Use of machine learning technology to model the distribution of lithotypes in the Permo-Carboniferous oil deposit of the Usinskoye field
Abstract
Permo-Carboniferous oil deposit of the Usinskoye field is characterized by an extremely complex type of the void space with intense cross-sectional distribution of cavernous and fractured rock. In this study, for this production site, the process of 3D geological modeling has been implemented. At the first stage, it provided for automated identification of reservoir volumes by comparing the data of core and well logging surveys; at the second stage, identification of rock lithotypes according to Dunham classification is performed on the basis of comparison of thin sections examination and well logging data. A large array of factual information enables the use of machine learning technology on the basis of Levenberg – Marquardt neural network apparatus toward achievement of our research goals. The prediction algorithms of reservoir and rock lithotype identification using well logging methods obtained on the basis of the training samples are applied to the wells without core sampling. The implemented approach enabled complementing the 3D geological model with information about rock permeability and porosity, taking into account the structural features of the identified lithotypes. For the Permo-Carboniferous oil deposit of the Usinskoye field, the volumetric zoning of the distribution of different rock lithotypes has been established. Taking into account the lithotypes identified based on machine learning algorithms, density and openness of fractures were determined, and fracture permeability in the deposit volume was calculated. In general, during the implementation, the machine learning errors remained within 3-5 %, which suggests reliability of the obtained predictive solutions. The results of the research are incorporated in the existing 3D digital geological and process model of the deposit under study.
Introduction
The main trend of recent times for the Russian oil and gas sector is the shift of hydrocarbon (HC) production to areas with a complex geological structure. This process is objective, since active oil reserves have long been in development and are operated by a high-water-cut well stock. The vast majority of Russian oil fields with active reserves that have not yet been put into development are classified as small or very small. In modern conditions, relatively large increments in hydrocarbon reserves can be obtained only by increasing the development efficiency of fields with hard-to-recover reserves. The Timan-Pechora oil and gas province (OGP) is an example of a territory with high prospects of a potential increase in hydrocarbon production [1]. The Timan-Pechora OGP is characterized by an extremely complex geological structure, high heterogeneity of productive strata, and division of deposits into separate blocks by a system of fractures [2]. For this territory, oil production is achieved through extensive use of enhanced oil recovery technologies to enable the development of extra-heavy oil [3, 4].
Morphologically, organogenic structures of the Timan-Pechora OGP belong to the category of hills, the difference from the classical reefs being the absence of typical massive framework organisms, rear and front plumes [5]. The geological cross-section of Permo-Carboniferous sediments has a cyclic structure manifested in a regular change of lithotypes of carbonate rock. As a rule, the rock structure includes voids of different origins, which is explained by a long post-sedimentation history of rock exposure to multidirectional influences (changes in the relative sea level, etc.).
During sediment genesis in the Permo-Carboniferous complex, morphologically pronounced organogenic structures were formed consisting of various species of crinoids and bryozoans, whose skeletons underwent destruction due to a steady drift and active hydrodynamics of the environment. During diagenesis, the primary structure of the void space was mainly altered by cementation and leaching. Whereas the development of cementation led mainly to the healing of voids, the leaching processes, on the contrary, increased the void space. Dolomitization of rock in the Permo-Carbonife-rous complex is generally insignificant, which is probably due to a good communication of the sedimentation basins with the waters of the World Ocean [6]. During the stage of catagenesis, the processes of cementation and leaching had also been quite intense. In addition, fracturing occurred predominantly within the development zones of dense lithotypes, resulting in the ability of local voids in reservoir rock to connect into a single filtration system.
Thus, the facies heterogeneity of Permo-Carboniferous sedimentary rock is reflected primarily in the structure of the void space and the progressive processes of leaching [7, 8]. Researchers have proposed principles for rock lithotyping based on grain size, the amount of silty material, and se-condary fracturing. The classification system proposed by R.Dunham is most commonly used in practice [9], according to which the main role in the formation of carbonate sediments is played by two main types: boundstones, in which the original components are bound together during sedimentation, and bioclastic limestones, consisting of fragments of organisms and micritic material (pack-, wacke- and mudstones) [7].
One of the most significant production sites of the Timan-Pechora OGP in terms of the volume of reserves is the Permo-Carboniferous deposit of the Usinskoye field. Tectonically it is located within the northern part of the Usinsky swell in the junction zone of the Kolvinsky megaswell and the Synyanyrdskaya depression (Fig.1). The Permian sediments of the deposit are represented by bryozoic-crynoic limestones, the Upper Carboniferous sediments – by detrital and organogenic fragmental, occasionally brecciform varieties. In the Middle Carboniferous sediments, detrital and organogenic fragmental limestones prevail in the upper part. The lower part is composed of algal and organogenic fragmental, occasionally dolomitized limestones with thin interlayers of argillite-like clays.
In the entire geological cross-section of the Permo-Carboniferous sequence, porous and cavernous intervals occur. With an oil-saturated layer of more than 350 m, this site is characterized by abnormally high oil viscosity within the range of 340 to 2000 mPa·s, which predetermines the use of thermal methods of oil production. The deposit is being geologically studied for almost 50 years, which explains a large number of dedicated scientific papers. According to the data of core petrophysical studies, formation of reservoir void space is mainly associated with leaching processes, which predetermined a complex type of the void space with intensive distribution of fracturing across the carbonate section [10, 11]. In some cases, even in dense varieties, the rock is saturated with oil or bituminous organic matter; negative impact of bituminization on well performance is exacerbated to an even greater extent in rocks with reduced permeability and porosity [12, 13]. In the meantime, there are well-performing intervals with low matrix porosity, which suggests the impact of fracturing on oil production [14]. Сavernous processes manifest in frequently occurring incomplete core recovery. In general, the Permo-Carboniferous sequence of the Usinskoye field can be classified as fracture-cavernous-porous type, which can justify treating it as a single reservoir.
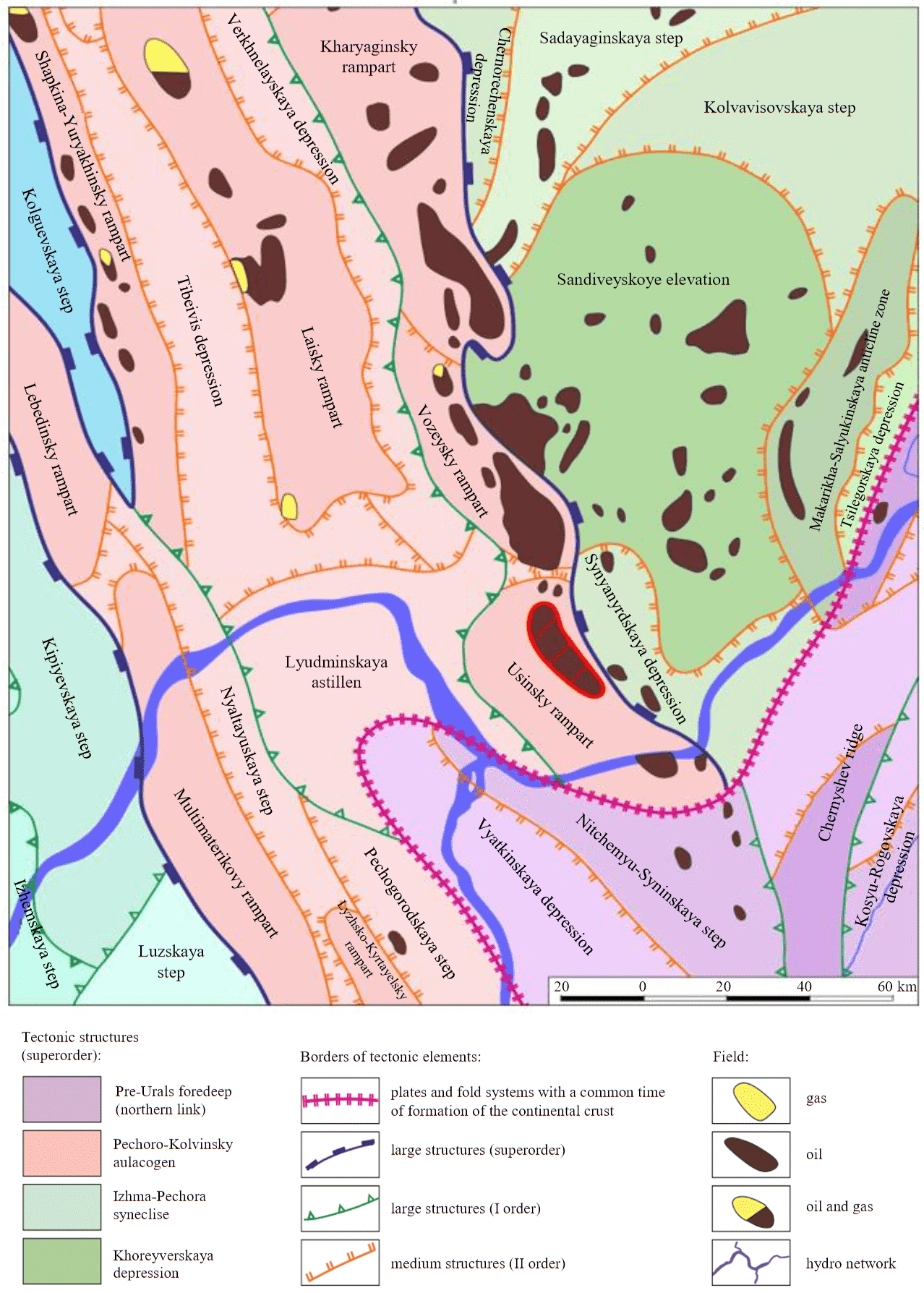
Fig.1. Extract from the map of tectonic zoning of the Timan-Pechora sedimentary basin
Problem definition
The lithofacial geological model is primarily based on well core studies. For the deposit under study, the scope of this type of work was carried out in atmospheric conditions on 7855 standard samples (d = 30 mm) oriented parallel to the strata. For potentially fractured-cavernous sediments, petrophysical studies should include full-size samples, which enables a comprehensive study of both microcracks and large-sized caverns [15]. The study also included 1416 full-size core samples (d = 64 and 110 mm). As a result, it was established that the reservoirs are represented by limestones, dolomitized limestones and their transitional varieties. The fractures are predominantly vertical, open type or fine-grain calcite, fracture openness is within 5 to 600 μm.
Based on the principles of R.Dunham's classification, an analysis of more than 1000 thin sections for the Permo-Carboniferous deposit of the Usinskoye field was carried out in [14, 16], aiming at establishing the relationship between rock lithology and permeability and porosity. Figure 2 presents the thin sections showing the structure of the void space of different lithotypes.
Mud-, wacke-, pack-, grain-, float- and rudstones whose primary components were not bound during their accumulation are represented in different proportions by biogenic and fragmental material. These lithotypes differ by cementation type and grain size. Figure 2, а shows a thin section of this type of rock, which belongs to the impermeable cross-section. A distinctive feature of boundstones is their biomorphic whole-skeleton structure. Figure 2, b shows a thin section for boundstone from cavernous-porous rock interval. A standalone type is dolomitized limestone (crystal carbonate according to Dunham [9]), where the primary structure cannot be recognized.
Core analysis for different lithotypes performed earlier in [14] showed that permeability coefficients (k) for rud-, pack-, grain- and floatstones are within the low range of values below 50 mD, for mudstones – mostly 50 to 200 mD. Boundstones are found to have rock fracturing and wider k variation ranges than other types. Coefficient k values exceeding 1000 mD are found in boundstones and occasionally dolomitized limestones. In the meantime, whereas dolomitized carbonates have porous structure of void space, boundstones belong to the porous-cavernous type. The general conclusion is that the structure of porous space, as well as porosity and permeability, are primarily related to the mineral composition of rock [14].
To identify reservoirs of the porous type, the study uses a standard set of well logging methods, including well diameter measurement, spontaneous potential logging (SPL), gamma-ray logging (GRL), neutron-gamma or neutron-neutron logging (NNL), acoustic logging (AL). Notably, the entire set of the six well logging methods has been performed only for 22 % wells (320 out of 1438). Besides, in 104 wells, special survey has been performed using the method of gamma-gamma density logging (GGL-d).
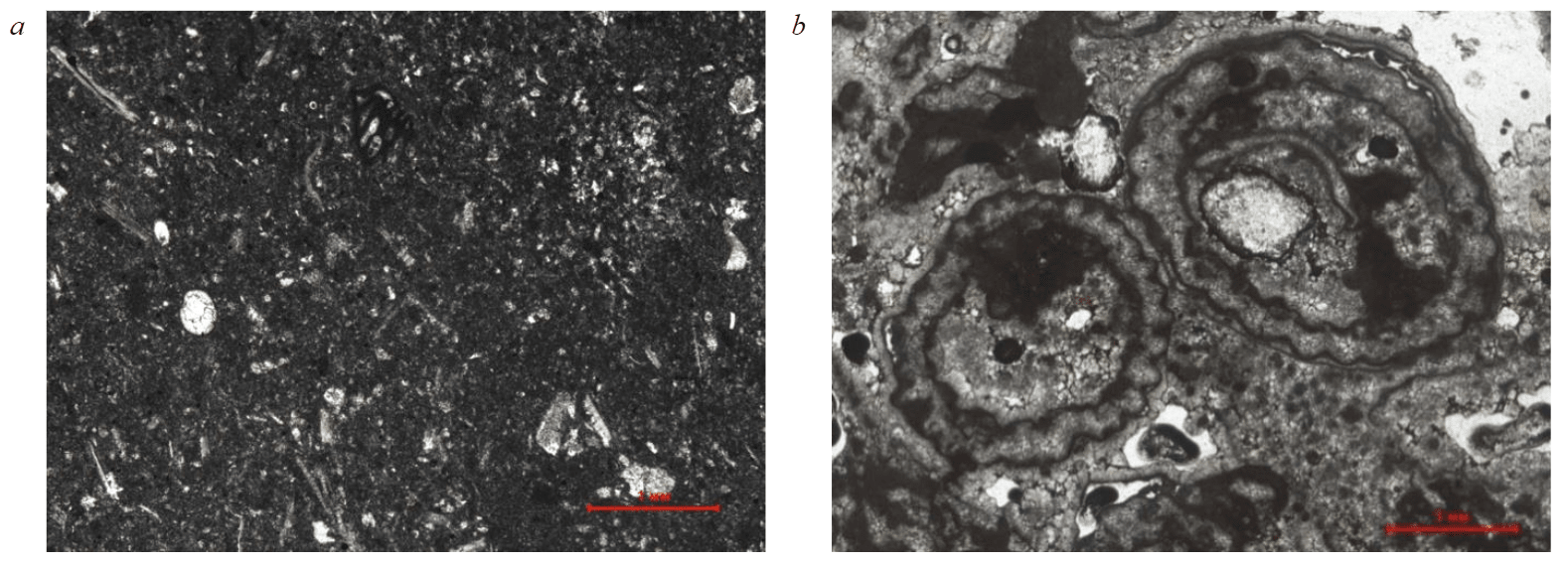
Fig.2. Photographs of thin sections of Permo-Carboniferous deposits of the Usinskoye deposit for the wackestone (a) and boundstone (b) lithotypes
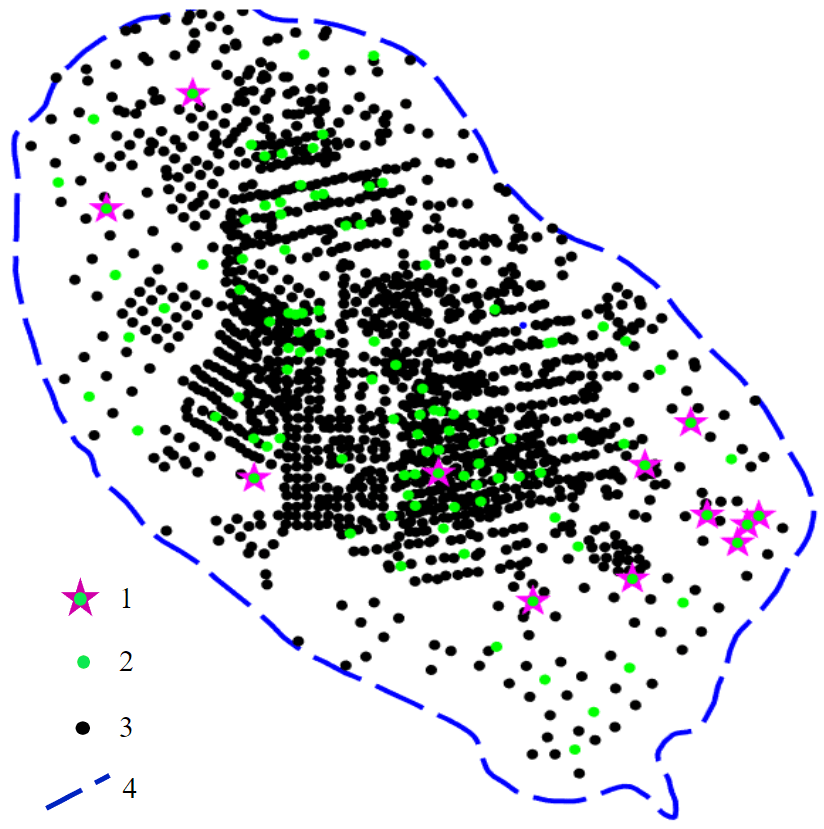
Fig.3. Examination by core and geophysical studies of wells of the Permo-Carboniferous deposit of the Usinskoye field 1 – thin rock section analysis; 2 – wells with core sampling; 3 – wells without core sampling; 4 – outer contour of the oil-water contact of the deposit
Figure 3 shows location of wells, from which we can see that the core sampling wells are evenly spaced, but on a fairly sparse grid. Twelve wells where thin sections have been analyzed are even less characteristic of the entire deposit area. The deposit volume, which is a multiple of higher density, characterizes the logging complex, which was carried out in all the wells and was the basis for 3D geological modeling. Besides, the wells with core sampling have to be the basis for justification of dependencies of indicators of well logging methods on rock porosity and permeability.
The process of building a 3D geological model of the deposit taking into account rock lithotypization can be broken down into two consecutive stages. At the first stage, the objective is to identify reservoir volumes through comparing core examination and well logging data. At the second stage, additionally involved thin section examination data enrich the 3D geological model with information to better identify lithotypes, specifically the rock porosity and permeability data taking into account the structural peculiarities. A large array of factual information (BigData) enables using of machine learning technology toward the implementation of the established goal.
The machine learning model suggests transformation of input data on the basis of implementation of multiclass classification methods [17]. In recent years, this methodological approach has been extensively used in the oil industry to differentiate between types of rock in terms of their reservoir properties and to evaluate the influence of lithology on well performance [18-20]. In this study, stages of machine learning implementation are implemented by means of Prime software based on neural network apparatus. The authors have additionally written dedicated program codes to enable automation of the process across the entire well stock.
Methodology
Use of machine learning technology in reservoir propagation modeling
In the first stage, machine learning has been used for automated identification of reservoirs based on the data of the well logging set of methods in scope of the 3D geological model of the deposit. The neural networks method has been implemented on the basis of the data of 122 wells with a full set of well logging methods and core sampling (Fig.3), for which high quality interpretation has been achieved in conjunction with field data. The array of wells was divided into two equal parts; half of the wells were included in the training sample, the other half in the examination sample. Accordingly, optimization algorithms should be developed on 61 wells of the training sample, whereas prediction reliability was evaluated on 61 wells of the examination sample.
Initially, special software tools were used to reformat the information from continuous well logging curves into discrete curves with breakdown into single interlayers. Then an optimization learning algorithm based on Levenberg – Marquardt modification (LMA) [21, 22] was used for the wells of the training sample to implement successive approximation of the initial parameter values to the sought local optimum. The LMA method is usually considered in theory as a Gauss – Newton algorithm (GNA) using the regularization parameter and the confidence region of the approach. Root-mean-square error of the model is applied as an optimization criterion [23, 24]. According to the current thinking, classification results based on LMA are considered more reliable than GNA [25]. For LMA implementation, the model characteristics were evaluated on the basis of the experiment planning procedure, where maximizing the proximity of model values to the discrete actual reservoir/non-reservoir curve was the criterion for optimizing the solutions. The LMA model consisted of two intermediate hidden layers, with the number of neurons in the first and second layer being 80 and 2, respectively. The number of consecutive “sliding window” points in the model settings was assumed to be 2.
As a result, it was found that in the absence of GRL and SPL information, the results of applying machine learning become unsatisfactory. Accordingly, use of these methods in the development of predictive solutions is recognized as mandatory. With that in mind, 36 possible situational models of machine learning were generated, which included 2 to 6 well logging methods. The classification results were tested on the wells of the examination sample. Less than 10 % prediction errors were obtained for six models: model with a set of six well logging methods; model with a set of five methods (without GGL-d); two models with a set of four methods; model with three methods (GRL, SPL, NL); model with two methods (GRL, SPL).
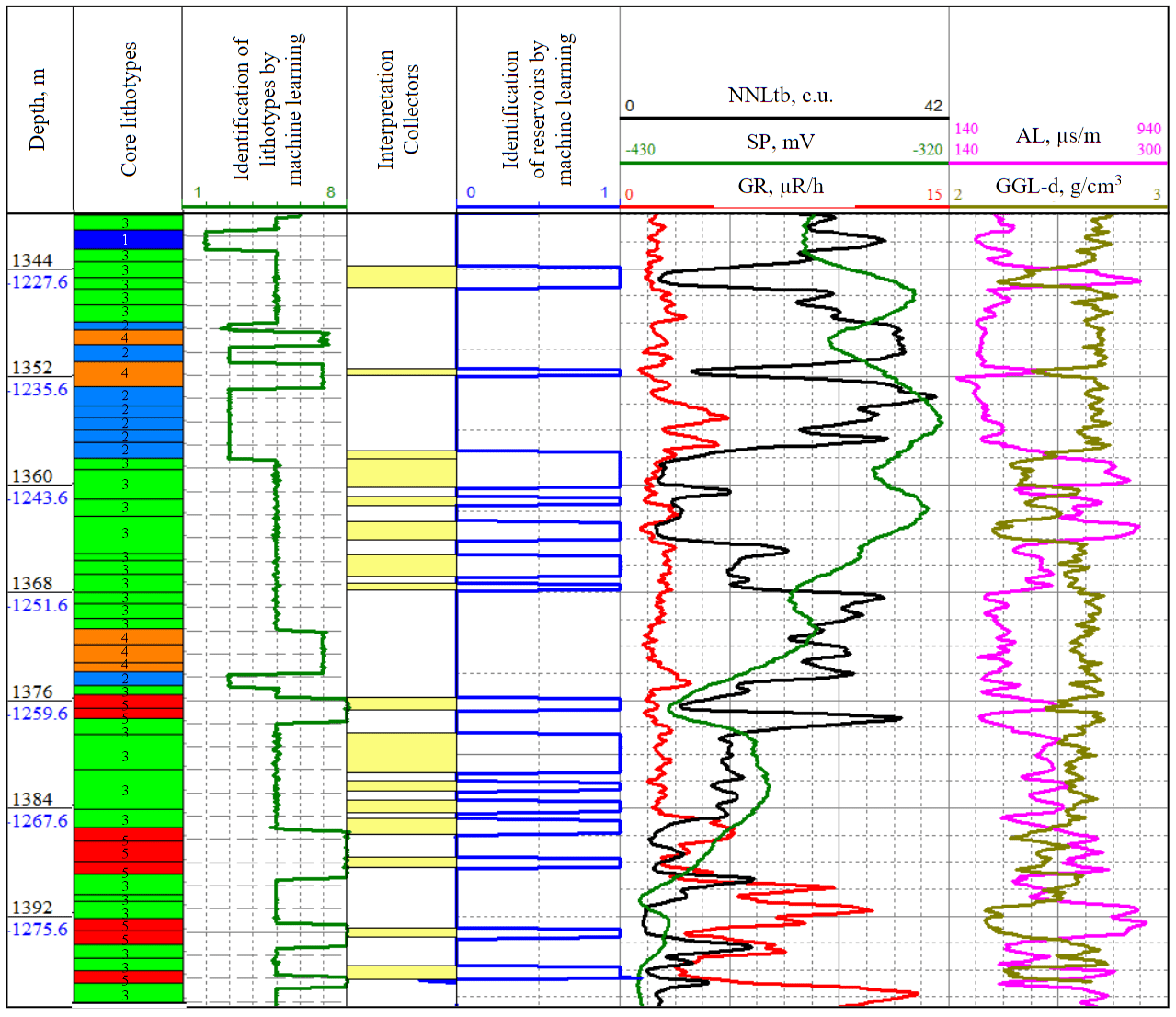
Fig.4. The result of the identification of reservoirs and lithotypes for the well using machine learning Lithotype classes: 1 – mudstone; 2 – wackestone; 3 – packstone; 4 – grainstone; 5 – boundstone
The results of automated identification of reservoirs based on well logging data using machine learning for one of the wells with core sampling are shown in Fig.4. A discrete probability curve of reservoir identification was obtained for the machine learning technology (within 0 to 1 unit fractions). Figure 4 shows that the results of reservoir identification in machine learning for this well are almost identical to the standard well logging interpretation.
Maximum repeatability of input and prediction results – 95 % (error 5 %) for the analyzed well stock was obtained with the full set of the six methods of research. In the process of exclusion of methods from the well logging set, the repeatability decreased and amounted to 91 % (error 9 %) with the use of two methods. Taking into account satisfactory reliability of prediction solutions, algorithms of automated reservoir identification were applied to the remaining 983 wells. Forecast for specific wells was carried out on the basis of the models with maximum possible use of well logging methods.
Use of machine learning technology to model the propagation of lithological rock types
The next research objective was to associate Permo-Carboniferous intervals with carbonate rock lithotypes based on machine learning. To this end, a training sample of 12 wells with thin sections examination was used. As a result, when implementing the LMA method, the geological cross-section of wells was characterized by a discrete lithotypization curve with identification of classes from 1 to 8. An example of comparison of lithotype identification using thin sections and the automated well logging method (Fig.4) shows good repeatability of the results, which amounted to 97 % for the wells of the training sample.
Comparison of lithotypes to the results of reservoir identification shows that permeable intervals are mostly associated with boundstones, somewhat less frequently with dolomitized limestones. In some cases, low-thickness intervals of permeable rock are represented by floatstones and rudstones. For mud-, wacke-, pack- and grainstones, in almost all cases, permeability and porosity are below the reservoir boundary values.
The obtained algorithms were applied to identify the rock lithotypes from the geological cross-section of the remaining wells, which resulted in building a 3D model of lithotype distribution. Figure 5, a shows a zoning diagram across the deposit area and the geological profile of lithotype distribution based on the results of the neural network method implementation.
The geological profile shows that the distribution of lithotypes in the 3D model has a pronounced stratification. This is consistent with the accepted model of Permo-Carboniferous deposit formation. Analysis of zoning distribution of lithotypes across the deposit volume shows that boundstones, which are the most promising in terms of reservoir properties, mainly occur in the vaulted part of the deposit. The distribution of lithotypes in the central part has an uncertain strip-like corridor orientation to the north, northeast in the direction of tectonic disturbance propagation. Reservoir properties of the lateral parts are mainly associated with dolomitized carbonates, which mostly occur in the northwestern and southeastern sides of the deposit.
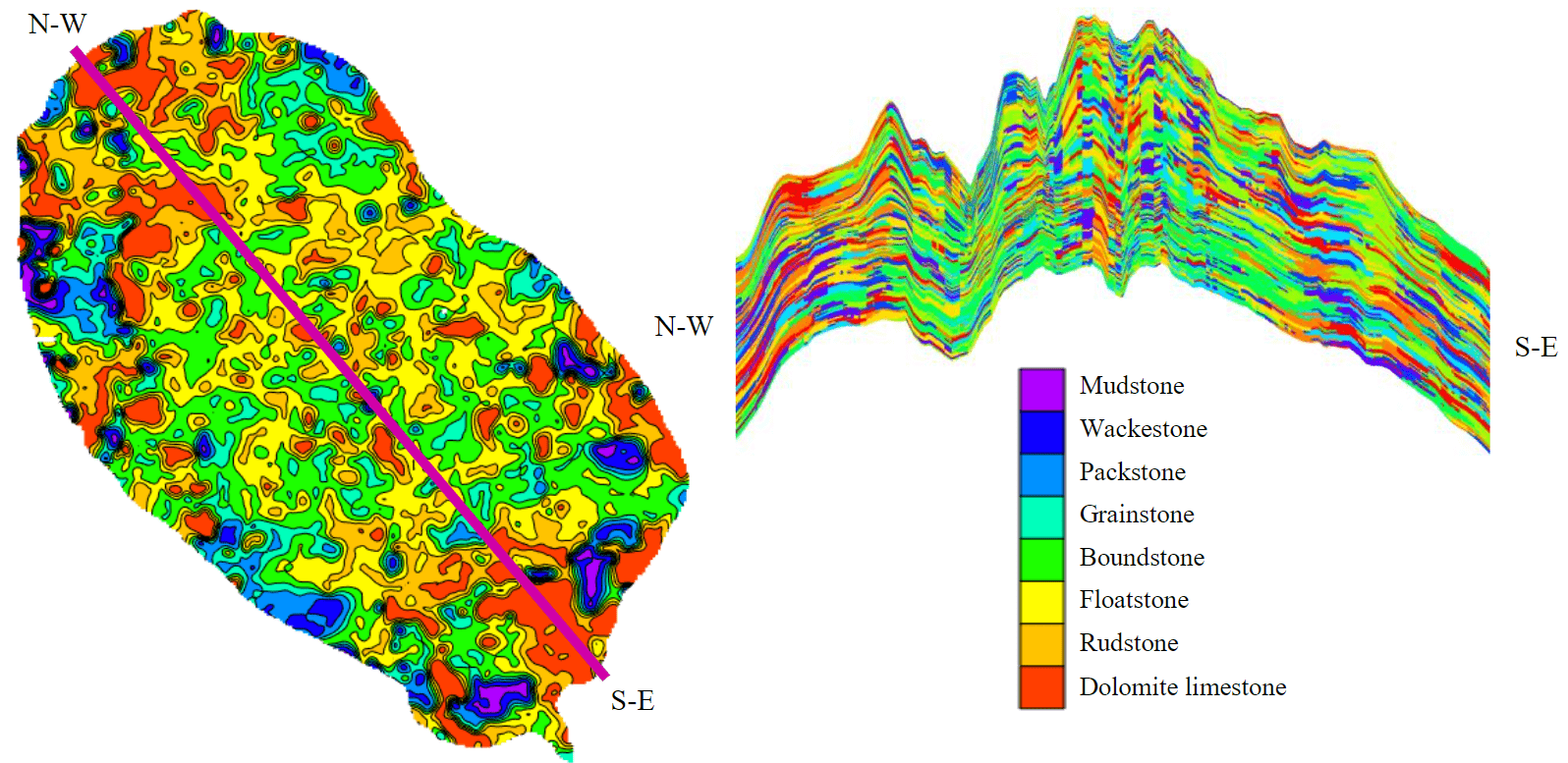
Fig.5. Distribution of lithological types of rocks according to machine learning
Use of machine learning technology in modeling the rock fracturing
Porosity and permeability of the rocks of Permo-Carboniferous deposit of the Usinskoye field mostly depend on their potential fracturing. Fracturing characteristics may be comprehensively evaluated using the tress state data of the core [26-28], hydrodynamic well studies [29-31] and well logging methods [32-34].
For the Permo-Carboniferous deposit, special well logging methods included massive studies of fracturing using an azimuthal electric Formation Micro Imager (FMI) [35, 36]. Fractures in FMI studies are displayed as sinusoids, which amplitude depends on the direction of their occurrence in relation to the well. Healed fractures are displayed on FMI images in light shades due to the resistance of minerals that fill them. Dark shades on FMI are interpreted as intervals with large pores and open natural fractures [37, 38].
According to FMI interpretation data for 35 wells, routes of the majority of open fractures are partially visualized on the image, which suggests their partial openness; development of cavernosity is observed along the routes of some of them. Natural fracturing density T ranges from 0 to 23 single fractures per 1 m of geological cross-section.
Fracture openness may be assessed using Borview software (Schlumberger):
where Rm – drilling mud resistivity, Ohm∙m; Rxo – background resistivity, Ohm∙m; dA – current rectangular window area, inch2; h – length of the fracture segment in the window, inch; Е – excess of electrical conductivity in the fracture route over the dense rock field, S; 1/K; b – individual coefficients of FMI device, taking into account wellbore coverage and actual wellbore diameter at the measuring point.
As a result, maximum openness of single fractures interpreted by FMI reached about 250 μm, with an average estimate of 15 μm across the 3D volume of the deposit.
LMA method was implemented to estimate fracturing parameters, where T and W were prediction indicators based on FMI data, and the output of well logging methods was used as an input. The training errors amounted to 2-5 %, which suggests reliability of the obtained predictions. The averaged results of W and T values distribution over the deposit area are shown in Fig.6, a, b.
Analysis of the zonality of fracture density distribution shows that the range of T values distribution across the area of the deposit is mainly relatively narrow, 3 to 6 fractures per 1 m of the cross-section. In general, the western part of the deposit is characterized by somewhat higher T values (Fig.6, a).
Fracture openness is manifested in a much greater range: according to the 3D model cube, W varies from 0.4 to 247 μm. Maximum fracture opening is established for the southern and northern parts of the deposit, but when averaged over the area, W values did not exceed 35 μm. For the vaulted part of the deposit, the calculated values of W below 5 μm are mainly observed (Fig.6, b).
In [39], by generalizing the studies of fracturing on the thin sections according to E.S.Romm, a formula was proposed to estimate the fracture permeability through density and openness:
where В – coefficient reflecting the quantity dimension (predictor), assumed equal to 1570 in calculations; А – fracture orientation coefficient, assumed equal to 0.0228 in calculations.
Formula (2) for the 3D model is used to calculate kfr values. Since parameter W in the formula is in the third degree, kfr calculated values are influenced to a much greater extent by openness and to a lesser extent by density of fractures. As a result, distribution of permeability largely repeats the zoning of fracture openness, whereas in 3D model cells, kfr varies from 0 to 1782 mD. Figure 6, c shows averaged results of kfr distribution over the deposit area.
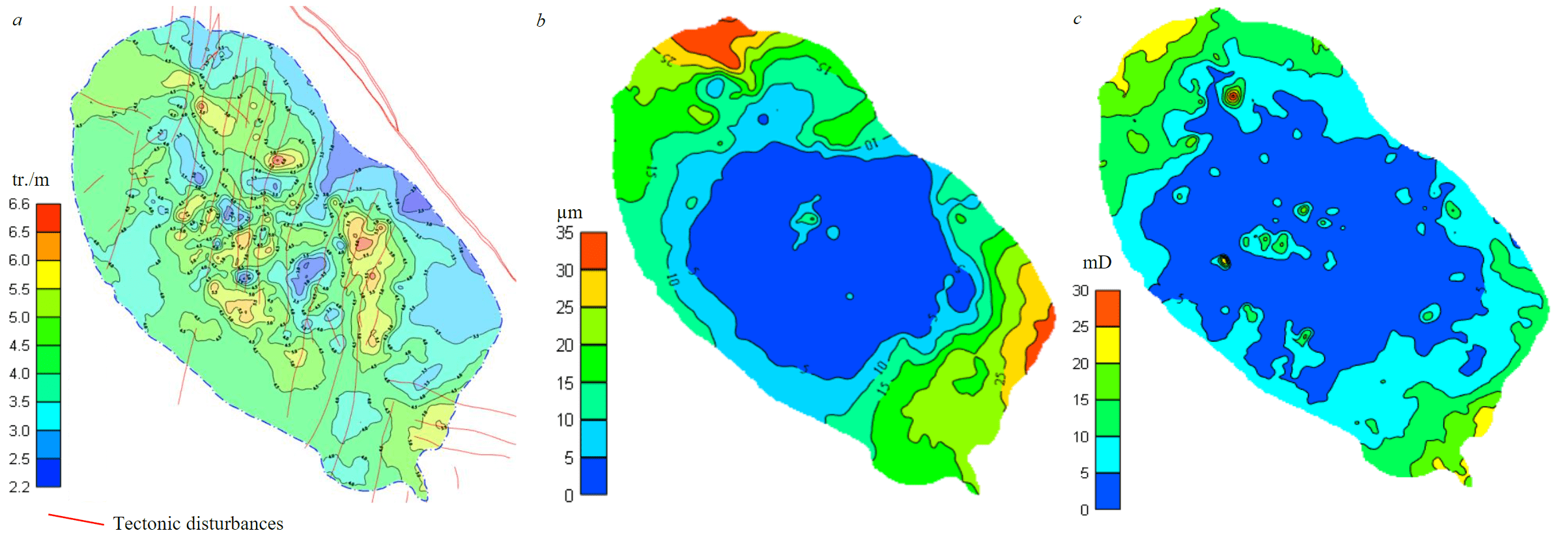
Fig.6. Density distribution (a), opening (b) and permeability (c) of fractures over the deposit area
As seen from Fig.6, c, kfr values of more than 20 mD averaged over the deposit area are confined to its northwestern and southeastern parts, which is consistent with the predominant distribution of the lithotype of dolomitized carbonates. Comparison of the obtained results with the facies analysis shows that the development of fracturing processes is mainly associated with areas of carbonate shoals. In the vaulted part of the deposit, for a large area kfr does not exceed 5 mD; ho-wever, narrow belt-like corridors of increased permeability occur occasionally. In general, variabi-lity of fracture permeability throughout the deposit volume is primarily related to the peculiarities of development of the barrier reef structures.
Conclusion
As a result of the research based on the use of Levenberg – Marquardt neural networks, algorithms for identifying rock lithotypes and fracture parameters of productive formations were developed. Volumetric zoning of rock lithotypes distribution according to Dunham classification was established for the Permo-Carboniferous oil deposit of the Usinskoye field. In each of the identified lithotypes, the density and openness of fractures have been determined based on machine learning algorithms, to further calculate fracture permeability in the deposit volume. The calculated fracture permeability volumes can be used to create geological and process models of double porosity.
In general, the errors in the implementation of machine learning were about 3-5 %, which confirms reliability of the obtained predictive solutions. The research results are incorporated in the existing 3D digital geological and process model of the deposit under study; this enables optimization of the geological and process activities at the wells.
References
- Prishchepa O.M., Borovikov I.S., Grokhotov E.I. Oil and gas content of the understudied part in the northwest of the Timan-Pechora oil and gas province according to the results of basin modeling. Journal of Mining Institute. 2021. Vol. 247, p. 66-81. DOI: 10.31897/PMI.2021.1.8
- Timonina N.N. Trend of Innovative Development of Oil and Gas Industry of Komi Republic. Vestnik of institute of geology of Komi science center of Ural branch RAS. 2014. Vol. 237. N 9, p. 25-28 (in Russia).
- Kayukov V.V., Zaytsev E.V. Investment Potential of Oil and Gas Industry in the Komi Republic. Resursy Evropeiskogo Severa. Tekhnologii i ekonomika osvoeniya. 2015. N 1, p. 91-96 (in Russian).
- Timonina N.N., Nikonov N.I. The Development Strategy of Oil and Gas Complex of the Republic of Komi. Georesursy. 2013. Vol. 52. N 2, p. 39-44 (in Russian).
- James N.P., Bourque P.A.Reefs and mound. Facies models – response to sea-level change. Hamilton: Geological Association of Canada, 1992, p. 323-347.
- Postnikov A.V., Olenova K.Yu., Sivalneva O.V. et al. Genetic void types and their distribution regularities in carbonate reservoirs of Timan-Pechora province. Exposition Oil Gas. 2022. Vol. 86. N 1, p. 22-28 (in Russian). DOI: 10.24412/2076-6785-2022-1-22-28
- Evdokimov N.V., Zhemchugova V.A. Early Permian organogenic buildups within the Northern part of Timan-Pechora basin. Moscow University Bulletin. Series 4. Geology. 2020. N 3, p. 57-65 (in Russian). DOI: 10.33623/0579-9406-2020-3-57-65
- Zhemchugova V.A., Evdokimov N.V., Poort J., Akhmanov G.G. Lower Permian Carbonate Buildups in the Northern Timan-Pechora Basin as the Main Hydrocarbon Exploration Object. Lithology and Mineral Resources. 2020. Vol. 55. N 4, p. 245-260. DOI: 10.1134/S0024490220040069
- Dunham R.J. Classification of carbonate rocks according to depositional texture. Classification of Carbonate Rocks: Classification of Carbonate Rocks Symposium. Tulsa: American Association of Petroleum Geologists, 1962, p. 108-121.
- Chuikina D.I., Serebrennikova O.V., Stakhina L.D., Altunina L.K. Salient Features of the Deposit Geological Structure in the Usinskoe Oil Field and the Composition of the Oil Produced. Exposition Oil Gas. 2018. 61. N 1, p. 18-21 (in Russian).
- Ageeva A.Yu., Putilov I. Influence of Lithological-Facies Zones on the Efficiency of Cyclic Steam Treatments (on the Example of a Permo-Carboniferous Super-Viscous Oil Reservoir of the Usinsk Field of the Komi Republic). Perm Journal of Petroleum and Mining Engineering. 2020. Vol. 20. N 4, p. 331-343 (in Russian). DOI: 10.15593/2712-8008/2020.4.3
- Diakonova T.F., Bata L.K., Saetgaraev A.D., Bronskova E.I. Geological Factors and Diagnostic Signs of Non-Water-Wetting Rocks in Timan-Pechora Province Fields. Karotazhnik. 2021. Vol. 307. N 1, p. 19-30.
- Galkin S.V., Kolychev I.Yu., Rakintsev V.A. Possibilities of Determining the Type of Wettability of the Collectors by Logging Data When Optimizing the System of Flooding of Oil Reservoirs. Bulletin of the Tomsk Polytechnic University. Geo Аssets Engineering. 2022. Vol. 333. N 1, p. 34-44 (in Russian). DOI: 10.18799/24131830/2022/1/3225
- Putilov I.S., Vinokurova E.E., Guliaeva A.A. et al. Creation of a Conceptual Geological Model Based on Lithological-Petrographic Research on the Example of the Permo-Carboniferous Deposit of the Usinskoe Deposit. Perm Journal of Petroleum and Mining Engineering. Vol. 20. N 3, p. 212-222 (in Russian). DOI: 10.15593/2712-8008/2020.3.2
- Putilov I.S., Rekhachev P.N., Gurbatova I.P. et al. Full-Size Core Epoch at Laboratory Research of Eor Technologies. Perm Journal of Petroleum and Mining Engineering. 2016. Vol. 15. N 19, p. 155-164 (in Russian). DOI: 10.15593/2224-9923/2016.19.6
- Popov N.A., Putilov I.S., Gulyaeva A.A., Vinokurova E.E. Application of deep learning technologies for studying thin sections on the example of Usinskoye oil field. Izvestiya Tomskogo politekhnicheskogo universiteta. Inzhiniring georesursov. 2020. Vol. 331. N 6, p. 100-112 (in Russian). DOI: 10.18799/24131830/2020/6/2681
- White G., Cabrera C., Palade A. et al. WasteNet: waste classification at the edge for smart bins. School of Computer Science and Statistics, 2020, p. 1-8. DOI: 10.48550/arXiv.2006.05873
- Ladeyshchikov S.V., Putilov I.S., Piatunina E.V., Laptev A.P. Forecast Facies of Terrigenous Deposits based on Highly Informed Seismic 3D and Machine Learning. Journal of Geophysics. 2018. N 5, p. 31-37 (in Russian).
- Kochnev A., Galkin S., Krivoshchekov S. et al. Application of machine learning algorithms to predict the effectiveness of radial jet drilling technology in various geological conditions. Applied Sciences (Switzerland). 2021. Vol. 11. Iss. 10. N 4487. DOI: 10.3390/app11104487
- Zaklyuchnov I.S., Putilov I.S. Using an Improved Reservoir Prediction Method based on Seismic Attributes and Well Data Comparison for Reservoir Prediction (Padunskoe Oilfield). Journal of Geophysics. 2021. N 5, p. 19-23 (in Russian).
- Amini K., Rostami F., Caristi G. An efficient Levenberg – Marquardt method with a new LM parameter for systems of nonlinear equations. Optimization. 2018. Vol. 67. Iss. 5, p. 637-650. DOI: 10.1080/02331934.2018.1435655
- Umar A.O., Sulaiman I.M., Mamat M. et al. On damping parameters of Levenberg – Marquardt algorithm for nonlinear least square problems. International Conference on Recent Trends in Applied Research (ICoRTAR), 14-15 August 2020, Nigeria. Journal of Physics: Conference Series. 2021. Vol. 1734. N 012018. DOI: 10.1088/1742-6596/1734/1/012018
- Wang H., Fan J. Convergence rate of the Levenberg – Marquardt method under Hölderian local error bound. Optimization Methods and Software. 2020. Vol. 35. Iss. 4, p. 767-786. DOI: 10.1080/10556788.2019.1694927
- Jinyan Fan, Jianchao Huang, Jianyu Pan. An adaptive multi-step Levenberg – Marquardt method. Journal of Scientific Computing. 2019. Vol. 78, p. 531-548. DOI: 10.1007/s10915-018-0777-8
- Liang Chen, Yanfang Ma. Shamanskii-like Levenberg – Marquardt method with a new line search for systems of nonlinear equations. Journal of Systems Science and Complexity. 2020. Vol. 33, p. 1694-1707. DOI: 10.1007/s11424-020-9043-x
- Dyskin A., Pasternak E., Shapiro S. Fracture mechanics approach to the problem of subsidence induced by resource extraction. Engineering Fracture Mechanics. 2020. Vol. 236. N 107173. DOI: 10.1016/j.engfracmech.2020.107173
- Zhukov V.S., Kuzmin Y.O. Experimental evaluation of compressibility coefficients for fractures and intergranular pores of an oil and gas reservoir. Journal of Mining Institute. 2021. Vol. 251, p. 658-666. DOI: 10.31897/PMI.2021.5.5
- Galkin S.V., Krivoshchekov S.N., Kozyrev N.D. et al. Accounting of geomechanical layer properties in multi-layer oil field development. Journal of Mining Institute. 2020. Vol. 244, p. 408-417. DOI: 10.31897/PMI.2020.4.3
- Sergeev V.L., Nguyen Phuong Thac Hoai. Models and Algorithms for Adaptive Interpretation of Combined Well Test Data of Intelligent Wells. Bulletin of the Tomsk polytechnic university. Geo assets engineering. 2018. Vol. 329. N 10, p. 67-75 (in Russian).
- Ghadami N., Rasaei M.R., Hejri Sh. et al. Consistent porosity – permeability modeling, reservoir rock typing and hydraulic flow unitization in a giant carbonate reservoir. Journal of Petroleum Science and Engineering. 2015. Vol. 131, p. 58-69. DOI: 10.1016/j.petrol.2015.04.017
- Jiawei Li, Gang Zhao, Xinfeng Jia, Wanju Yuan.Integrated study of gas condensate reservoir characterization through pressure transient analysis. Journal of Natural Gas Science and Engineering. 2017. Vol. 46, p. 160-171. DOI: 10.1016/j.jngse.2017.07.017
- Sait I. Özkaya. Fracture modeling from borehole image logs and water invasion in carbonate reservoirs with layer – bound fractures and fracture corridors. Journal of Petroleum Science and Engineering. 2019. Vol. 179, p. 199-209. DOI: 10.1016/j.petrol.2019.04.052
- Kashnikov Y.A., Ashikhmin S.G., Kukhtinskii A.E., Shustov D.V. The relationship of fracture toughness coefficients and geophysical characteristics of rocks of hydrocarbon deposits. Journal of Mining Institute. 2020. Vol. 241, p. 83- 90. DOI: 10.31897/PMI.2020.1.83
- Xie Fang, Zhang Chengsen, Liu Ruilin, Xiao Chengwen.Production prediction for fracture-vug carbonate reservoir using electric imaging logging data. Petroleum Exploration and Development. 2018. Vol. 45. Iss. 2, p. 349-356 (in Chinese). DOI: 10.11698/PED.2018.02.19
- Guodong Jin, Huilin Xing, Tianbin Li et al. An integrated approach of numerical well test for well intersecting fractures based on FMI image. Lithosphere. 2022. Iss. 1. N 4421135. DOI: 10.2113/2022/4421135
- Nabiei M., Yazdjerdi K., Asadi A., Soleimany B. Analysis of fractures in the Dalan and Kangan carbonate reservoirs using FMI logs: Sefid-Zakhur gas field in the Fars province, Iran. Carbonates and Evaporites. 2021. Vol. 36. N DOI: 10.1007/s13146-021-00686-w
- Kharitontseva P., Gardiner A., Chernov D. et al. An integrated approach for formation micro-image rock typing based on petrography data: a case study in shallow marine carbonates. Geosciences (Switzerland). 2021. Vol. 11. Iss. 6. N 235. DOI: 10.3390/geosciences11060235
- Watton T.J., Cannon S., Brown R.J. et al. Using formation micro-imaging, wireline logs and onshore analogues to distinguish volcanic lithofacies in boreholes: Examples from Palaeogene successions in the Faroe-Shetland Basin NE Atlantic. Geological Society London Special Publications. 2014. Vol. 397. Iss. 1. p. 173-192. DOI: 10.1144/SP397.7
- Belonovskaya L.G., Bulach M.Kh., Gmid L.P. The role of fracturing in the formation of capacitive-filtration space of complex reservoirs. Neftegazovaya geologiya. Teoriya i praktika. 2007. Vol. 2, p. 28 (in Russian).