Geochemistry of beryl varieties: comparative analysis and visualization of analytical data by principal component analysis (PCA) and t-distributed stochastic neighbor embedding (t-SNE)
- 1 — Ph.D., Dr.Sci. Chief Researcher Institute of Precambrian Geology and Geochronology of the Russian Academy of Sciences ▪ Orcid
- 2 — Postgraduate Student Saint Petersburg Mining University ▪ Orcid
- 3 — Ph.D. Senior Researcher Institute of Precambrian Geology and Geochronology of the Russian Academy of Sciences ▪ Orcid
Abstract
A study of the trace element composition of beryl varieties (469 SIMS analyses) was carried out. Red beryls are distinguished by a higher content of Ni, Sc, Mn, Fe, Ti, Cs, Rb, K, and B and lower content of Na and water. Pink beryls are characterized by a higher content of Cs, Rb, Na, Li, Cl, and water with lower content of Mg and Fe. Green beryls are defined by the increased content of Cr, V, Mg, Na, and water with reduced Cs. A feature of yellow beryls is the reduced content of Mg, Cs, Rb, K, Na, Li, and Cl. Beryls of various shades of blue and dark blue (aquamarines) are characterized by higher Fe content and lower Cs and Rb content. For white beryls, increased content of Na and Li has been established. Principal Component Analysis (PCA) for the CLR-transformed dataset showed that the first component separates green beryls from other varieties. The second component divides pink and red beryls. The stochastic neighborhood embedding method with t-distribution (t-SNE) with CLR-transformed data demonstrated the contrasting compositions of green beryls relative to other varieties. Red and pink beryls form the most compact clusters.
Introduction
Peculiarities of trace and rare-earth elements distribution in accessory and rock-forming minerals are used as a basis for solving many issues of petrogenesis of igneous rocks, recently with the use of local microanalysis of minerals (SIMS and LA-ICP-MS methods) [1-3]. The study of zircon, the most informative geochronometer, has received the greatest development [4-6]. Much less research has been devoted to the trace element composition of beryl. The results of studying the distribution of minor and trace elements in beryl, along with the study of microinclusions, as well as obtaining various spectroscopic data, are widely used to establish the genesis conditions and characteristics of the mineral-forming environment [7-9]. However, a significant share of such studies refers specifically to the gem-quality variety of green beryl, the emerald [10-12]. The study of the trace element composition of emerald, in addition to solving a variety of genetic problems, has shown the possibility of their application in the design of various discrimination diagrams, as well as in determining the geographical origin of this variety of beryl [13-15]. It should be noted that a comparative analysis of the trace element composition of all major varieties of beryl based on advanced local research methods and a representative number of analytical data has not been carried out before. Filling this gap is the goal of this study.
The research materials and methodology of the study
Research Materials
The mine-ralogical and geochemical study was carried out on beryl samples from the educational collection of the Mining Museum, as well as those provided by colleagues. In total, 111 samples of beryl were analyzed (469 local analyzes on the ion microprobe, taking into account the rejection of outliers), which were divided into seven varieties (see Table). It should be emphasized, that the unique collections of the Mining Museum have made a decisive contribution to the study (94 samples, on which about 300 analyzes were performed). When distinguishing varieties, the color of beryl was taken as the basis, a feature most often used both in mineralogical and geochemical studies, as well as in gemology. For green beryls (emeralds of varying degrees of color saturation and transpa-rency), 210 analyzes were performed in 37 samples. Red beryl (bixbite), which is an extremely rare variety of beryl, was analyzed in one sample (7 analyzes). The composition of pink beryls (morganites) was studied in eight samples (26 analyzes). The sample of yellow beryls (heliodors,
davidsonites) was less representative and consisted of three samples (28 analyzes). The group of beryls of various shades of blue (aquamarines, bazzites) and dark blue (maxixe beryl) is considered in this work under the general term “aquamarines” and includes 23 samples (87 analyzes). White opaque beryls, whose intensity and type of coloring did not allow them to be included in other color-segregated varieties, were included in the group of white beryls. There are 16 samples among the white beryls, in which 62 local analyzes were performed. The group of transparent colorless beryls (goshenites, rosterites) consists of 23 samples (49 analyzes). Primary analytical data on the composition of yellow beryls were previously partially published in [16], on the composition of green beryls – in [17, 18]. The beryl grains selected for analysis were mounted in epoxy resin in standard samples (washers) 1 inch in diameter and grounded approximately to the middle of the grain, bringing it to the surface of the washer.
Analytical methods
The contents of trace and minor elements, water and volatiles in beryl were determined by secondary ion mass spectrometry (SIMS) using a Cameca IMS-4f ion microprobe at the Yaroslavl branch of the Institute of Physics and Technology (IPT) named after K.A.Valiev, Russian Academy of Sciences. The basics of the measurement technique corresponded to those reported in [19-21]. The analyzes were carried out in two steps using different protocols for the determination of volatiles (Cl, F, H) and light (B, Li) impurity elements and the main set (Na, Mg, P, K, Ca, Sc, Ti, V, Cr, Mn, Fe, Co, Ni, Ga, Rb, Cs).
Primary O2− ions were accelerated to about 10 keV and focused at the sample surface into a spot about 20−30 µm in diameter. The intensity of primary ion current was 5 nA (protocol “volatiles”) and 1.5 nA (main protocol). Positive secondary ions were collected from an area of 10 µm (protocol “volatiles”) or 25 µm (main protocol) in diameter, limited by a field aperture. Secondary ions with energies of 75 eV ÷ 125 eV were used to form an analytical signal (energy filtration technique). Three counting cycles were carried out with a discrete transition between mass peaks within a given set. The absolute concentrations of each element were calculated from the measured intensities of positive single-atom secondary ions, which were normalized to the intensity of secondary 30Si+ ions. Calibration curves were based on the measurements of the set of well-characterized standard samples [22, 23].
Quantification of phosphorus, scandium, iron, nickel and cobalt was carried out taking into account isobaric mass peak interference:
- The phosphorous content was estimated using stripping procedure. The contribution of 30Si1H+ to the measured intensity of mass peak at 31 a.m.u. was determined from the measured intensity of mass peak at 29 a.m.u., that is the interference of 29Si+ and 28Si1H+, using the known abundances of natural silicon isotopes.
- When quantifying scandium, the subtraction of 29Si16O+ + 28Si17O+ ion signal in the range of 45 a.e.m. required additional measurement of signal intensity at the 44th mass (28Si16O+ + 44Ca+). The contribution of 44Ca+ was estimated by recalculating from measured 42Ca+ intensity and known natural calcium isotope abundances [10].
- The 56Fe+ and 59Co+ signal was corrected regarding to the Si2+ cluster ion spectrum, assuming that the Si+/Si2+ intensity ratio for this matrix is known and it does not vary significantly if the sample charging is well controlled.
- The contribution of 46Ti16O+ was taken into account when calculating nickel concentration by measuring the 62nd nickel isotope. TiO+ signal intensity was estimated by measuring 47Ti+ ion current intensity, and 47Ti+/46Ti16O+ was measured in standard glasses. Because Ti concentration in beryl is low (15-34 ppm), the corrected Ni concentration is very close to the uncorrected one.
Trace elements composition of beryl varieties
Note. Min - minimum. Max - maximum. IQR - interquartile rage, content is given in [ppm].
Ion probing of water required known approaches to decrease the background level. Before measurements, each sample was kept for at least 12 h in a mass spectrometer’s analytical chamber, where high vacuum conditions were maintained. Analysis was preceded by the ion sputtering of conducting gold film and a surface pollutant layer at the area to be analyzed. Then the procedure of auto adjustment of the sample potential was applied. The static primary beam spot overlapped the field of view of the secondary ion optics (10 µm in diameter) centered at the presputtered crater (~40 × 40 μm). An anhydrous silicate (olivine) grain introduced into each sample mount was used to measure 1H+ signal background. Water concentration was calculated from the 1H+/30Si+ ion current ratio based on calibration relationships:
C[H2O]/C[SiO2] = (I(1H+) − I(1H+)bg)/I(30Si+) × RSF × K(SiO2),
C[H2O] and C[SiO2] are H2O and SiO2 concentrations in wt.%; I(1H+), I(1H+)bg and I(30Si+) are measured secondary ion intensities in imp/s; I(1H+)bg is the background signal intensity; and RSF is the relative sensitivity factor. The correction coefficient K(SiO2) takes into account the dependence of RSF on SiO2 concentration for which linear approximation was used:
K(SiO2) = (1 − (SiO2 − 50) × 0.0185).
Calibrations were obtained using samples of natural and artificial glasses (in all, 28 standard samples) covering a wide range of variation in SiO2 (41-77 wt.%) and water (0.1-8 wt.%) concentrations ([24-28], unpublished data of R.E.Bocharnikov). The results of calibration show that the maximum deviation from the reference value was no more than 15 %, and the calculation error was 7 %.
A similar approach was used for calculating fluorine and chlorine concentrations. Standard glass NIST-610 [29] was used as a monitor before an analytical session. The trace element measurement error did not exceed 10 % for concentrations higher than 1 ppm and 20 % for concentrations in the range of 0.1-1 ppm. The trace and minor element detection limit ranges mainly from 0.005 to 0.010 ppm.
Processing of analytical data
Primary analytical data was checked for outliers. As a rule, such points resulted from the occurrence of microinclusions of another mineral in the area of analysis. For example, a microinclusion of biotite causes an anomalously high content of K, Ti, Fe, and some other elements compared to neighboring analytical points in the same beryl sample. Such analyzes were excluded from the sample. The entry of a gas-liquid inclusion into the analysis field causes an anomalous increase in the content of Na and Cl. In such cases, the outliers for specific elements were replaced by the average content of a given element within the same grain. When the element content was below the detection limit (in single cases), the value of this limit was used for statistical processing. Since the scheme for taking into account isobaric overlays for P was not always effective, the data on this element was completely excluded from the statistical calculations.
Statistical discrimination of analytical data on the trace element composition of beryl was carried out by principal components analysis (PCA) and stochastic neighbors embedding with t-distribution (t-SNE).
Principal component analysis (PCA) is a type of factor analysis developed in 1933 and has been successfully used in geology for more than 50 years in the statistical analysis of rocks and minerals [30-32]. Principal component analysis (PCA) is a set of techniques that allow you to identify the leading factors that determine the variance of the random variables under study. In this case, these values are a set of trace elements, the content of which in beryl was analyzed by the SIMS method. The PCA method makes it possible to reduce the dimension of the feature space and describe feature variations with the smallest number of variables (components, factors) with maximization of the variance between them. In other words, the most distinguishable objects appear far apart in the feature space. Since PCA is based on a multivariate normal distribution, this requirement was tested. Checking the type of distribution of trace elements in beryl showed that only water is normally distributed. The distribution law of the remaining trace elements is lognormal or another one, which cannot be precisely determined. Therefore, in the first case of the PCA application, the logarithms of the initial analytical data on beryl, except for the water, were taken. In the second case, a logarithmic normalization of all data was carried out using a centered log-ratio transformation (CLR transformation) [33], which takes into account the distortions inherent in compositional data [34]. The CLR transformation was performed by the CoDaPack 2.0 software [35]. Calculations by PCA were performed using the Statistica 7.0 software package.
The t-distribution stochastic neighbor embedding method (t-SNE method) was developed in 2008 [36]. This method is used for the study and visualization (in two-dimensional or three-dimensional space) of multidimensional data, including beryl geochemistry [37]. The t-SNE algorithm calculates a measure of similarity between pairs of objects in feature space with high and low dimensions. Then it tries to optimize these two similarity metrics. Currently, t-SNE is considered one of the best dimensionality reduction imaging techniques. One of the parameters that most strongly affects the result of visualization is perplexity. Perplexity can be considered as the degree of information entropy, which indicates how many neighboring points are taken into account when optimizing t-SNE. When calculating, we used the recommended [38] range of perplexity values from 10 to 50. As in the case of the PCA method, calculations by the t-SNE method were performed both for primary analytical data in logarithmic form (except water) and for CLR-transformed data. The calculations were performed using Python programming language.
Results and discussion
Statistical characteristics of beryl varieties
The Table shows the main statistical characteristics for the considered beryl varieties. Whereas distribution law for all trace elements, except for water, is not normal, we use the median value (Med) instead of the mean one. The minimum (Min) and maximum (Max) value of each element is also given. The least outlier-dependent measure of scattering was the interquartile range (IQR), which is the difference between the 75th and 25th percentiles [39].
Transition metals, and Mg, which is a minor element in beryl, as well as the scattered element Ga (the geochemical twin of Al), replace Al in the octahedral Y position [40-42]. Green beryls stand out sharply for their Cr content. For them, the median value is 254 ppm. In other varieties of beryl, the median value of Cr is extremely low and amounts to about 1-3 ppm. The highest value of 2.8 ppm was found for red beryls and the lowest of 0.7 ppm for yellow ones.
Green beryls also have a maximum content of V (88 ppm). Among other varieties, white beryls are distinguished by an increased content of V (12 ppm). In the remaining groups, the average V content ranges from 0.6 ppm in red and yellow to 2.8 ppm in colorless beryls.
The Ni content is maximum in red beryls, where its median value is 46 ppm. Among the other groups, green beryls stand out by their Ni content (with a median value of 6.1 ppm); in the rest, the Ni median values vary from 0.5 ppm (yellow beryls) to 3.6 ppm (white beryls).
The Co content in beryls of all varieties is at the same low level, no not exceeding 1 ppm.
The median values of Sc are noticeably higher in red, green, and yellow beryls (26-43 ppm). In the other groups, it is quite uniform and amounts to 3.6-6.3 ppm.
Red beryls are sharply differed by the increased content of Mn with the median values of 2861 ppm. For the other varieties, the median content of this element, ranging from 77 to 93 ppm, is fairly sustained.
Mg content is maximum in green (median is 1809 ppm) and minimum in pink (23 ppm) and yellow (12 ppm) beryls. In the other groups, the median Mg content is intermediate and varies from 360 to 833 ppm.
Red beryls are characterized by higher Fe content (the median value is 11569 ppm). Among the other varieties, aquamarines contain more Fe (2712 ppm). The minimum amount is recorded in pink beryls (138 ppm). In the remaining groups, the median Fe content lies in the range of 1045-1690 ppm.
The median Ti content reaches an abnormally high value of 2144 ppm in red beryls. For other varieties, it ranges from 3.7 to 12 ppm.
Red beryls are also characterized by an increased content of Ga, with a median value of 41 ppm. In other varieties, the median content of Ga varies between 7.1 and 17 ppm.
Large-ion lithophile elements (LILE) enter the crystal structure of beryl in the channels between the rings of silicon-oxygen tetrahedra [11]. Together with this group, we will consider the behavior of minor elements Na, K, and Ca, as well as Li, replacing Be.
The maximum median Cs content (16322 ppm) is set for pink beryls. In red beryls, the Cs content is lower (2613 ppm). In the remaining beryl groups, the median Cs values vary from 493 (white beryls) to 187 ppm (aquamarines).
Red beryls are characterized by an increased content of Rb (median value is 433 ppm). Pink beryls have less Rb (median value is 130 ppm). In the remaining groups, the Rb content is significantly lower, varying from 29 to 6.5 ppm. Yellow beryls and aquamarines differ in the minimum content of Rb.
The Na content is quite high in pink, white, and green beryls (the range of median values is 7862-5065 ppm). The minimum Na content is typical for red (median is 791 ppm) and yellow beryls (517 ppm).
Red beryls differ sharply in K content (median value is 1231 ppm). In the other groups, K is noticeably less concentrated: from 360 ppm (pink beryls) to 79 ppm (yellow beryls).
The distribution of Ca among beryl varieties is relatively uniform. In white, green and red beryls, Ca content is higher (240-194 ppm) compared to the other groups (90-44 ppm).
The maximum Li content is set in pink (4588 ppm), followed by white beryls (672 ppm). The minimum median content is noted for yellow beryls and reaches 71 ppm. In the remaining groups, the average Li content is in the range of 285-188 ppm.
The average B content is uniformly low (no more than 1 ppm) for all varieties of beryls, except red (6.4 ppm).
In addition to LILE, beryl structural channels may include water molecules and/or OH groups, as well as halogens (Cl and F).
The maximum average water content was recorded for pink beryls (33981 ppm), and slightly less was established for green beryls (29575 ppm). In the series of white – colorless – aquamarine – yellow beryls, the average water content varies from 25410 to 12537 ppm. An abnormally low water content (109 ppm) was noted in red beryls.
Pink beryls are also distinguished by an increased content of Cl (median value is 8545 ppm). The minimum content was recorded for yellow beryls (157 ppm). In the remaining groups, the average Cl content falls within the range of 1541-493 ppm.
The F content varies within relatively narrow limits from 22 ppm to 4.9 ppm for white and red beryls, respectively.
If we compare beryl varieties by trace element composition, then red beryls stand out the most. The maximum median content of some transition metals (Mn, Fe, Ti, Ni, and Sc) was recorded for them. It is noteworthy that in red beryls, the median values of the listed elements (except for Sc) are several times higher than in the other groups. The excess of the Ti content by more than two hundred times is especially noticeable. Also in red beryls, there is an accumulation of a number of lithophile elements: Cs, Rb, and K. However, the Na content is low and corresponds more to the minimum value established for yellow beryls. The geochemical feature of red beryls is an anomalously low water content, which differs hundreds of times from other groups. The halogen content is comparable with other beryl varieties.
A characteristic feature of pink beryls is an increased content of LILE. Their Cs content is more than 20 times higher than that of other beryl groups, and their Li content is more than 15 times higher. Pink beryls are also distinguished by their high Na and Rb content. In pink beryls, the maximum average content of volatile components (water and chlorine) is recorded. The content of transition metals in them is at the level of other varieties of beryls, while for Fe and Mg it is significantly lower. The increased content of elements of the LILE group and volatiles for pink beryls reflects the composition of the mineral-forming environment in greisens, hydrothermal and pneumatolytic deposits of the metasomatic type, where this variety occurs.
Green beryls are expectedly distinguished by the maximum content of transition metal chromophores (Cr and V). They also have the maximum content of Mg and increased content of Ni and Sc (compared with other varieties). Most likely, this feature of the composition is due to the fact that the protolith for green beryls, as a rule, is mica, rich in the above elements. Previously, it was found that the content of alkali metal and Mg impurities are higher in green beryls from schist deposits compared to samples from deposits of the Columbian type [43]. Among the lithophile elements, the elevated content was found for Na and water only. The content of other lithophile and volatile elements is on the average level, and the Cs content is minimal compared to other beryl varieties.
The group of yellow beryls is characterized by a moderate content of transition metals and a lower content of lithophile ones. This is especially true for the depletion in K, Na, and Li. Yellow beryls also have a reduced water and chlorine content.
In comparison to other types of beryls, aquamarines do not have any specific composition. They recorded an increased content of Fe and a lower content of such alkali metals as Cs and Rb.
White beryls are characterized by high content of Na and Li. For colorless beryls, no significant geochemical features have been identified.
The principal component analysis (PCA)
In the case of statistical processing of the logarithmic data (except the normally distributed water content) on the trace element composition of beryls of all varieties by the PCA method, it was found that the contribution of the three principal components in the total variance is about 64 %. On the factor loadings diagram for the first and second principal components with a weight of 32 and 17 %, respectively, two groups of elements are separated by the first principal component with positive loads: K (0.69), Na (0.85), and water (0.76), on the one hand, and V (0.63), Cr (0.68), Mg (0.76), Co (0.74), Ni (0.79), and Ca (0.80), on the other (Fig.1, a). Only Ga (–0.42) has a negative load by the first principal component. For the second principal component, Sc (0.63), V (0.59), Cr (0.57), and Mg (0.45) have the highest positive loads, while Li (–0.83), Cs (–0.73), Rb (–0.54), and Cl (–0.68) have the highest negative ones (modulo). Most likely, the first and second principal components can be interpreted as composition factors of the mineral-forming environment. In the space of the first and second principal components V, Cr, Mg, Co, Ni, and Ca characterize the composition of the protolith (mica), in which the green beryls are mainly formed. K, Na, and water by the first principal component reflect the entry of these elements during metasomatism. According to the second principal component, loads of Li, Cs, Rb, and Cl are associated with hydrothermal metasomatic processes, such as the formation of greisens, rare-metal pegmatites, and other rocks, in which pink beryls enriched with these impurity elements crystallize.
On the diagram of the values of the first and second principal components (Fig.1, b), the compact field of imaging points of pink beryls is most noticeably distinguished. The imaging points of green beryls occupy a much wider area, with at least half of the points highlighted by the maximum values of the first or second principal component. Yellow beryls are characterized by negative values of the first principal component, for which Ga also has negative values. This may be since the protolith for yellow beryls is high alumina rocks, which also contain significant amounts of Ga, a scattered element and geochemical twin of Al. It can be noted that the field corresponding to the compositions of green beryls minimally overlaps with the fields of aquamarines and colorless beryls.
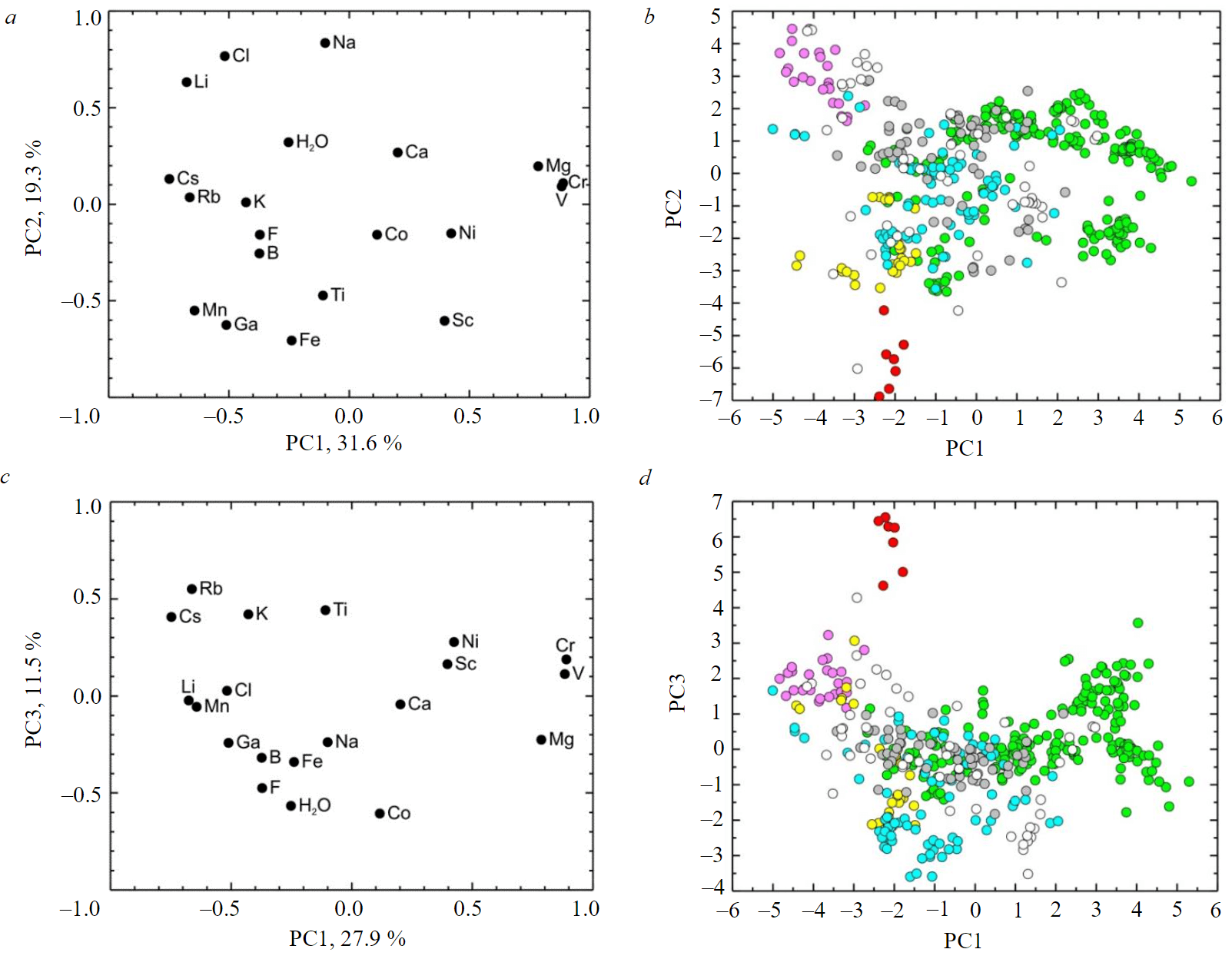
Fig.1. Visualization of beryl composition data by PCA (after taking the logarithm): in the coordinates of the first and second principal components of the load diagram (a) and the principal component values (b); the same in the coordinates of the first and third principal components (c, d). The percentage indicates the weight of the corresponding principal component (PC). Here and in other figures, the points of composition of beryls are shown: 1 – green, 2 – red, 3 – pink, 4 – yellow, 5 – aquamarine, 6 – white, and 7 – colorless
In the coordinates of the first and third (14 % weight) principal components (Fig.1, c), the highest modulo negative loadings for the third principal component are Mn (–0.76), Fe (–0.65), Ti (–0.61), and Ga (–0.57). Of these elements, only Fe and Mn are close on the diagram. No single element stands out with positive loads, with water showing the highest load value.
In the diagram of the values of the first and third principal components (Fig.1, d), the imaging points of red beryls are sharply singled out by the negative values of the third principal component. The other varieties are not distinguished in any way by the third principal component. The third principal component may be interpreted as a factor of the red beryls formation as a result of autometasomatic alteration of rhyolitic tuffs at the late magmatic stage [44]. It is believed that the red color of this variety is due precisely to the impurity of Mn [45].
In the case of statistical processing by the principal component analysis of the data on the trace composition of beryl, previously normalized by CLR method, it was found that the contribution of the three principal components in the total variance is about 59 %. On the load diagram for the first and second principal components with weights of 28 and 19 %, respectively, Cr (0.89), V (0.88), and Mg (0.79) are visibly isolated, forming a compact association with positive loadings on the first principal component (Fig.2, a). Approximately the same negative loads on the first principal component have pairs of elements (Li (–0.68) and Cl (–0.52), Cs (0.75) and Rb (–0.66), Mn (–0.64) and Ga (–0.51), differing among themselves by loads on the second main component. The second principal component has maximum positive loads of Na (0.83), Cl (0.77), and Li (0.63), negative loads of Mn (–0.55), Ga (–0.63), and Fe (–0.71), and separately located Sc (–0.60). All of the elements allocated by the second principal component, except for Sc, have negative load values by the first principal component.
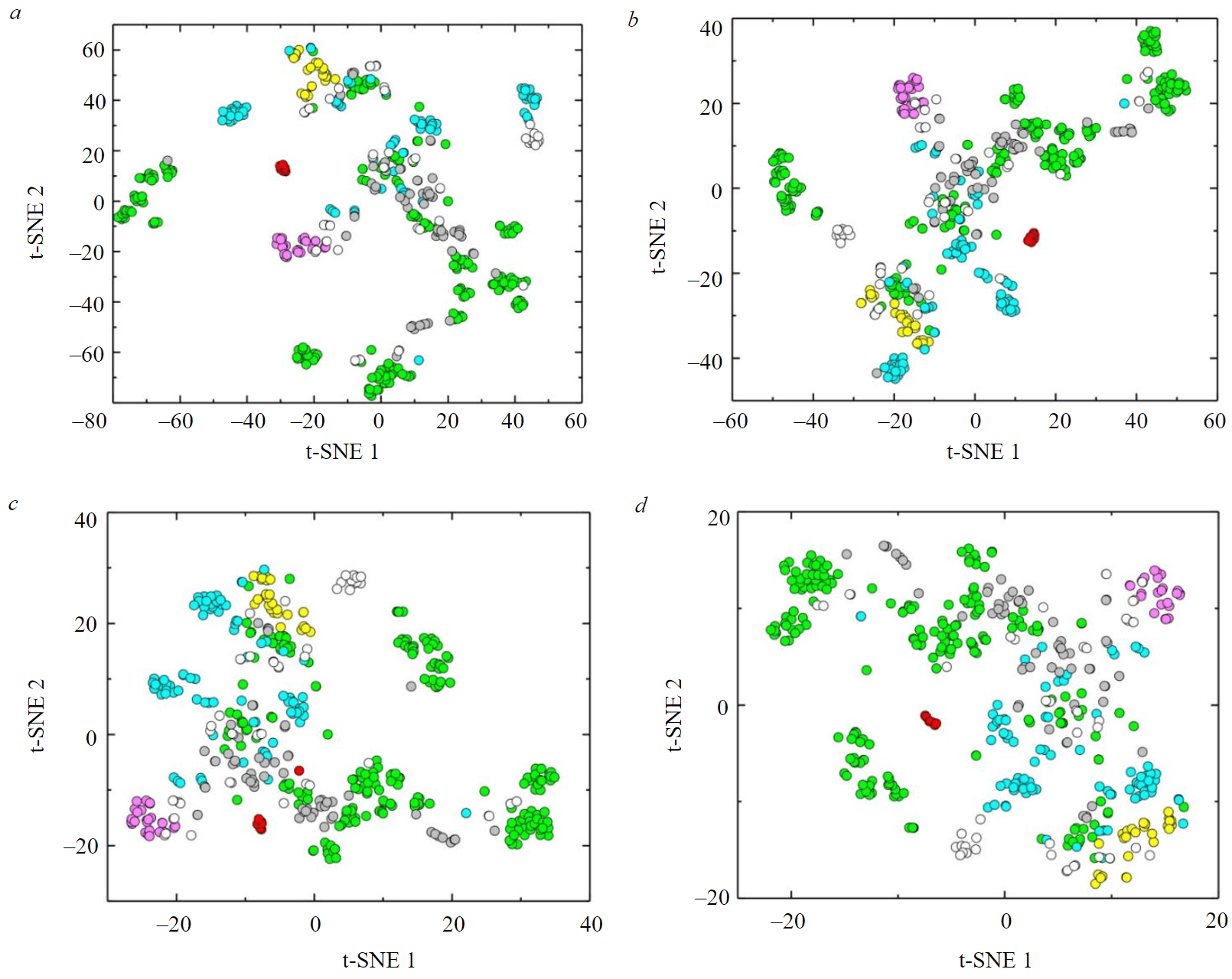
Fig.2. Visualization of beryl composition data by PCA method (after CLR transformation) in coordinates of the first and second principal components of the load diagram (a) and principal component values (b); the same in coordinates of the first (c) and third (d) principal components
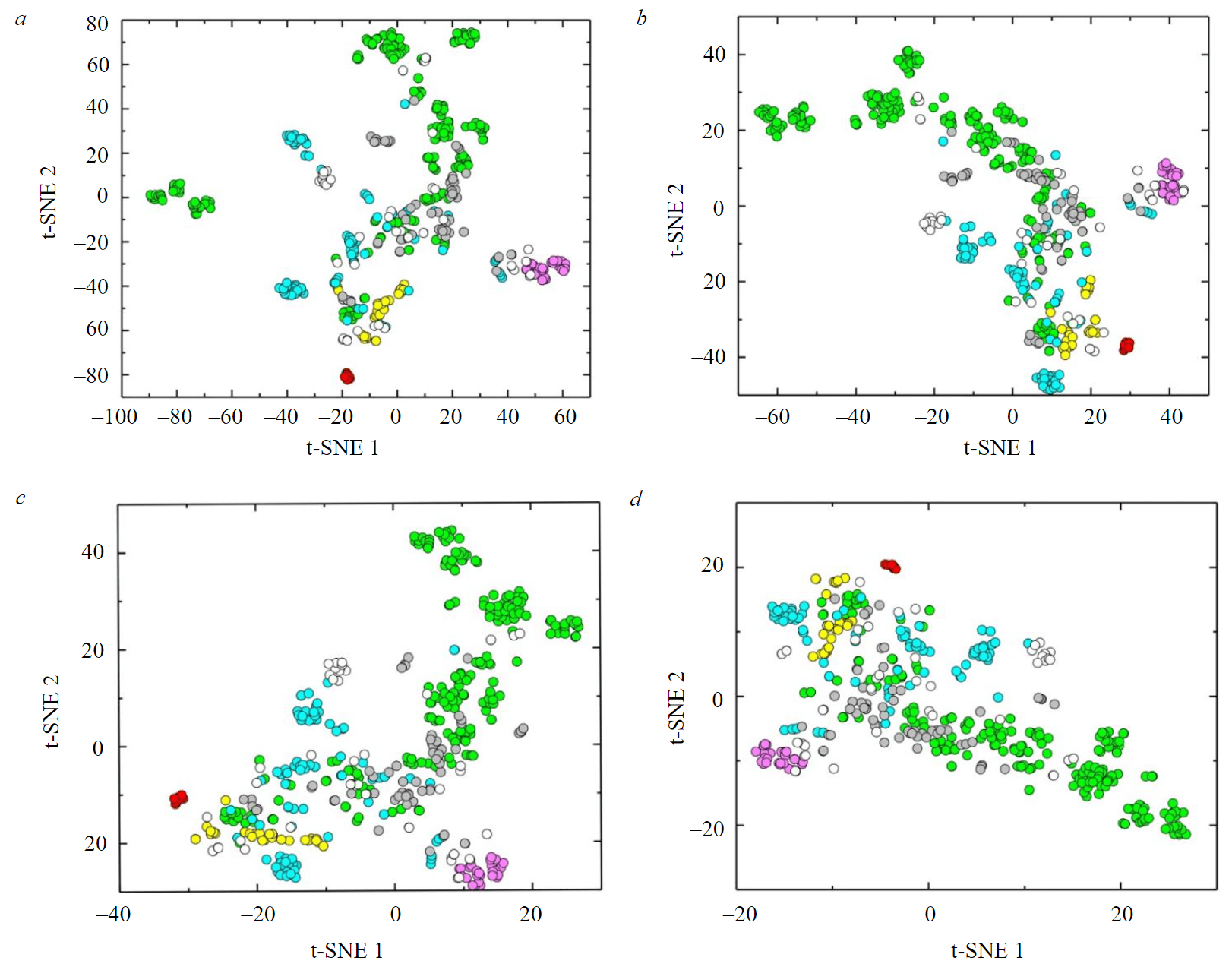
Fig.3. Visualization of beryl composition data by t-SNE method (after taking the logarithm) for perplexity values of 10 (a); 20 (b); 30 (c); and 50 (d)
On the diagram of the values of the first and second principal components (Fig.2, b), the right half of the graph is mainly occupied by the points of compositions of green beryls with the maximum positive values for the first principal component. The largest (modulo) negative values of the first principal component are established for imaging points of pink, colorless, yellow, and red beryls. According to the values of the first principal component, white beryls and aquamarines occupy an intermediate position between the listed varieties and green beryls. The fields of compositions of pink beryls (positive values) and red beryls (negative values) are mostly distinguished by the second principal component.
In the coordinates of the first and third (weight 12 %) principal components (Fig.2, c), the maximum modulo negative loads on the third principal component are Co (–0.61), water (–0.57), and fluorine (–0.47). Positive loads are set for Rb (0.55), Cs (0.41), K (0.42), and Ti (0.44).
In the diagram of the values of the first and third principal components (Fig.2, d), the imaging points of red beryls are sharply separated by the positive values of the third principal component. The field of pink beryl compositions is the closest to them.
The principal components analysis for CLR–transformed primary data showed that the first principal component separates the imaging points of green beryls from other varieties. The second principal component separates pink and red beryls, while the third principal component separates the red beryls from the other varieties. In general, the results of visualization of analytical data with the CLR transformation do not fundamentally differ from the similar data considered with the standard logarithm. Possible options for interpreting the principal components will also be close or even coincide for these two methods of preprocessing the same analytical data. Nevertheless, the use of the principal components analysis with the CLR transformation gave a more clear data imaging than in the case of standard logarithms.
Stochastic neighbor embedding method with t-distribution (t-SNE)
Different values of perplexity were used to visualize the logarithmic primary analytical data (except for water content) by the t-SNE method. Consider the options for visualizing data in two-dimensional space at the 10, 20, 30, and 50 perplexity values (Fig.3).
At a perplexity value of 10 (Fig.3, a), the imaging points of one variety of beryl are mostly densely grouped. Thus, the points of red beryls (seven individual analyzes) practically merge into one common point. The overall configuration of most of the points has a horseshoe shape. At the same time, green beryls are present in all parts of this figure as separate clusters with different numbers of points. In the central part of the field, which is bordered by a horseshoe, there are points of red and pink beryls. Some points of colorless beryls tend to pink ones. Aquamarines, white and colorless beryls do not form separate clusters and are scattered across the diagram field. Yellow beryls, on the contrary, are relatively compactly grouped in the upper part of the horseshoe.
At a perplexity value of 20 (Fig.3, b), the tendency to isolate point clusters belonging to different types of beryls is more clearly traced. Large clusters of points of green beryls are grouped in the upper right and left parts of the diagram; red, pink, and yellow beryls are in individual clusters adjacent to the general figure. Colorless beryls and aquamarines form clusters and are represented by points scattered across the diagram, along with white beryls. These three varieties mostly do not overlap with the compositions of green beryls.
At a perplexity value of 30 (Fig.3, c), clusters of certain varieties of beryls spread across the diagram, occupying almost half of its area. It is noticeable that specific clusters of green beryls practically do not overlap with points of other varieties. The clusters of red and pink beryls (located in close proximity to each other) are compact, while clusters of yellow beryls are rather sparse.
At a perplexity value of 50 (Fig.3, d), the figurative points of certain beryl varieties are even less compact. Compactness is preserved only for clusters of red and pink beryls that are distant from each other. The points corresponding to the yellow beryls noticeably lose unity at this parameter and do not form a compact cluster.
During t-SNE visualization of CLR-normalized analytical data, perplexity values of 10, 20, 30, and 50 were also used (Fig.4).
With a perplexity value of 10, the general pattern is the extremely close and dense location of points (Fig.4, a). Thus, the set of points of green beryls breaks up into more than 10 individual clusters. The remaining varieties of beryls mostly do not overlap with clusters of green beryls. The clusters of red (the most compact cluster) and pink beryls, with adjoining points of colorless beryls and aquamarines, are the most distant from the total set of points. The points of yellow beryls form a compact elongated area, tending to red beryls.
At a perplexity value of 20, the figurative points line up in a more regular arc, maintaining the compactness of individual clusters (Fig.4, b). Clusters of green beryls are more closely related to each other and are located mainly in the upper part of the diagram. Clusters of red and pink beryls are still isolated from the general sequence of points. The points of yellow beryls are close to each other and tend to the compositions of red beryls.
When the perplexity value is 30 (Fig.4, c), certain clusters of green beryls are scattered; in general, the points of this variety are significantly mixed with the points of other beryl groups. Nevertheless, the clusters of red and pink beryls, which are considerably distant from each other, remain compact.
At a perplexity value of 50 (Fig.4, d), the structure of the mutual arrangement of points of beryl varieties, established at a perplexity value of 30, is largely preserved. The most compact clusters, still distant from each other, are red and pink beryls. However, the points of yellow beryls cease to be a single cluster. Aquamarines, white, and colorless beryls do not form separate clusters and alternate with some green beryls, which occupy almost the entire field of the diagram and, conversely, form separate clusters.

Fig.4. Visualization of beryl composition data by t-SNE method (after CLR transformation) for perplexity values of 10 (a); 20 (b); 30 (c); and 50 (d)
Conclusions
The study of the composition of trace elements of beryl varieties based on a representative sample (469 local analyzes in 111 samples performed by the SIMS method) allowed us to establish the following patterns.
- Red beryls stand out sharply among other types with increased content of Ni, Sc, Mn, Fe, Ti, Cs, Rb, K, and B and reduced content of Na and water. Pink beryls are characterized by an increased content of Cs, Rb, Na, Li, Cl, and water with a reduced content of Mg and Fe. Green beryls show an increased content of Cr, V, Mg, Na, and water with a reduced content of Cs. A feature of yellow beryls is the reduced content of Mg, Cs, Rb, K, Na, Li, and Cl. Aquamarines are marked by an increased content of Fe and reduced Cs and Rb contents. For white beryls, increased content of Na and Li has been established. Colorless beryls do not have evident features of the trace element composition.
- The principal component analysis (PCA) with CLR transformation of primary data has shown that the first principal component tends to isolate the figurative points of green beryls from other varieties. According to the second principal component, pink beryls are separated from red ones, and according to the third one – red beryls are separated from other varieties. Using the principal components analysis with the CLR transformation proved to be more informative than using the logarithm of the data.
- The stochastic neighbor embedding method with t-distribution (t-SNE) with CLR-transformed data demonstrated the contrasting compositions of green beryls relative to other varieties. Green beryls form long elongated areas at almost any value of perplexity, which probably indicates the possibility of their formation by any type of protolith. Red and pink beryls form the most compact clusters with minimal similarity in composition. Data visualization is most effective when the perplexity values are 20 or 30.
The comparison of the results of two different multivariate statistical methods (principal component analysis and stochastic neighbor embedding with t-distribution) demonstrated their capabilities in studying the similarity of a significant number of relatively homogeneous objects (beryls of different colors) with respect to their composition of trace elements, including volatile components and water. The authors distinguished the numbers of indicator elements according to the peculiarities of their content: when visualized, they form clusters of imaging points of differently colored beryls.
In conclusion, it should be noted that such a large-scale study of beryl, covering the composition of all known varieties, would be difficult to implement without the use of the unique collections of the Mining Museum of St. Petersburg Mining University.
References
- Sergeeva L.Y., Berezin A.V., Gusev N.I. et al. Age and metamorphic conditions of the granulites from Capral-Jegessky synclinoria, Anabar shield. Journal of Mining Institute. 2018. Vol. 229, p. 13-21. DOI: 10.25515/PMI.2018.1.13
- Leontev V.I., Skublov S.G., Shatova N.V., Berezin A.V. Zircon U-Pb geochronology recorded Late Cretaceous fluid activation in the Central Aldan gold ore district, Aldan Shield, Russia: First Data. Journal of Earth Science. 2020. Vol. 31, p. 481-491. DOI: 10.1007/s12583-020-1304-z
- Skublov S.G., Rumyantseva N.A., Li Q.L. et al. Zircon xenocrysts from the Shaka Ridge record ancient continental crust: New U-Pb geochronological and oxygen isotopic data. Journal of Earth Science. 2022. Vol. 33, p. 5-16. DOI: 10.1007/s12583-021-1422-2
- Rumyantseva N.A., Skublov S.G., Vanshtein B.G. et al. Zircon from gabbroids of the Shaka ridge (South Atlantic): U-Pb age, oxygen isotope ratios and trace element composition. Proceedings of the Russian Mineralogical Society. 2022. N1, 44-73 (in Russian). DOI: 10.31857/S0869605522010099
- Skublov S. G., Li S.-K. Anomalous geochemistry of zircon from the Yastrebetskoe rare metal deposit (SIMS- and TOF-study). Journal of Mining Institute. 2016. Vol. 222, p. 798-802. DOI: 10.18454/PMI.2016.6.798
- Yatsenko I.G., Skublov S.G., Levashova E.V. et al. Composition of spherules and lower mantle minerals, isotopic and geochemical characteristics of zircon from volcaniclastic facies of the Mriya lamproite pipe. Journal of Mining Institute. 2020. Vol. 242, p. 150-159. DOI: 10.31897/PMI.2020.2.150
- Bidny A.S., Baksheev I.A., Popov M.P., Anosova M.O. Beryl from deposits of the Ural Emerald Belt, Russia: ICP-MS-LA and infrared spectroscopy study. Moscow University Geology Bulletin. 2011. Vol. 66. N 2. p. 108-115. DOI: 3103/S0145875211020037
- Karampelas S., Al-Shaybani B., Mohamed F. et al. Emeralds from the most important occurrences: chemical and spectroscopic data. Minerals. 2019. Vol. 9. Iss. 9. 561. DOI: 10.3390/min9090561
- Uher P., Chudik P., Bacik P. et al. Beryl composition and evolution trends: an example from granitic pegmatites of the beryl-columbite subtype, Western Carpathians, Slovakia. Journal of Geosciences. 2010. Vol. 55. Iss. 1, p. 69-80. DOI: 10.3190/jgeosci.060
- Aurisicchio C., Conte A.M., Medeghini L. et al. Major and trace element geochemistry of emerald from several
deposits: Implications for genetic models and classification schemes. Ore Geology Reviews. 2018. Vol. 94, p. 351-366. DOI: 10.1016/j.oregeorev.2018.02.001 - Giuliani G., Groat L.A., Marshall D. et al. Emerald deposits: A review and enhanced classification. Minerals. 2019. Vol. 9. Iss. 2. 105. DOI: 10.3390/min9020105
- Groat L.A., Giuliani G., Marshall D.D., Turner D. Emerald deposits and occurrences: A review. Ore Geology Reviews. 2008. Vol. 34, p. 87-112. DOI: 10.1016/j.oregeorev.2007.09.003
- Saeseaw S., Pardieu V., Sangsawong S. Three-phase inclusions in emerald and their impact on origin determination. Gems & Gemology. 2014. Vol. 50, p. 114-132. DOI: 10.5741/GEMS.50.2.114
- Saeseaw S., Renfro N.D., Palke A.C. et al. Geographic origin determination of emerald. Gems & Gemology. 2019. Vol. 55, p. 614-646. DOI: 10.5741/GEMS.55.4.614
- Zheng Y., Yu X., Guo H. Major and trace element geochemistry of Dayakou vanadium-dominant emerald from Malipo (Yunnan, China): Genetic model and geographic origin determination. Minerals. 2019. Vol. 9. Iss. 12. 777. DOI: 10.3390/min9120777
- Gavrilchik A.K., Skublov S.G., Kotova E.L. Trace Element Composition of Beryl from the Sherlovaya Gora Deposit, South-Eastern Transbaikalia, Russia. Proceedings of the Russian Mineralogical Society. 2021. N 2, p. 69-82 (in Russian). DOI: 10.31857/S0869605521020052
- Gavrilchik A.K., Skublov S.G., Kotova E.L. Features of trace element composition of beryl from the Uralian izumrudnye kopi. Mineralogy. Vol. 7. N 3, p. 32-46 (in Russian). DOI: 10.35597/2313-545X-2021-7-3-2
- Abdel Gawad A.E., Ene A., Skublov S.G. et al. Trace element geochemistry and genesis of beryl from Wadi Nugrus, South Eastern Desert, Egypt. Minerals. 2022. Vol. 12. 206. DOI: 10.3390/min12020206
- Nosova A.A., Narkisova V.V., Sazonova L.V., Simakin S.G. Minor elements in clinopyroxene from Paleozoic volcanics of the Tagil island arc in the Central Urals. Int. 2002, Vol. 40, p. 219-232.
- Portnyagin M.V., Simakin S.G., Sobolev A.V. Fluorine in primitive magmas of the Troodos Ophiolite Complex, Cyprus: analytical methods and main results. Int. 2002. Vol. 40, p. 625-632.
- Portnyagin M., Almeev R., Matveev S., Holtz F. Experimental evidence for rapid water exchange between melt inclusions in olivine and host magma. Earth and Planetary Science Letters. 2008. Vol. 272. Iss. 3-4, p. 541-552. DOI: 10.1016/j.epsl.2008.05.020
- Jochum K.P., Dingwell D.B., Rocholl A. et al.The preparation and preliminary characterisation of eight geological MPI-DING reference glasses for in-situ microanalysis. Geostandards Newsletter. 2000. Vol. 24. Iss. 1, p. 87-133.
DOI: 10.1111/j.1751-908X.2000.tb00590.x - Jochum K.P., Stoll B., Herwig K. et al. MPI-DING reference glasses for in situ microanalysis: New reference values for element concentrations and isotope ratios. Geochemistry, Geophysics, Geosystems. 2006. Vol. 7. Iss. 2. N Q02008. DOI: 10.1029/2005GC001060
- Danyushevsky L.V., Eggins S.M., Falloon T.J., Christie D.M.H2O abundance in depleted to moderately enriched mid-ocean ridge magmas; Part I: Incompatible behaviour, implications for mantle storage, and origin of regional variations. Journal of Petrology. 2000. Vol. 41. Iss. 8, p. 1329-1364. DOI: 10.1093/petrology/41.8.1329
- Kamenetsky V.S., Everard J.L., Crawford A.J. et al. Enriched end-member of primitive MORB melts: Petrology and geochemistry of glasses from Macquarie island (SW Pacific). Journal of Petrology. 2000. Vol. 41. Iss. 3, p. 411-430. DOI: 10.1093/petrology/41.3.411
- Shishkina T.A., Botcharnikov R.E., Holtz F. et al.Solubility of H2O and CO2-bearing fluids in tholeiitic basalts at pressures up to 500 MPa. Chemical Geology. 2010. Vol. 277. Iss. 1-2, p. 115-125. DOI: 10.1016/j.chemgeo.2010.07.014
- Sobolev A.V., Chaussidon M. H2O concentrations in primary melts from island arcs and mid-ocean ridges: Implications for H2O storage and recycling in the mantle. Earth and Planetary Science Letters. 1996. Vol. 137. Iss. 1-7, p. 45-55. DOI: 10.1016/0012-821X(95)00203-O
- Tamic N., Behrens H., Holtz F. The solubility of H2O and CO2 in rhyolitic melts in equilibrium with a mixed CO-H2O fluid phase. Chemical Geology. 2001. Vol. 174. Iss. 1-3, p. 333-347. DOI: 10.1016/S0009-2541(00)00324-7
- Rocholl A.B.E., Simon K., Jochum K.P. et al. Chemical characterisation of NIST silicate glass certified reference material SRM 610 by ICP-MS, TIMS, LIMS, SSMS, INAA, AAS and PIXE. Geostandards Newsletter. 1997. Vol. 21. Iss. 1, p. 101-114. DOI: 10.1111/j.1751-908X.1997.tbx
- Belonin M.D., Golubeva V.A., Skublov G.T. Factor Analysis in Geology. Moscow: Nedra, 1982, p. 269 (in Russian).
- Dmitrijeva M., Ehrig K.J., Ciobanu C.L. et al. Defining IOCG signatures through compositional data analysis: A case study of lithogeochemical zoning from the Olympic Dam deposit, South Australia. Ore Geology Reviews. 2019. Vol. 105, 86-101. DOI: 10.1016/j.oregeorev.2018.12.013
- Garber J.M., Hacker B.R., Kylander-Clark A.R.C. et al. Controls on trace element uptake in metamorphic titanite: Implications for petrochronology. Journal of Petrology. 2017. Vol. 58. Iss. 6, p. 1031-1057. DOI: 10.1093/petrology/egx046
- Aitchison J. The statistical analysis of compositional data. Journal of the Royal Statistical Society: Series B (Methodological). 1982. Vol. 44. Iss. 2, p. 139-160. DOI: 10.1111/j.2517-6161.1982.tb01195.x
- Pawlowsky-Glahn V., Egozcue J.J. Compositional data and their analysis: an introduction. Geological Society, London, Special Publications. 2006. Vol. 264, p. 1-10. DOI: 10.1144/GSL.SP.2006.264.01.01
- Comas-Cufí M., Thió i Fernández de Henestrosa S. CoDaPack 2.0: a stand-alone, multi-platform compositional software. CoDaWork'11: 4th International Workshop on Compositional Data Analysis., 1-5 June 2011, Sant Feliu de Guíxols, Spain. International Center for Numerical Methods in Engineering (CIMNE) Barcelona, 2011.
- Van der Maaten L., Hinton G. Visualizing data using t-SNE. Journal of Machine Learning Research. 2008. Vol. 9, p. 2579-2605.
- Wang H.A., Krzemnicki M.S. Multi-element analysis of minerals using laser ablation inductively coupled plasma time of flight mass spectrometry and geochemical data visualization using t-distributed stochastic neighbor embedding: Case study on emeralds. Journal of Analytical Atomic Spectrometry. 2021. Vol. 36, p. 518-527. DOI: 10.1039/D0JA00484G
- Honghua Liu, Jing Yang, MingYe et al. Using t-distributed Stochastic Neighbor Embedding (t-SNE) for cluster analysis and spatial zone delineation of groundwater geochemistry data. Journal of Hydrology. 2021. Vol. 597. N 126146. DOI: 10.1016/j.jhydrol.2021.126146
- Sammon L.G., McDonough W.F.A geochemical review of amphibolite, granulite, and eclogite facies lithologies: Perspectives on the deep continental crust. Journal of Geophysical Research: Solid Earth. 2021. Vol. 126. Iss. 12. N e2021JB022791. DOI: 10.1029/2021JB022791
- Kupriyanova I.I. Typomorphism of minerals. Moscow: Nedra, 1989, p. 69-85 (in Russian).
- Andersson L.O. The positions of H+, Li+ and Na+ impurities in beryl. Physics and Chemistry of Minerals. 2006. Vol. 33, p. 403-416. DOI: 10.1007/s00269-006-0086-x
- Staatz M.H., Griffitts W.R., Barnett P.R. Differences in the minor element composition of beryl in various environments. American Mineralogist. 1965. Vol. 50. N 10, p. 1783-1795.
- Popov M.P., Solomonov V.I., Spirina A.V. et al.An analysis of geochemical features of crystallization of emeralds as an approach to determine the deposit of them. News of the Ural State Mining University. 2021. Vol. 2(62), p. 16-21. DOI: 10.21440/2307-2091-2021-2-16-21
- Aurisicchio C., Fioravanti G., Grubessi O., Mottana A.Genesis and growth of the red beryl from Utah (USA). Rendiconti Lincei. 1990. Vol. 1. Iss. 4, p 393-404. DOI: 10.1007/BF03001774
- Andersson L.O. Comments on beryl colors and on other observations regarding iron-containing beryls. The Canadian Mineralogist. Vol. 57. N 4, p. 551-566. DOI: 10.3749/canmin.1900021