREAM [10], HEART [11], SHERPA [12], FLIM [13], SPAR-H [14], TRACer [15], and HFACS [16]. However, due to information uncertainty, these methods are often limited to qualitative and semi-quantitative applications, resulting in subjective analysis outcomes and diminished forecast quality. Algorithms for quantitatively assessing injury risk that factor in workers’ personal qualities and are based on regression models, as exemplified in studies [17-20], facilitate objective forecasting with minimal assumptions when screening a set of the most significant individual qualities. Yet, the existing methods do not account for the specific working conditions in companies engaged in underground mining. This underscores the need to develop a method considering the influence of the most significant psychophysiological attributes of individuals on their susceptibility to injuries in the context of underground mining.
Methods
To develop a method suitable for assessing the susceptibility to injury across various specialties among miners, the study was conducted in conditions that simulated the influence of hazardous and harmful production factors specific to different workplaces underground. These factors included the operation of dangerous machinery and tools, high levels of physical and sensory stress, working in confined spaces and uncomfortable working postures over extended periods, insufficient lighting, and high noise levels. Work scenarios, including abnormal and extreme conditions, were replicated using a training center for junior specialists, a facility enabling the simulation of intense exposure to a combination of hazardous and harmful production factors.
To ensure the statistical reliability and representativeness of the findings, a sample was created of 250 men aged 18 to 20 who were undergoing simulator training at the center. To account for individuals’ adaptive abilities and eliminate the influence of both prior adaptation to the conditions simulated and the experience acquired, the sample consisted exclusively of individuals who had not previously undergone simulator training at the center. Participants were selected based on criteria such as successful completion of medical screening, absence of chronic diseases, and the required level of physiological and psychological development.
It is well-established that the likelihood of human error hinges on a number of an individual’s mental, psychological, and physical qualities, which determine their ability to perform work functions safely, inclination towards risk, and susceptibility to work-related injuries. These qualities include:
- mental attributes such as individual thinking and memory features, stability, and concentration [21], reaction time to stimuli [22], and adaptability to changes in psychomotor, perceptual, affective, and attention factors [23];
- psychological attributes such as anxiety [24, 25], emotional balance [26, 27], self-confidence, self-control, responsibility, sociability, and teamwork ability [21];
- physical attributes, which include height, weight, body proportions, physical strength, endurance [17, 28], sensory abilities, sensory processing sensitivity, muscle reaction time, and movement coordination and accuracy [29].
To incorporate this array of individual qualities, the authors identified a set of primary individual indicators for the study participants (Table 1). Since the key objective of the study was to analyze the fundamental psychophysiological attributes of individuals, the observation process did not account for secondary professional attributes, such as qualifications level, acquired experience, motivation for safe work, motor stereotypes, etc.
Table 1
List of workers’ individual attributes under study
Indicator |
Designation |
Measurement method/tool |
Resting heart rate, bpm |
X1 |
Heart rate monitoring before exercise |
Stange’s test, s |
X2 |
Inspiration breath-hold time |
Hench’s test, s |
X3 |
Exhalation breath-hold time |
Number of push-ups |
X4 |
Maximum number in 30 s |
Number of bends |
X5 |
|
Number of squats |
X6 |
|
Number of jumps with rotation on the spot |
X7 |
|
Heart rate after exercise, bpm |
X8 |
Heart rate monitoring after exercise |
Tidal volume of the lungs, l |
X9 |
Dry spirometer |
Height, cm |
X10 |
Body height measurement in a standing position |
Systolic blood pressure, mm Hg |
X11 |
Korotkoff’s method |
Diastolic blood pressure, mm Hg |
X12 |
Korotkoff’s method |
Psychological self-assessment, score |
X13 |
Self-assessment questionnaire [30, 31] |
Psychological adaptation, score |
X14 |
Test [32] |
Situational anxiety, score |
X15 |
Spielberg – Khanin scale [30, 33] |
Personal anxiety, score |
X16 |
Spielberg – Khanin scale [30, 33] |
Personal adaptive potential, score |
X17 |
Methods presented in [34] |
Behavioral regulation, score |
X18 |
Methods presented in [34] |
Communicative potential, score |
X19 |
Methods presented in [34] |
Moral (normative) sense, score |
X20 |
Methods presented in [34] |
An essential criterion in selecting indicators for the analysis was their testability using standard methods, without the need for specialized equipment. The chosen indicators allowed us to make conclusions about the following:
- the level of physical development and fitness, functional reserves of the respiratory system, and constitutional features of the workers’ bodies determining their performance and resistance to physical stress;
- the state and functional reserves of the workers’ cardiovascular, central, and autonomic nervous systems, which determine their physical capabilities and the level of neuropsychic stability;
- the workers’ adaptive capacities, which determine their ability to quickly and successfully adapt to changing working conditions.
Over a span of five months, following the initial assessment of the participants’ psychophysiological attributes, the medical service of the training center documented all cases of injury while solving training problems and doing exercises in the training complex simulating confined spaces and exposure to dangerous production factors. Over the period, 26 injuries were recorded and 16 workers got injured, i.e., 6.4 % of the participants in the study suffered injuries, with 2.4 % getting injured once and 4 % getting injured twice. The average value of the individual injury risk observed in the sample was 0.250 yr–1.
To identify the factors that determine workers’ predisposition to industrial injuries and to prove the statistical significance of their relationship with injury rates, a discriminant analysis of variables was performed using STATISTICA 10 software by StatSoft. The following factors influenced the choice of this method: its potential for finding independent variables that make it possible to rank workers by levels of predisposition to industrial injuries; no necessity for the normal distribution of values in samples; the ability to use the dependent variable in the nominal form.
The training sample that was prepared using the results of observations was subjected to a discriminant analysis for two groups of the nominal variable (injury and no injury), and an array of quantitative primary indicators of individual psychophysiological attributes were used as independent variables (Table 1). The discriminant analysis procedure involved step-by-step exclusion of variables making an insignificant contribution to the discrimination of the analyzed groups of the nominal variable. As a criterion for excluding a statistically insignificant variable, the value of the F-criterion was used at a significance level of p < 0.05. As a result, a set of predictors was identified that have a statistically significant effect on the presence or absence of injuries (Table 2). To identify the types of dependencies between the variables indicated in Table 2 and the quantitative indicator of injury rate, the identified relationships were formalized in the course of a univariate regression analysis. As a dependent continuous variable, the indicator of the average annual injury frequency rate (individual risk rate) was used, which is generally accepted in studies associated with risk theory. In order to identify the general trend for plotting functions, the predictor variables i were split into intervals and the mean values for each interval were found. The workers were grouped in accordance with the selected intervals of the variables i, and the average values of the injury frequency rate in each group were found. Consequently, variables best approximated by strictly monotonic functions with an approximation confidence level of R2 > 0.80 (Fig.1) were identified. This made it possible to include in the final models for the quantitative assessment of injury risks only those factors whose influence on injury rates could be unequivocally justified.
Table 2
List of psychophysiological indicators that have a statistically significant relationship with the injury rate
Indicator |
Designation |
F-criterion |
p-value |
Number of push-ups |
X4 |
5.11 |
0.025 |
Resting heart rate, bpm |
X1 |
3.88 |
0.049 |
Situational anxiety, score |
X15 |
4.51 |
0.034 |
Stange’s test, s |
X2 |
5.08 |
0.025 |
Personal adaptive potential, score |
X17 |
4.89 |
0.027 |
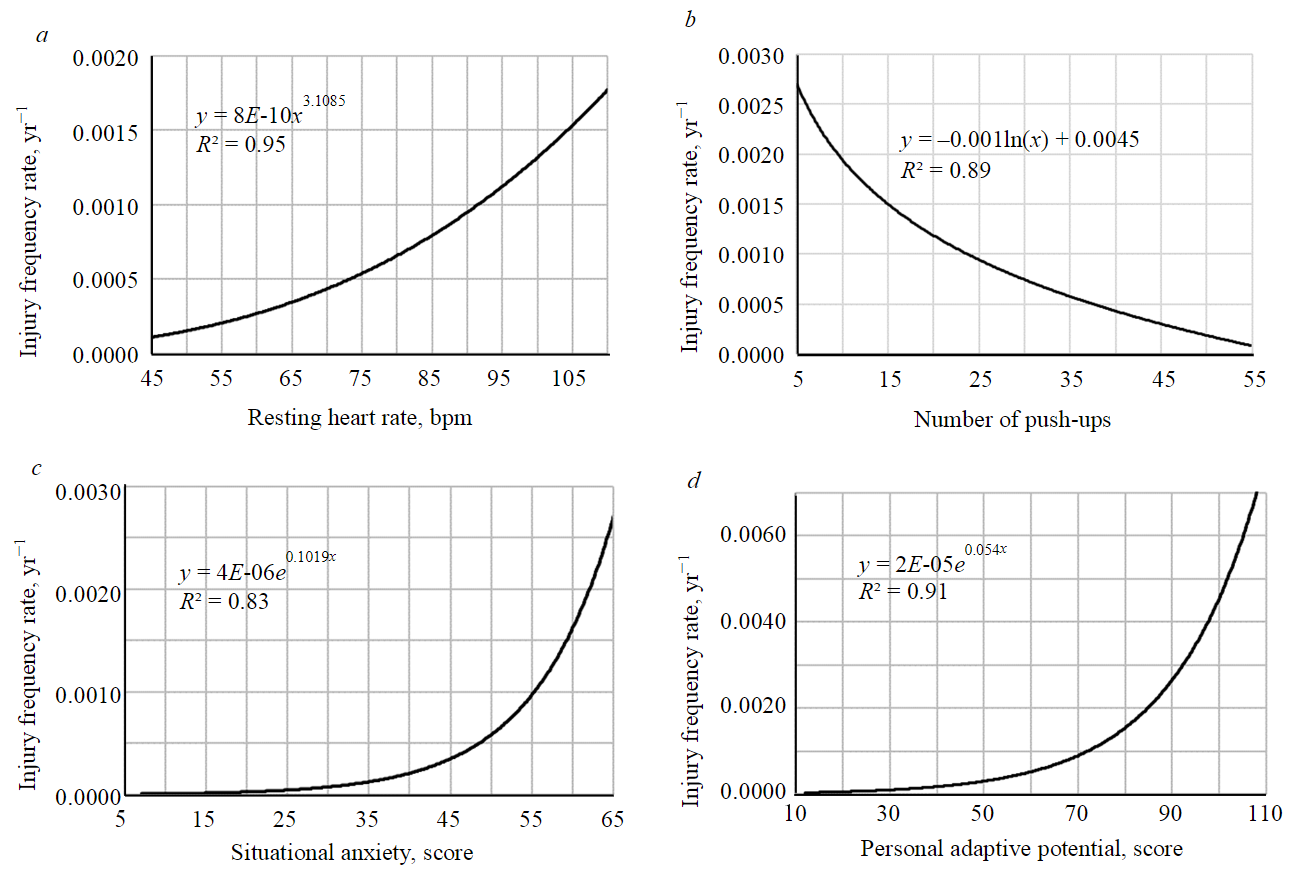
Fig.1. Average injury frequency rate as a function of resting heart rate (a); number of push-ups (b); situational anxiety (c); personal adaptive potential (d)
For the purpose of a comprehensive quantitative assessment of occupational injury risks to workers and their predisposition to injuries, a multivariate regression analysis was performed. This analysis yielded calculation models enabling the determination of the conditional mathematical expectation of the personal injury frequency rate (personal injury risk denoted as RT, yr–1) while considering the functions shown in Fig.1. Due to constraints related to the size of the initial sample and variations in working conditions among miners of different professions [23], two models for calculating the personal risk indicator were developed. For workplaces with unstable working conditions that change under normal operation, it is recommended to assess the miner’s predisposition to injuries taking into account his or her adaptive abilities based on the personal adaptive potential indicator:
For workplaces where working conditions can change only in emergencies and extreme situations, the assessment of the miner’s predisposition to injuries can be performed taking into account his or her neuropsychic stability based on the situational anxiety indicator:
The quality of the equations derived from the multivariate regression analysis was evaluated using a number of standard criteria at a significance level of p = 0.05. As the F-test and the t-statistic show, the selected variables can be included in the model and the regression coefficients are statistically significant. The F-statistic value indicates that the regression models are statistically reliable.
Discussion
In the mining industry, not enough attention is paid to the alignment of miners’ psychophysiological attributes with working conditions, resulting in inadequately prepared personnel who cannot promptly respond to dangerous situations [35]. Utilizing methods to assess occupational risks becomes paramount in confirming an individual’s suitability for work in specific mining conditions, as his or her unique set of qualities may indicate an elevated susceptibility to injuries and unsuitability for a particular type of activity [29, 36].
The identified cluster of individual attributes can be used to gauge an individual’s injury propensity in the distinct context of underground mining. The study shows that those participants who sustained injuries initially exhibited a lower level of overall health, which is manifested in diminished neuropsychic stability, suboptimal regulation of physiological functions, and limited functional reserves. A comparative analysis of psychological assessments revealed that uninjured individuals displayed superior behavioral regulation in novel and challenging test conditions, demonstrating a more advanced adaptability, fitter cardiovascular and autonomic systems, and superior physical fitness.
An increase in the resting heart rate (Fig.1, a) leads to a monotonous increase in the observed injury frequency rate, which is quite logically explained by the predominance of sympathicotonia in individuals with faster heart rates. This predetermines their tendency to feel excessively emotional and stressed, and, consequently, experience fatigue and low motivation along with lower levels of attention, which makes them more prone to industrial injuries. Heart rate can also be viewed as an indicator of physical health, characterizing the body’s resistance to physical fatigue and a decline in performance.
Crucially, the injured workers had a significantly lower level of physical fitness. Figure 1, b demonstrates the inverse relationship between the number of push-ups and the injury frequency rate due to an increase in personal injury risk with a decrease in physical abilities in conditions of high-intensity physical work. On the contrary, workers who were physically fit showed a lower tendency to injuries, which may be due to their high physical strength and endurance, well-developed motor abilities and muscle coordination, and higher body resistance to a number of dangerous external influences.
Figure 1, c shows the influence of situational anxiety, which indicates that workers sustaining injuries tend to experience stress when responding to emergencies and extreme situations. The function shown in Fig.1, d demonstrates the difference between the groups of injured and non-injured workers in their levels of personal adaptive potential. The integral indicator of personal adaptive potential X17 is directly dependent on the indicators of behavioral regulation X18, communicative potential X19, and moral (normative) sense X20. High values of these indicators signal a tendency to nervous breakdowns and inadequate self-esteem, impaired communication skills, and inadequate perception of reality. Such workers are characterized by a low level of socialization, cannot assess their place and role in the team, and experience difficulties in performing group tasks. These accentuated character traits identified among the injured participants suggest that it is challenging for them to adapt to new working conditions.
The analysis of the functions shown in Fig.1 is of practical interest from the point of view of identifying risk groups based on workers’ predisposition to injuries. The ranges where the rate of change grows significantly make it possible to say that workers demonstrating the following characteristics belong to the group most at risk in terms of suffering injuries: a resting heart rate of more than 80 bpm, the number of push-ups in 30 s lower than 15, the situational anxiety indicator higher than 50, and the personal adaptive potential indicator higher than 80.
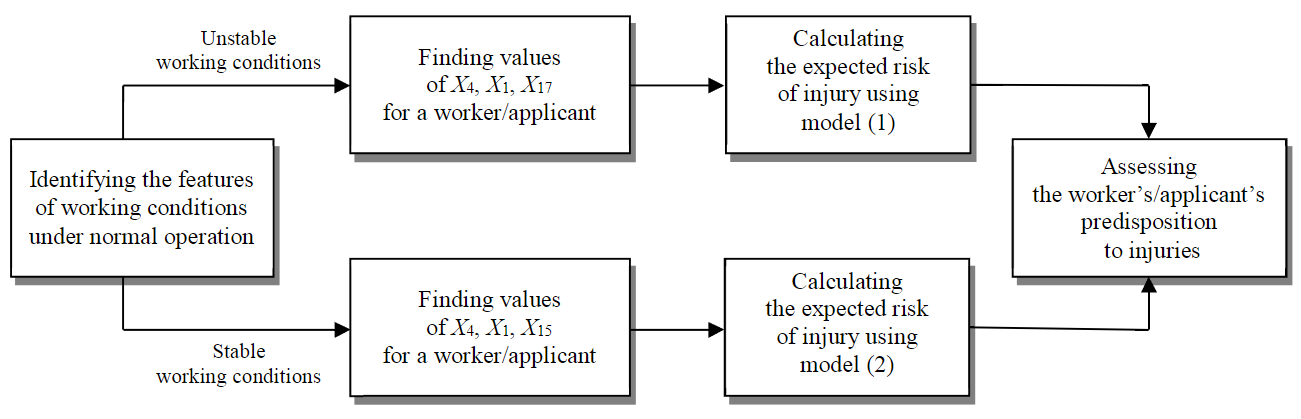
Fig.2. Algorithm for categorizing workers based on their predisposition to injuries
Joint consideration of the above factors is possible when using models (1) or (2). However, it is worth considering the scope of these models. The calculated coefficients of determination for model (1) R21 = 0.12 and model (2) R22 = 0.10 indicate that the factors included in the model determine 12 and 10 % of variations in the observed injury frequency rate, respectively. This is due to the complex and multifactorial nature of industrial injuries that cannot be accurately predicted solely based on workers’ individual attributes. Therefore, models (1) and (2) cannot be used for an accurate quantitative forecast of the likelihood of injuries in the mining sector. For this purpose, it is necessary to create complex probabilistic and statistical models to ensure a comprehensive and multifactorial risk assessment. However, given that the likelihood of an industrial injury is influenced by many factors, the variation shares of 12 and 10 % explained by the models seem to be quite significant. The study identified at a statistically significant level a number of workers’ individual attributes that largely predetermine their predisposition to occupational injuries in underground mining. The scope of application of models (1) and (2) is limited to the purposes of a priori determination of miners’ predisposition to industrial injuries taking into account their individual psychophysiological characteristics on the basis of a quantitative indicator of the expected risk of injury. The indicated scope of application fits the objective of identifying risk groups by injury predisposition during job selection procedures and in managing the human factor.
To facilitate the practical application of models (1) and (2), the authors present a method for classifying miners or candidates applying for mining positions into risk groups based on their susceptibility to injuries (Fig.2). The process begins by defining the specific features of the working conditions at the miner’s workplace under normal operating circumstances. Subsequently, an evaluation algorithm is developed as follows:
- if the conditions at the workplace are unstable under normal operation, the subsequent step involves measuring the resting heart rate X1, the number of push-ups X4, and the personal adaptive potential indicator X17 (see Table 1). This group of working conditions includes workplaces that exhibit regular changes in the production process and the production environment parameters or frequent changes in the composition of work teams, workplaces of mine rescue team members, etc. In this case, the personal injury risk indicator is calculated using model (1);
- if the workplace maintains relatively stable conditions under normal operation, with potential changes only in an emergency or extreme situation, the subsequent step involves measuring the resting heart rate X1, the number of push-ups X4, and the situational anxiety indicator X15 (Table 1). Workplaces that belong to this group include those characterized by the stability of working conditions and the possibility of emergencies, accidents, disruptions to the production process, etc. In the next step, the personal injury risk indicator is calculated using model (2).
To assign a miner or job applicant to a particular injury risk group, the quantitative indicator of the expected injury risk is assessed using the threshold risk values proposed in Table 3. As the quantitative value of the permissible risk of injury in the mining industry has not yet been established, the average value of the permissible risk of fatal injury established for the Russian coal industry can be used, which is 2·10–4 yr–1 [37].
Table 3
Risk groups indicating miners’ predisposition to injuries
Risk group |
Threshold values, yr–1 |
Description |
Low risk level (acceptable risk) |
RT1, RT2 < 1·10–3 |
The miner/applicant has a minimal predisposition to industrial injuries; the person’s injury risk is relatively safe from the influence of his/her individual attributes |
Moderate risk level (acceptable risk) |
1·10–3 ≤ RT1, RT2 < 2·10–3 |
The miner/applicant has a moderate predisposition to industrial injuries; the person’s individual attributes may influence his/her injury risk |
High risk level (unacceptable risk) |
RT1, RT2 ≥ 2·10–3 |
The miner/applicant has a high predisposition to industrial injuries; the person’s individual attributes make him/her susceptible to danger and industrial injuries |
Considering damage to health, the maximum permissible level of injury risk without fatality is determined to be ten times the specified value at RT(acc) = 2·10-3 yr–1. The permissible risk range is proposed to be divided into two equal zones separated by a boundary (RT(thr) = 0,5RT(acc) = 1·10–3 yr–1), with the upper zone meaning that the person’s individual attributes make them predisposed to injuries and the lower zone meaning that they do not. To validate the proposed method utilizing primary data from study participants not previously included in the training sample, the expected injury frequency rates were calculated, and injury risk groups were identified. Due to the mixed nature of the simulated working conditions, testing was conducted using both model (1) and model (2). A comparison of the results with the observed injury rates revealed the following:
- model (1): among the injured participants, 56 % were classified as high risk, 25 % as moderate risk, and 19 % as low risk. The overall distribution of participants into high-risk, medium-risk, and low-risk categories was 17.2 %, 52 %, and 30.8 %, respectively;
- model (2): among the injured participants, 50 % were classified as high risk, 38 % as moderate risk, and 12 % as low risk. The overall distribution of participants into high-risk, medium-risk, and low-risk categories was 19.6 %, 48 %, and 32.4 %, respectively.
The sensitivity of models (1) and (2) to identifying workers with a high risk of injury is estimated at 69 and 68 %, respectively. The distribution of workers by risk groups in both the sample of injured workers and the total sample shows that when using models (1) and (2), the a priori probability of classifying an injured worker as belonging to the group with the high level of risk is 3.3 and 2.5 times higher than for a non-injured worker. The opposite situation is observed when the injured workers are classified as belonging to the categories of acceptable risk: when using models (1) and (2), the a priori probability of their being classified as belonging to the group with the moderate level of risk is 2.1 and 1.3 times lower than that for non-injured workers and also 1.6 and 2.7 times lower for the low level of risk. This indicates the method’s sensitivity in identifying individuals prone to occupational injuries, confirming its suitability for professional selection and human factor management purposes.
Individuals classified as high or moderate risk for injury require priority human factors management measures. Before they are admitted to work, miners falling into these groups must complete a specialized professional training program emphasizing safe work practices. Special attention should be paid to solving issues regarding workers’ adaptation to new working conditions, reducing anxiety, and enhancing emotional stability [38, 39]. Effective methods in this direction involve the use of specialized training programs utilizing virtual and augmented reality technologies [40]. Virtual simulators, in particular, hold significant potential, allowing the simulation and practice of various hazardous situations, thereby reducing the likelihood of inappropriate and dangerous behavior in miners in extreme conditions.
Certainly, miners’ psychophysiological attributes must align with the specific working conditions. Reciprocally, providing miners with acceptable working conditions that account for individual characteristics reduces stress levels, positively influences motivation, and minimizes the risk of occupational injuries [41, 42].
Conclusion
The safeguarding of miners against occupational accidents depends to a great extent on their individual qualities and cannot be solely guaranteed through organizational and technical measures. Hence, it is crucial to have an effective system of employee selection and training procedures, which necessitates the use of methodological approaches aimed at identifying workers susceptible to occupational injuries.
The application of the method proposed by the authors allows for the proactive identification of miners prone to occupational injuries. This offers vital insights for human factor management procedures in mining companies. Taking into account miners’ key psychophysiological attributes offers a fresh perspective on the issue of injuries, enabling the formulation of crucial strategies to protect workers from accidents. In combating occupational injuries, preventive measures targeting human errors should be all-encompassing, not only focusing on managing the human factor but also ensuring the maintenance of a working environment.
References
- Zhaobo Chen, Gangzhu Qiao, Jianchao Zeng. Study on the Relationship between Worker States and Unsafe Behaviours in Coal Mine Accidents Based on a Bayesian Networks Model. Sustainability.2019. Vol. 11. N 5021. DOI: 10.3390/su11185021
- Khomenko A.O., Chekmareva M.A., Zabolotskikh T.V. et al. Specificity of psychological and socio-psychological risk-oriented approaches to labor protection management. Russian Journal of Labor Economics. 2019. Vol. 6. N 2, p. 901-912 (in Russian). DOI: 10.18334/et.6.2.40693
- 3. Litvinenko V.S. Digital Economy as a Factor in the Technological Development of the Mineral Sector. Natural Resources Research. 2020. Vol. 29. N 3, p. 1521-1541. DOI: 10.1007/s11053-019-09568-4
- Glebova E.V., Volokhina A.T., Vikhrov A.E. Assessment of the efficiency of occupational safety culture management in fuel and energy companies. Journal of Mining Institute. 2023. Vol. 259, p. 68-78. DOI: 10.31897/PMI.2023.12
- Yurak V.V., Dushin A.V., Mochalova L.A. Vs sustainable development: scenarios for the future. Journal of Mining Institute. 2020. Vol. 242, p. 242-247. DOI: 10.31897/PMI.2020.2.242
- Palyanitsina A.N., Akhmedova A.N. Implementing the case study method in a process of teaching oil engineers. Journal of Physics: Conference Series. 2020. Vol. 1515. N 022049. DOI: 10.1088/1742-6596/1515/2/022049
- Tskhadaya N.D., Zakharov D.Yu. Improvement of the Procedure of Recruitment of Personnel for Hazardous Work Environment. Journal of Mining Institute. 2018. Vol. 230, p. 204-208. DOI: 10.25515/PMI.2018.2.204
- Reitynbarg D.I. Some psychological issues of industrial injuries prevention theory. Istoriya rossiiskoi psikhologii v litsakh: Daidzhest. 2018. N 2, p. 103-149 (in Russian).
- Pakholkova N.V., Vakhnina E.G., Zaitsev A.V. Relevance of personality development of students in modern conditions. Theory and Practice of Physical Culture.2020. N 4, p. 40-41 (in Russian).
- Wenyu Wang, Zhengqing Dai, Jiahao Rao, Meng Tian. Human Operating Risk Assessment for Outdoor Terminal Box of Electric Power. Wireless Personal Communications. 2022. Vol. 126. Iss. 3, p. 2303-2319. DOI: 10.1007/s11277-021-09067-x
- Williams J.C. HEART – a proposed method for achieving high reliability in process operation by means of human factors engineering technology. Safety and Reliability. 2015. Vol. 35. Iss. 3, p. 5-25. DOI: 10.1080/09617353.2015.11691046
- Torres Y., Nadeau S., Landau K. Classification and Quantification of Human Error in Manufacturing: A Case Study in Complex Manual Assembly. Applied Sciences. 2021. Vol. 11. Iss. 2. N 749. DOI: 10.3390/app11020749
- Hu-Chen Liu, Jing-Hui Wang, Ling Zhang, Qi-Zhen Zhang. New success likelihood index model for large group human reliability analysis considering noncooperative behaviors and social network. Reliability Engineering and System Safety. 2022. Vol. 228. N 108817. DOI: 10.1016/j.ress.2022.108817
- Wenjun Zhang, Xiangkun Meng, Xue Yang et al.A Practical Risk-Based Model for Early Warning of Seafarer Errors Using Integrated Bayesian Network and SPAR-H. International Journal of Environmental Research and Public Health. 2022. Vol. 19. Iss. 16. N 10271. DOI: 10.3390/ijerph191610271
- Dehghani T., Mahdavi S., Fardosrad N. et al.EvaluatingHuman Errors using HEART and TRACEr Methods: Case Study at a Petrochemical Plant. International Journal of Occupational Hygiene. 2019. Vol. 11. N 4, p. 247-258.
- Li Yang, Xue Wang, Junqi Zhu et al. Comprehensive Evaluation of Deep Coal Miners’ Unsafe Behavior Based on HFACS-CM-SEM-SD. International Journal of Environmental Research and Public Health. 2022. Vol. 19. Iss. 17. N 10762. DOI: 10.3390/ijerph191710762
- Park S.J., Jung M., Sung J.H. Influence of Physical and Musculoskeletal Factors on Occupational Injuries and Accidents in Korean Workers Based on Gender and Company Size. International Journal of Environmental Research and Public Health. 2019. Vol. 16. N 345. DOI: 10.3390/ijerph16030345
- López-Garcia J.R., Garcia-Herrero S., Gutierrez J.M., Mariscal M.A. Psychosocial and Ergonomic Conditions at Work: Influence on the Probability of a Workplace Accident. BioMed Research International. 2019. Vol. 2019. N 2519020, p. 1-13. DOI: 10.1155/2019/2519020
- Frolova E.A. Factors influencing forecasting of individual injuries. South-Siberian Scientific Bulletin. 2020. N 2 (30), p. 52-56 (in Russian). DOI: 10.25699/SSSB.2020.30.62670
- Ivanova M.V., Glebova E.V., Retinskaya I.V. Assessment of professional suitability of LLC “Gazprom transgaz Samara” specialists applying the method of regression analysis. Environmental Protection in Oil and Gas Complex. 2018. N 5, p. 20-22 (in Russian). DOI: 10.30713/2411-7013-2018-5-20-22
- Mamayev V.V., Rozanova Ye.N., Dikenshteyn I.F. The role of the human factor in the injury rate of firemen. Scientific Bulletin of the NIIGD “Respirator”. 2017. N 4 (54), p. 82-91 (in Russian).
- Shangareyev R.R. Employees motivation – the basic mechanism professional risks on production management systems. Oil and Gas Business. 2018. N 3, p. 180-198 (in Russian).
- 23. Gendler S.G., Tumanov M.V., Levin L.Yu. Principles for selecting, training and maintaining skills for safe work of personnel for mining industry enterprises. Naukovyi Visnyk Natsionalnoho Hirnychoho Universytetu. 2021. N 2, p. 156-162. DOI: 10.33271/nvngu/2021-2/156
- Skopintseva O.V., Balovtsev S.V. Air quality control in coal mines based on gas monitoring statistics. Mining Informational and Analytical Bulletin. 2021. N 1, p. 78-89 (in Russian). DOI: 10.25018/0236-1493-2021-1-0-78-89
- Shabanov N.I., Lipkovich I.E., Petrenko N.V et al. Ergonomics and psychophysiological foundations of occupational safety in agricultural engineering: A monograph. Zernograd: AChII FGBOU VO Donskoi GAU v g. Zernograde, 2018, p. 265 (in Russian).
- Zinovieva O.M., Merkulova A.M., Smirnova N.A., Shcherbakova E.A. Psychosocial risk management in mining. Mining Informational and Analytical Bulletin. 2022. N 1, p. 20-33 (in Russian). DOI: 10.25018/0236_1493_2022_1_0_20
- Elmurzaev M.A., Panchenko I.A., Pakholkova N.V. Socio-psychological health / physical recreation model. Theory and Practice of Physical Culture. 2019. N 6, p. 44-46 (in Russian).
- Dubrovskaya Yu.A., Pikhkonen L.V., Dubrovskaya B.A. Professional competence formation in experience-oriented physical training and sports activities of mining engineers. Mining Informational and Analytical Bulletin. 2021. N 2, p. 127-138 (in Russian). DOI: 10.25018/0236-1493-2021-2-0-127-138
- Kang D. Workability and Life Satisfaction: Effects of Workers’ Positive Perceptions on Their Return to Jobs. Safety and Health at Work. 2022. Vol. 13. Iss. 3, p. 286-293. DOI: 10.1016/j.shaw.2022.05.002
- Ivanov E.A., Kalik V.V. On the need to study the physical qualities and personal characteristics of operators of unmanned aerial vehicles. Uchenye zapiski universiteta im. P.F.Lesgafta. 2023. N 1 (215), p. 193-196 (in Russian). DOI: 10.34835/issn.2308-1961.2023.01.p193-1
- Novikov V.S., Bochenkov A.A., Chermyanin S.V. A psychophysiological rationale of the problem of correction and rehabilitation of military personnel engaged in combat. Voenno-meditsinskii zhurnal. 1997. Vol. 318. N 3, p. 53-57 (in Russian).
- Belov V.V., Korzunin A.V., Yusupov V.V., Kostin D.V. The use of neurous and mental stability assesement techniques of servicemen. Vestnik LGU im. A.S.Pushkina. 2014. N 3, p. 75-88 (in Russian).
- Chutko L.S., Rozhkova A.V., Surushkina S.Yu. et al. Clinical manifestations of burnout. Zhurnal Nevrologii i Psikhiatrii imeni S.S. Korsakova. 2019. Vol. 119. N 1, p. 14-16 (in Russian). DOI: 10.17116/jnevro201911901114
- Maklakov A.G. Fundamentals of psychological support for the occupational health of military personnel: A dissertation abstract. St. Petersburg: VMA im. S.M.Kirova, 1996, p. 37 (in Russian).
- Koteleva N., Kuznetsov V., Vasilyeva N. A Simulator for Educating the Digital Technologies Skills in Industry. Part One. Dynamic Simulation of Technological Processes. Applied Sciences. 2021. Vol. 11. Iss. 22. N 10885. DOI: 10.3390/app112210885
- Nikolaev A.B. Prediction of phenomenon of human factor and correction of its negative effect. Bezopasnost truda v promyshlennosti. 2017. N 9, p. 52-57 (in Russian). DOI: 10.24000/0409-2961-2017-9-52-57
- Kabanov E.I. Allowable occupational injury risk assessment in coal mining industry. Mining Informational and Analytical Bulletin. 2022. N 5, p. 167-180 (in Russian). DOI: 10.25018/0236_1493_2022_5_0_167
- Lvov V.V., Smirnova Z.V., Artemova E.I. et al. State of University-Employer Interaction Models in Russia. Journal of Entrepreneurship Education. 2019. Vol. 22. Iss. 4, p. 1-9.
- Kretschmann J., Plien M., Thi Hoai Nga Nguyen, Rudakov M. Effective capacity building by empowerment teaching in the field of occupational safety and health management in mining. Journal of Mining Institute. 2020. Vol. 242, p. 248-256. DOI: 10.31897/PMI.2020.2.248
- Gubin V.V., Fedorova E.R., Darin A.A. Methods of training simulators development in aspect of increasing efficiency and safety production. International Journal of Management. 2019. Vol. 10. Iss. 2, p. 117-121. DOI: 10.34218/IJM.10.2.2019.010
- Barkhordari A., Malmir B., Malakoutikhah M. An Analysis of Individual and Social Factors Affecting Occupational Accidents. Safety and Health at Work. 2019. Vol. 10. Iss. 2, p. 205-212. DOI: 10.1016/j.shaw.2019.01.002
- Gendler S., Prokhorova E. Risk-Based Methodology for Determining Priority Directions for Improving Occupational Safety in the Mining Industry of the Arctic Zone. Resources. 2021. Vol. 10. Iss. 3. N 20. DOI: 10.3390/resources10030020