Anomaly detection in wastewater treatment process for cyber resilience risks evaluation
- 1 — Ph.D. Senior Researcher Saint Petersburg Federal Research Center of the RAS ▪ Orcid
- 2 — Ph.D. Senior Researcher Saint Petersburg Federal Research Center of the RAS ▪ Orcid ▪ ResearcherID
- 3 — Full-stack Developer OOO Webim ▪ Orcid
- 4 — Ph.D., Dr.Sci. Chief Researcher Saint Petersburg Federal Research Center of the RAS ▪ Orcid ▪ Scopus
Abstract
Timely detection and prevention of violations in the technological process of wastewater treatment caused by threats of different nature is a highly relevant research problem. Modern systems are equipped with a large number of technological sensors. Data from these sensors can be used to detect anomalies in the technological process. Their timely detection, prediction and processing ensures the continuity and fault tolerance of the technological process. The aim of the research is to improve the accuracy of detection of such anomalies. We propose a methodology for the identification and subsequent assessment of cyber resilience risks of the wastewater treatment process, which includes the distinctive procedure of training dataset generation and the anomaly detection based on deep learning methods. The availability of training datasets is a necessary condition for the efficient application of the proposed technology. A distinctive feature of the anomaly detection approach is a new method of processing input sensor data, which allows the use of computationally efficient analytical models with high accuracy of anomaly detection, and outperforms the efficiency of previously published methods.
Funding The research is supported by the grant of Russian Science Foundation N 23-11-20024 and Saint Petersburg Science Foundation.
Introduction
Wastewater treatment plant systems belong to critical infrastructure facilities, the sustainable functioning of which determines the safety of the population. Violations in the technological process of water treatment plants can lead to irreparable consequences for health and ecology [1, 2].
Water treatment plants are equipped with automated control systems for monitoring and timely control of water treatment processes [3-5]. The usage of such systems leads to new risks of cyber resilience breaches as a result of cyber attacks. For example, in 2000, a cyberattack was conducted on water treatment facilities in Maroochy (Australia). As a result, one million liters of untreated wastewater were discharged into the rainwater drainage system over a three-month period. Recently, a number of cyber threats targeting water treatment systems is constantly growing. In 2021, a cyberattack was launched against the Oldsmar water treatment plant in the US, the attacker managed to increase the level of sodium hydroxide in the water. In 2022, malefactors attacked South Staffs Water in the UK using the ransomware Clop.
To monitor the water treatment processes’ state, automated control systems collect and analyze various process data. This data can be used to identify anomalies caused by different threats, predict their occurrence and assess the risks associated with systems’ cyber resilience compromise. Timely detection, prediction and processing of such anomalies and related risks ensures the continuity and resilience of the technological process [6-8].
Recently, a large number of different methods have been proposed to detect anomalies in the functioning of cyber-physical objects [9], and the main focus in scientific research is done on the application of deep neural networks due to their ability to model complex nonlinear dependencies between different object parameters and reveal temporal and spatial patterns in the data [10]. In particular, in [11], a model of a variational autoencoder MTS-DVGAN based on two long short-term memory networks (LSTM-networks) is proposed to detect anomalies in data from water distribution systems and wastewater treatment facilities. The performed experiments showed that the application of such a model allows to detect deviations in the functioning of the water treatment system with a high level of efficiency (up to 97.84 %). The study [12] presents the MTAD-CAN model consisting of an autoencoder and two decoders with a coupled attention mechanism designed to extract both temporal dependencies between the parameters of a multidimensional time series and correlation relationships between the parameters themselves. Experiments with data from a water treatment plant system showed an anomaly detection accuracy of up to 92 %. Time series spectral analysis methods has also shown their efficiency in detection of defects in process equipment that can lead to breach of system’s cyber resilience [13], and in [14] a solution combining visual data analysis and machine learning methods has been proposed.
Detected anomalies can be used to assess and predict risks of cyber resilience breach of a technological process [15-17]. Event logs and network traffic, as well as data from physical sensors, are often used as input data [18-20]. To analyze and predict system’s cyber resilience risks, it is necessary to consider the probability of successful threat implementation and the possible damage in case of its success [21]. To determine the probability of successful implementation of threat, various threat models have been proposed [22-24]. To define a threat model, it is necessary to first determine the model of the analyzed system or technological process.
Threat models can be represented as graphs and Markov chains, and can be built using machine learning techniques to identify anomalies. Tabular, graph-based and probability-based methods can be used for risk estimation. One of the existing problems is the analysis of damage caused by destructive activity on water treatment systems (or other automated control systems), this stage is one of the risk components [25]. For this purpose, expert assessment of potential damage or modeling of contamination spread with subsequent consideration of the cost of its elimination can be used.
To determine cyber resilience risk, integral qualitative and quantitative metrics of risk level are used. When forming integral metrics, a tabular approach, a weighting function, a minimax approach, etc. can be used. In this case, the tasks to be solved include determination of metrics to be considered in the integral assessment, development of a methodology for their integration into the risk assessment procedure and analysis of sensitivity of the proposed integral assessment.
Threat models constructed using machine learning techniques can accurately detect and predict anomalies caused by various types of threats. However, there are two key practical challenges to their application: the existence of a dataset to train the analysis model and the availability of computational resources. Training dataset must be realistic structured data that contain annotations describing object state at different periods of time. Analysis of publicly available datasets has shown that there are very few such datasets [26, 27]. Moreover, the anomalies presented in them are trivial and their detection does not require the usage of machine learning methods [28]. One of the possible reasons for the lack of reliable, labeled datasets describing the functioning of cyber-physical systems is the lack of a unified methodology for their creation. This paper proposes a methodology for generating datasets modeling the functioning of wastewater treatment plants at the process level. It specifies stages starting with the selection of the technological process finishing with specification of the malefactor model and possible destructive activities.
The application of anomaly detection methods based on deep learning places high demands on computational resources, which are not always available in practice. Thus, the task of developing anomaly detection and prediction methods optimized for devices with limited computational resources, such as industrial microcomputers, which are not equipped with graphical processing units, while providing anomaly detection performance comparable to classical deep learning models, is highly relevant practical task. In this paper, such a problem is solved by using a special transformation of the data vector into an image, which allows the application of “lightweight” convolutional neural networks with small number of layers.
The aim of the research is to improve the accuracy of anomaly detection in wastewater treatment process for system’s cyber resilience risk assessment, considering the limitations of the available computational resources.
The research tasks include development of a methodology for detecting and assessing risks in the technological process of wastewater treatment based on machine learning; development of a methodology for generating data sets modeling the functioning of wastewater treatment facilities at the process level; development and testing of a methodology for detecting anomalies in the data flow from process equipment in real time; development of a method for transforming the input vector of data into images.
The methodology of detection and risk assessment in the technological process of wastewater treatment based on machine learning is developed. This methodology includes the stages of a training dataset generation, identification of anomalies in the data flow from process equipment in real time and calculation of dynamic risk assessments considering the detected anomalies. To identify anomalies in the technological process, the authors propose a method transforming the input data vector into images, which allows using convolutional neural networks with small number of layers.
Methods
A methodology for identification and assessment of cyber resilience risks in wastewater treatment process based on machine learning is proposed. It includes a methodology for generating a training dataset required for anomaly detection using machine learning, and a metho-dology for analyzing data from sensors of the wastewater technological process.
Methodology for identification and assessment of cyber resilience risks in the wastewater treatment process
It is based on the analysis of data received from the automated control system of the wastewater treatment process (Fig.1). The methodology includes two modes of application – design mode and operation mode – and three main stages – train dataset generation, anomaly detection and cyber resilience risk assessment. The stage of dataset generation is represented by the methodology of training dataset generation. The output of the methodology is a train dataset that serves as input data for the next stage – training analytical models to detect anomalies in the technological process in the design mode, this stage is represented by the anomaly detection methodology. In operation mode, the trained models and data from sensors/actuators of the process control system are used to detect anomalies in data streams from sensors. The output data of this stage are the identified anomalous processes and sensors/actuators. In turn, this data serve as the input for the next step – cyber resilience risk assessment, represented by the cyber resilience risk assessment metho-dology proposed for the wastewater treatment process.
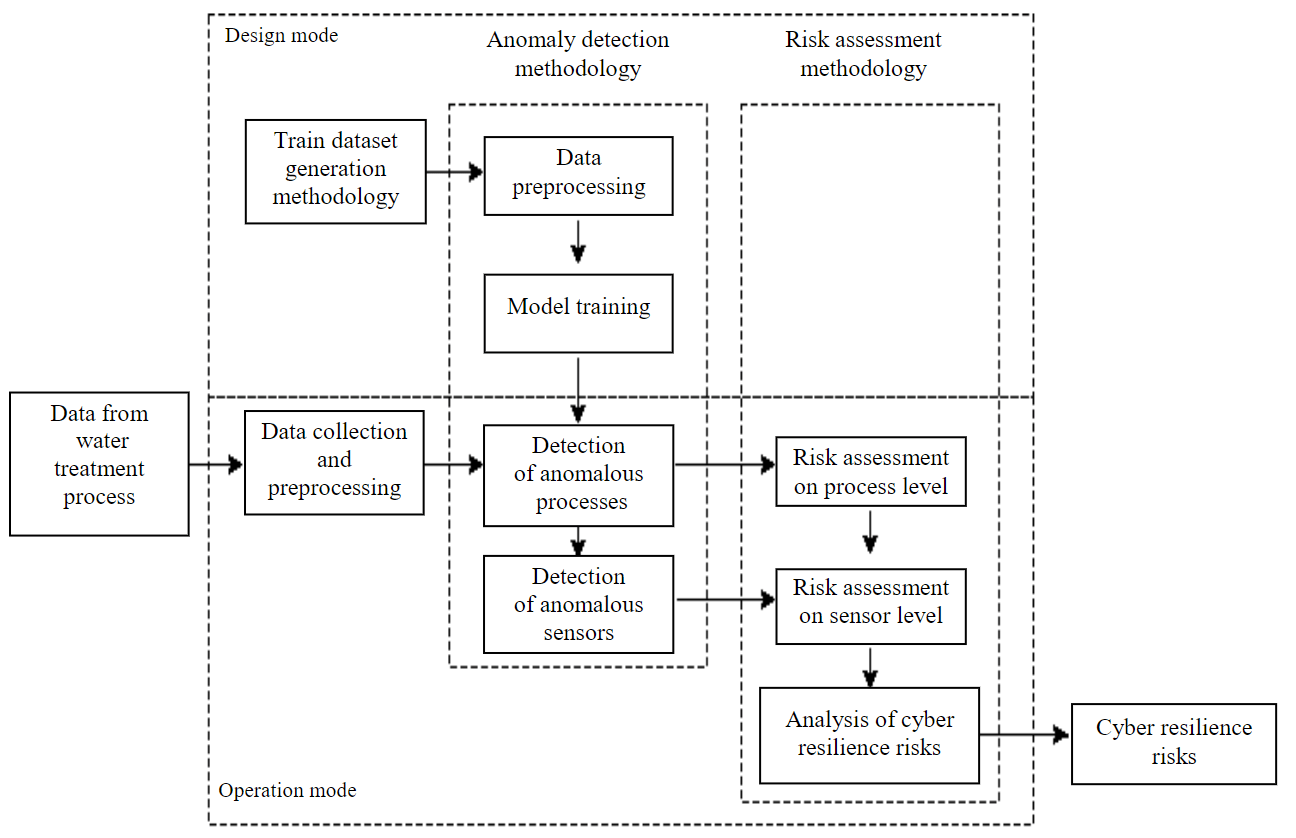
Fig.1. Scheme of methodology for identification and assessment of risks in the wastewater treatment process
Methodology of training dataset generation
The developed methodology takes into account the requirements formulated in [27-29]:
- Inclusion of data from both technological equipment and the communication and computing infrastructure of the system, i.e., network traffic data, logs of automated control systems, etc.
- Availability of annotations and a structured annotation scheme that explains the annotations and includes information about anomalies in the technological process, including the possible cause – intentional or unintentional impact on the process.
- Closeness of generated data to real world data, and limitations, due to the use of simplified mathematical models or the limited hardware capabilities should be documented.
Thus, the methodology for generating datasets modeling the functioning of the technological process consists of the following steps:
- process definition and specification;
- determination of the type of testbed and its implementation;
- generation of data consistent with the normal operation of the system;
- development of a threat model to continuity and fault tolerance of the technological process taking into account the possible consequences of their violation;
- development of scenarios of threat implementation considering the used technological stack for modeling the technological process;
- implementation of threat scenarios and collection data;
- evaluation and validation of the generated dataset.
The methodology defines the input and output of each step, including documentation, since each step depends on the result of the previous one. In addition, it allows, if necessary, to reproduce the modeled process, validate the obtained data and explain the obtained results. For example, during the first stage of the methodology a formal model of the technological process with a given set of significant parameters affecting its continuity and fault-tolerance and a technological scheme are formed. Based on the results of this stage, the type of the test bed to be created is determined. According to [27], there are three types of test beds: software (virtual), hardware and hybrid. The software only is used to design virtual test beds for modeling the technological process. Such test beds have a low cost of implementation and a high level of reproducibility. In some cases, for example, when modeling processes performed in hazardous conditions, the use of such stands is the only possible solution. However, mathematical modeling of many processes is an extremely difficult task, for example, when describing the flotation process of water treatment it is necessary to build a hydrodynamic model of the process with a large number of controls and monitored variables taking into account the physical and chemical interaction of substances [30, 31], so the results of their use may be less accurate and reliable. Hardware test beds are built using specialized equipment and software, so the data obtained with their help are more reliable. They reflect possible delays and inaccuracies in the data arising from the use of physical devices and sensors. A significant disadvantage is the cost of developing such test beds and, as a consequence, the low level of reproducibility. Hybrid test beds are a compromise between software and hardware test beds. They are partly constructed using software tools and technological equipment. Thus, at the second stage of methodology, the implementation characteristics of the experimental test bed are elaborated, as well as the format of collected data and the interval of their acquisition are determined.
The results of the first step also have impact on definition of threat model and malicious scenarios. For example, the following potential attacker’s targets can be identified for the flotation process of water treatment: stopping raw water mixing; damage to raw water flow meter, reagent pump, acidity sensors; lack of coagulant in the tank, flocculant in the tank, soda in the tank, etc. Threats can be implemented at the physical, information and communication level. The physical level includes physical devices such as water flow sensors, air flow sensors, digital converters, controllers and telecommunication equipment. The targets at the information and communication level are represented by information flows from sensors to controllers, and from controllers to SCADA-system, etc, while the targets at the logical components’ level are firmware and software.
The final stage includes evaluation and validation of the generated dataset. They include statistical analysis of the obtained data, and if real data are available, comparative analysis of genera-ted and real data by evaluating the difference between the probability distributions of real and synthetic data.
The collected dataset can be used to analyze technological data.
Anomaly detection technique based on the transformation of tabular data into an image
The proposed technique is based on the idea of using convolutional neural networks, which are good at extracting spatial relationships between attributes. Their application requires transforming the input data vector to a two-dimensional matrix, since the two-dimensional convolution operation extracts spatial relationships better. Let v‾={v0, v1,…vk } be the input vector of values from k analyzed sensors and actuators of the system, then the proposed methodology consists of the following steps:
- transformation of each one-dimensional feature vector into a two-dimensional matrix Imv, usually considered as a grayscale image;
- anomaly detection using convolutional neural network (CNN).
The key steps of the methodology are presented in Fig.2.
To construct an image based on a one-dimensional vector v‾, the following steps are performed:
- initial preprocessing of feature values, which consists in normalizing them to the range [0, 1] for numeric attributes or one-hot encoding the values for categorical attributes;
- preprocessed feature values are interpreted as 8-bit values for a grayscale image;
- layout pixels according to a given algorithm into an image.
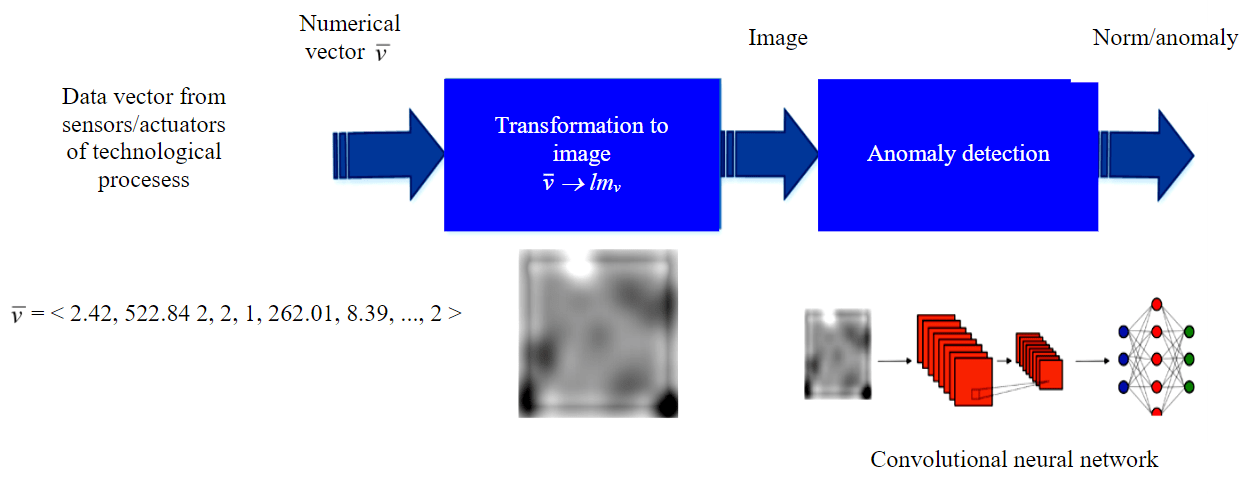
Fig.2. Diagram of anomaly detection in technological processes
To determine coordinates of attribute-pixels direct layout algorithms as well as algorithms can be used based on a nonlinear transformation depending on similiarity of attributes [32-34].
The direct pixel layout algorithm is the most common approach to image generation. The key point in its operation is to determine the dimensionality of the generated image. Usually the input for convolutional neural networks are n x n images, so the following approach is proposed to determine the image size. Let N be the number of numerical attributes to be analyzed and M be the number of values that all categorical attributes can take, then n = ceil (N + M)/2, where ceil is the rounding function to the nearest integer. The image is then generated line by line, with each row generated sequentially. Unused pixels are usually filled with the value 0x00 (black). Thus, in direct pixel layout, the order of features in the vector and the shape of the image determine the position of the corresponding pixel in the image, as a result the neighboring pixels may have no correlation with each other.
Application of algorithms based on non-linear transformation, such as DeepInsight [33, 35] allows taking into account the relationships between features and placing similar attributes close to each other. The basic idea is to use techniques for projecting multidimensional data into a lower dimensional space to determine the position of a given feature on a two-dimensional plane. The scheme of this approach is presented in Fig. 3.
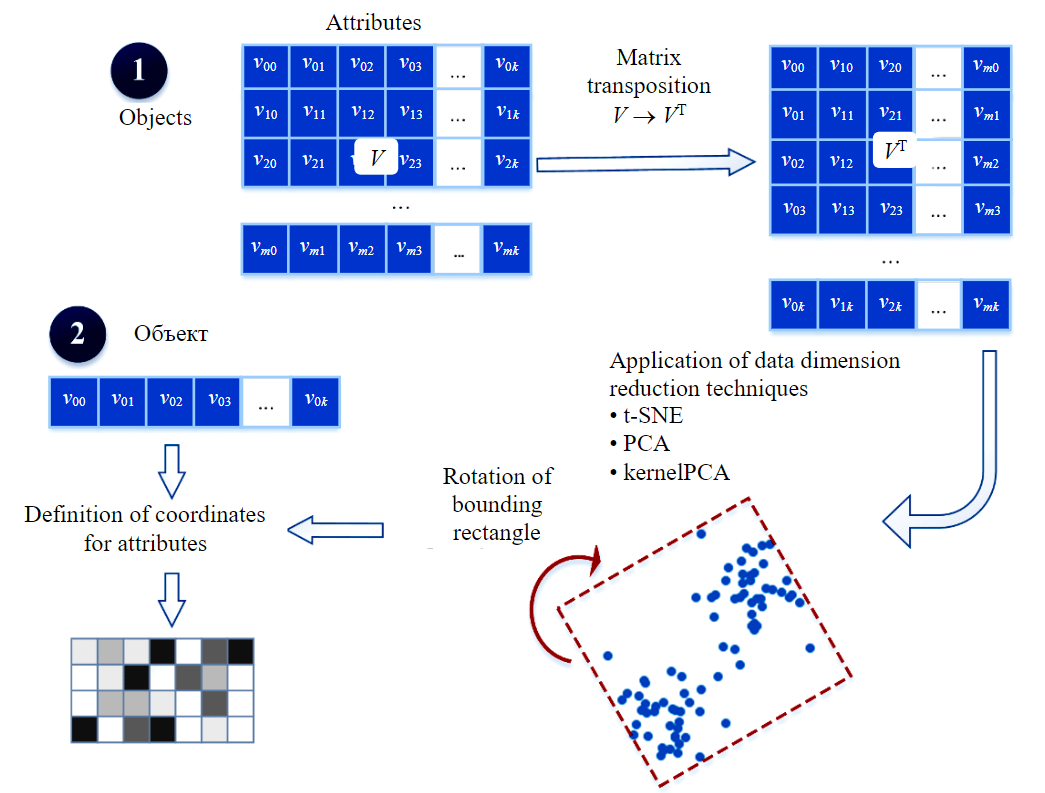
Fig.3. Scheme for determining attribute coordinates using nonlinear transformation
Let
- Transposion of the matrix V so that each feature is represented by a vector of m elements, i.e. the corresponding row of the transposed matrix VT.
- Application of data dimension reduction technique to map each feature to a two-dimensional plane. This step uses nonlinear dimension reduction techniques such as kernelPCA, t-SNE [36]. The resulting projections determine the position of the features, but not their values.
- Construction the smallest rectangle that bounds all attribute projections and rotation of their coordinates in Cartesian space to get the pixel coordinates for the features.
- Matching attributes to pixel coordinates in the generated projection.
The transformation of tabular data into a matrix structure (image) is characterized by additional computational and time costs required to determine the location of features on the image grid. However, this transformation is performed only at the training stage and does not affect the computational and time characteristics of the real-time anomaly detection process.
To detect anomalies, it was proposed to use a two-layer convolutional neural network, the structure of which is shown in Fig.4. It consists of two convolutional and two fully connected layers. The ReLU function is used as the activation function in the subsampling layers and the sigmoid function is used in the last fully connected layer. The Adam optimizer is used in training and the logarithmic loss function is used as the loss function.
Methodology for assessing cyber resilience risks of wastewater treatment process
Detected anomalies in wastewater treatment processes can lead to cyber resilience breaches. Therefore, they are used to assess risks of breach. In this case, the presence of a single anomaly does not indicate the realization of cyber resilience risks, the risk score grows with the increase in the number of anomalies. Note that risks are identified for processes and individual sensors/actuators measuring various process parameters. The cyber resilience risks are defined based on the criticality of the processes and, therefore, the damage that can be caused to the water treatment process in the case of a breach of process’s cyber resilience.
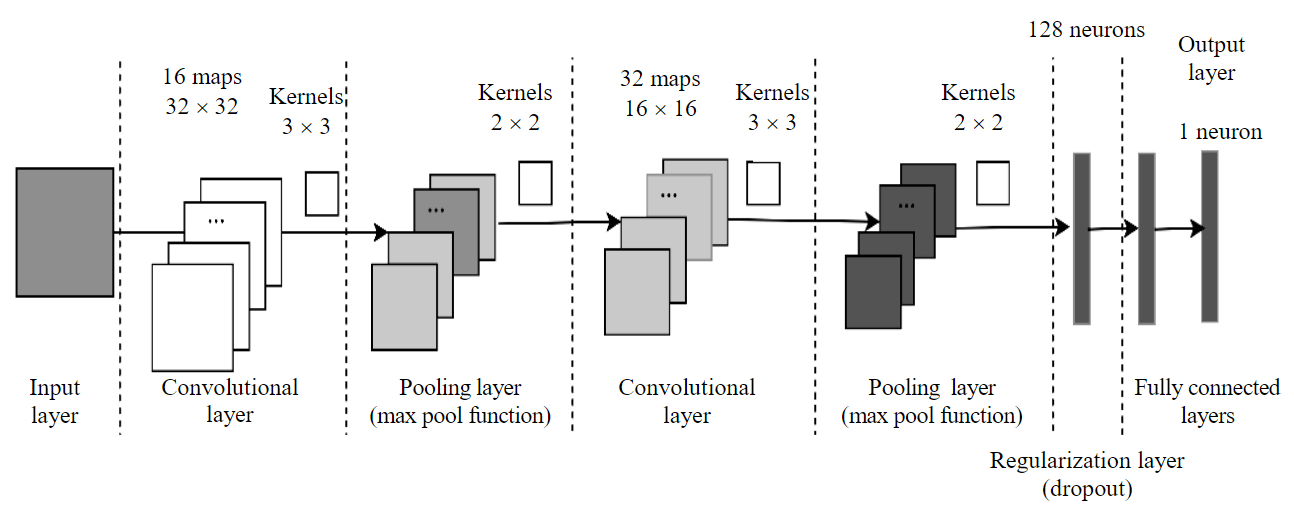
Fig.4. Structure of convolutional neural network used for anomaly detection
The input data for the methodology are the identified anomalies, as well as a description of wastewater treatment processes, their criticality and sensors/actuators measuring various technological parameters. An algorithm is developed to assess cyber resilience risks. It is based on the following assumptions: the risk level is higher for processes that are more likely to exhibit anomalous behavior; the risk level is higher for more critical processes. In addition, a model of the analyzed system is introduced:
where Pi – wastewater treatment process, i ∈ {1:n}; n – number of processes implemented in wastewater treatment system; Si – set of sensors/actuators; Pri – probability of anomaly in process Pi; Cri – criticality of process Pi, Cri ∈ {1,2,3}, determined expertly on a scale: 1 – low, 2 – high, 3 – critical; Ri – risk, Ri ∈ {0:3}.
Risk is determined by a combination of the criticality of the process and the probability of cyberthreat realization, which depends on the number of anomalies detected. Note that each anomaly also has a probability associated with the confidence score produced by machine learning algorithms. The inputs to the algorithm are the total number of records containing sensor/process actuator readings collected over the entire monitoring period; the number of consecutive records allocated for analysis, called a batch, m; and the total number of batches over the entire monitoring period n. The risk level R is defined on the scale [0, 6], initially R = 0. Algorithm for calculating the risk level is as follows:
- Pr = 0 // the initial probability of cyber threat realization.
- R = 0 // the initial level of risk.
- If R = 0, go to step 4, otherwise go to step 5 // if the risk for the process has not yet been assessed (n = 0).
- R = R_proc = Cr // risk is calculated based on the criticality of the process.
- If n = 0, Pr = 0, go to step 9.
- If wi> 0, go to step 7, otherwise go to step 10 // there were anomalies in the current batch.
- w
– sum of anomaly probabilities for anomalous batches, from the batch we take max Pr; w – share of batches with anomalies, 0 ≤ w ≤ 1. - If Pr+ logn(1 + ) ≤ 1, Pr = Pr + logn(1 + w), otherwise Pr = 1 // the likelihood of a cyber threat materializing is increasing.
- R = R + Cr∙ Pr.
- If norm > N_norm,go to step 11 // norm – number of consecutive batches without anomalies, N_norm = 10.
- coeff = (n – an)/n // an – total number of batches with anomalies.
- If coeff > R_coeff, go to step 13, otherwise go to step 14 // R_coeff – coefficient determining the maximum possible risk reduction, R_coeff = 0.3, experimentally determined.
- coeff = R_coeff.
- Pr = Pr – coeff.
- R = R – Cr∙ Pr.
The outputs of the methodology are scores for cyber resilience risks for individual processes, sensors/actuators and the wastewater treatment process as a whole.
Discussion of results
Anomaly detection is one of the most important steps in detecting and assessing cyber resilience risks in wastewater treatment process. An open dataset which was gene-rated using the methodology the closest to the proposed by the authors was used as a train dataset. Secure Water Treatment (SWaT) dataset [37] was created using a hardware-software testbed, which represents a reduced copy of a real system for water treatment and disinfection. The simulated process consists of six consecutive sub-processes: raw water intake, addition of necessary chemicals to it, filtration, dechlorination using ultraviolet (UV) lamps, feeding into the reverse osmosis system, and removal of clean water and sludge. Fig.5 shows the scheme of the modeled technological process, subprocesses are marked by letters P1-P6, respectively.
The communication part of the SWaT test bed consists of a multilevel communication network, programmable logic controllers, supervisory control and data acquisition (SCADA) server and workstation, as well as historian server. The test bed architecture allows operational personnel to connect remotely to the facility infrastructure. The dataset has several versions, differing in the types of data collected, the disruptive impacts conducted and the total duration of the test bed functioning.
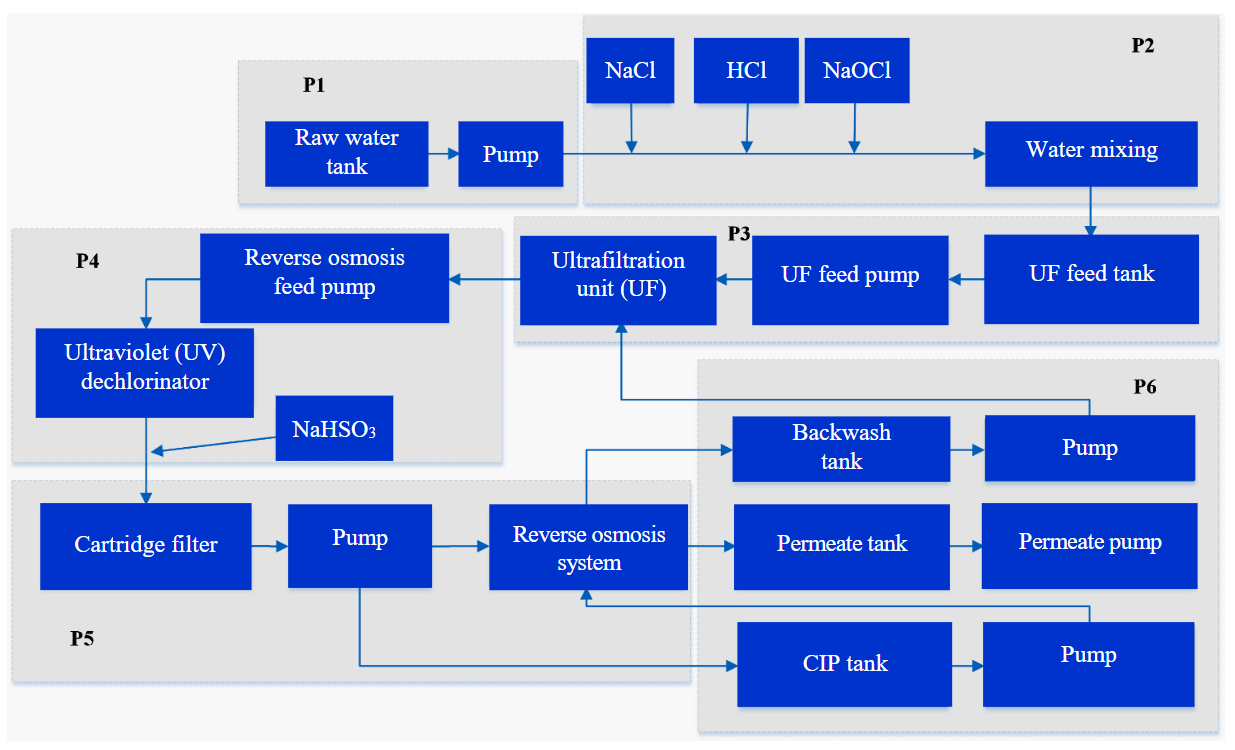
Fig.5. Diagram of the technological process modeled by the SWaT testbed [37]
In the performed experiment, a variant of the dataset containing 36 different attack scenarios for spoofing the transmitted data was used. The destructive activity targeted different physical devices belonging to different technological sub-processes. The dataset contains 19 attacks modifying the values of one sensor, six attacks spoofing the values of two and three sensors; and seven attacks on sensors belonging to different technological subprocesses (Table 1).
Table 1
Characteristics of anomalies in SWaT dataset
Type of record in dataset |
Number of processes targeted for destructive impact |
Processes |
Number of records |
Norm |
0 |
0 |
399157 |
Anomaly |
1 |
P1 |
4053 |
1 |
P2 |
1809 |
|
1 |
P3 |
37860 |
|
1 |
P4 |
1700 |
|
1 |
P5 |
1044 |
|
2 |
P3, P4 |
1691 |
|
2 |
P1, P3 |
1445 |
|
2 |
P3, P6 |
697 |
|
2 |
P4, P5 |
463 |
To evaluate the performance of anomaly detection, we used the following metrics: precision and recall. The precision metric determines the proportion of records that are classified as anomalous and are true anomalous. The recall metric reflects the ratio of anomalous records that are detected by the algorithm correctly. The higher the values of these metrics, the better the anomaly detection performance. Since the dataset under study is unbalanced, i.e., the number of normal records far exceeds the number of anomalous records, the F1-measure, which is the harmonic mean between precision and recall, was also used. The plots of accuracy and loss functions are shown in Fig.6. An analysis of the inference time and the number of model parameters was performed. The latter metrics allow estimating the required computational resources. The authors also performed a comparative analysis of the results with methods presented in the literature, namely solutions based on autoencoder MTS-DVGAN [12] and MTAD-CAN [13], as well as long short-term memory neural network (LSTM) and autoencoder with 1-class support vector machine (deepSVDD), trained on the same dataset (Table 2). It should be noted that there is no information on the inference time and number of model parameters for the MTS-DVGAN and MTAD-CAN models, so a dash is indicated in the columns of the table.
The experiments show that the proposed anomaly detection model shows the highest recall, while the precision is only slightly inferior to the MTS-DVGAN model. At the same time, the proposed solution is characterized by a small number of parameters, and the inference time for one record is 2.07∙10–5. Although these data are not available for the MTS-DVGAN model, however taking into account the architecture of the neural networks used in it – variational autoencoder with two short long memory networks – it is possible to assume that the number of parameters is not less than the parameters of the LSTM model. Consequently, the proposed model has high efficiency and low computational complexity and can be used in systems with limited computational resources.
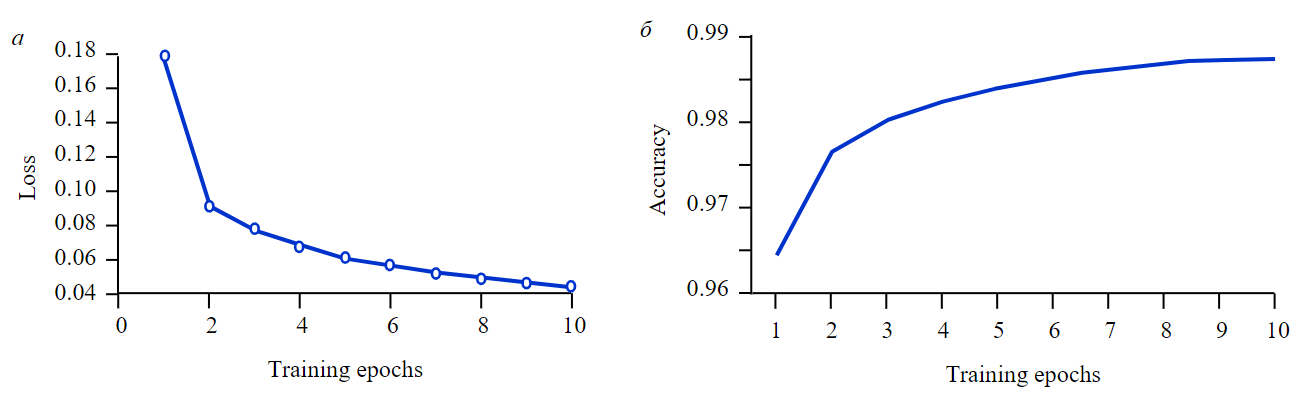
Fig.6. Loss function (а) and accuracy (б) of convolution neural network during training
Note that the models trained in the design mode are further used to detect anomalies, calculate the probabilities of successful cyber resiliency threat implementation, and assess the cyber resilience risks of the wastewater treatment process.
Table 2
Experimental results for anomaly detection in the SWaT dataset
Approach to anomaly detection |
Precision |
Recall |
F1-measure |
Number of model parameters |
Model inference time, с |
Proposed by authors |
0.98 |
0.96 |
0.97 |
10 561 |
2.07∙10–5 |
DeepSVDD |
0.95 |
0.68 |
0.82 |
11 648 |
2.43∙10–5 |
LSTM |
0.98 |
0.71 |
0.82 |
66 035 |
4.37∙10–5 |
MTS-DVGAN [10] |
0.99 |
0.93 |
0.79 |
– |
– |
MTAD-CAN [11] |
0.91 |
0.94 |
0.92 |
– |
– |
Conclusion
The paper presents the methodology for identification and assessment of cyber resilience risks of wastewater treatment process. The stages of the methodology, including the generation of a training data set, anomaly detection and risk assessment are described. Each stage is presented by a separate methodology. The stage of anomaly detection in the technological process is the key stage, since the risk assessments depend on its performance. A new approach to preprocessing the input data for subsequent anomaly detection is proposed. The experiments have shown that this approach uses fairly simple neural network with low inference latency, which allows processing intensive data streams. Due to the small number of model parameters, it can be used in devices with limited computational resources, such as industrial controllers, microcomputers, etc. Thus, the research goal of improving the accuracy of anomaly detection in the wastewater treatment process for system’s cyber resilience risk assessment, considering the limitations of the available computational resources, has been achieved.
Further directions of research work relate to the optimization of the image generation procedure by determining a sufficient amount of data samples, investigation of approaches on data from technological processes and development of methods for detecting anomalies in the processes themselves. Experiments with the calculation of cyber resilience risks as well as generation of own dataset are planned.
References
- Balaram V., Copia L., Saravana Kumar U. et al. Pollution of water resources and application of ICP-MS techniques for monitoring and management – A comprehensive review. Geosystems and Geoenvironment. 2023. Vol. 2. Iss. 4. N 100210. DOI: 10.1016/j.geogeo.2023.100210
- Chukaeva M., Petrov D. Assessment and analysis of metal bioaccumulation in freshwater gastropods of urban river habitats, Saint Petersburg (Russia). Environmental Science and Pollution Research. 2023. Vol. 30. Iss. 3, p. 7162-7172. DOI: 10.1007/s11356-022-21955-8
- Safiullin R.N., Afanasyev A.S., Rezchenko V.V. The Concept of Development of Monitoring Systems and Management of Intelligent Technical Complexes. Journal of Mining Institute. Vol. 237, р. 322-330. DOI: 10.31897/PMI.2019.3.322
- Patokin D., Danilov A., Isakov A. Environmental monitoring of natural waters in the zone of impact of an enterprise producing explosives. IOP Conference Series: Earth and Environmental Science. 2020. Vol. 578. N 012038. DOI: 10.1088/1755-1315/578/1/012038
- Smirnov Yu.D., Matveeva V.A., Yakovlev N.M., Sakhabutdinova E.R. Modern wastewater treatment technologies in galvanic production: Analysis and evaluation. Gornyi Zhurnal. N 9, p. 55-60 (in Russian). DOI: 10.17580/gzh.2023.09.08
- Romasheva N.V., Babenko M.A., Nikolaichuk L.A. Sustainable development of the Russian Arctic region: environmental problems and ways to solve them. Mining Informational and Analytical Bulletin. N 10-2, p. 78-87. DOI: 10.25018/0236_1493_2022_102_0_78
- Chukaeva M.A., Matveeva V.A., Sverchkov I.P. Complex processing of high-carbon ash and slag waste. Journal of Mining Institute. Vol. 253, p. 97-104. DOI: 10.31897/PMI.2022.5
- Russkevich E.A. Violating the Rules of Centralized Management of Technical Means of Counteracting the Threats to Information Security. Journal of Digital Technologies and Law. Vol. 1. N 3, p. 650-672. DOI: 10.21202/jdtl.2023.28
- Landauer M., Onder S., Skopik F., Wurzenberger M. Deep learning for anomaly detection in log data: A survey. Machine Learning with Applications. 2023. Vol. 12. N 100470. DOI: 10.1016/j.mlwa.2023.100470
- Yuan Luo, Ya Xiao, Long Cheng et al. Deep Learning-based Anomaly Detection in Cyber-physical Systems: Progress and Opportunities. ACM Computing Surveys. 2022. Vol. 54. Iss. 5. N 106. DOI:1145/3453155
- Haili Sun, Yan Huang, Lansheng Han et al. MTS-DVGAN: Anomaly detection in cyber-physical systems using a dual variational generative adversarial network. Computers & Security. 2024. Vol. 139. N 103570. DOI: 10.1016/j.cose.2023.103570
- Feng Xia, Xin Chen, Shuo Yu. et al. Coupled Attention Networks for Multivariate Time Series Anomaly Detection. IEEE Transactions on Emerging Topics in Computing. Vol. 12. Iss. 1, p. 240-253. DOI: 10.1109/TETC.2023.3280577
- Zhukovskiy Y., Buldysko A., Revin I. Induction Motor Bearing Fault Diagnosis Based on Singular Value Decomposition of the Stator Current. Energies. 2023. Vol. 16. Iss. 8. N 3303. DOI: 10.3390/en16083303
- Meleshko A., Shulepov A., Desnitsky V. et al. Visualization Assisted Approach to Anomaly and Attack Detection in Water Treatment Systems. Water. 2022. Vol. 14. Iss. 15. N 2342. DOI: 10.3390/w14152342
- Pliatsios D., Sarigiannidis P., Lagkas T., Sarigiannidis A. A Survey on SCADA Systems: Secure Protocols, Incidents, Threats and Tactics. IEEE Communications Surveys & Tutorials. Vol. 22. Iss. 3, p. 1942-1976. DOI: 10.1109/COMST.2020.2987688
- Cherdantseva Y., Burnap P., Blyth A. et al. A review of cyber security risk assessment methods for SCADA systems. Computers & Security. 2016. Vol. 56. P. 1-27. DOI: 10.1016/j.cose.2015.09.009
- Mehmood A., Epiphaniou G., Maple C. et al. A Hybrid Methodology to Assess Cyber Resilience of IoT in Energy Management and Connected Sites. Sensors. 2023. Vol. 23. Iss. 21. N 8720. DOI: 10.3390/s23218720
- Xirong Ning; Jin Jiang. Design, Analysis and Implementation of a Security Assessment/Enhancement Platform for Cyber-Physical Systems. IEEE Transactions on Industrial Informatics. 2022. Vol. 18. Iss. 2, p. 1154-1164. DOI: 10.1109/TII.2021.3085543
- Teixeira A., Kin Cheong Sou, Sandberg H., Johansson K.H. Secure Control Systems: A Quantitative Risk Management Approach. IEEE Control Systems Magazine. 2015. Vol. 35. Iss. 1, p. 24-45. DOI: 10.1109/MCS.2014.2364709
- Fedorchenko E.V., Novikova E.S., Kotenko I.V. The security and privacy measuring system for the internet of things devices. Voprosy kiberbezopasnosti. N 5 (51), p. 28-46 (in Russian). DOI: 10.681/2311-3456-2022-5-28-46
- Doinikova E.V., Kotenko I.V. Security assessment and selection of countermeasures for cybersecurity management. Moscow: Rossiiskaya akademiya nauk, 2021, p. 184 (in Russian).
- Jbair M., Ahmad B., Maple C., Harrison R. Threat modelling for industrial cyber physical systems in the era of smart Computers in Industry. 2022. Vol. 137. N 103611. DOI: 10.1016/j.compind.2022.103611
- Palleti V.R., Adepu S., Mishra V.K., Mathur A. Cascading effects of cyber-attacks on interconnected critical infrastructure. Cybersecurity. 2021. Vol. 4. N 8. DOI: 10.1186/s42400-021-00071-z
- Khalil S.M., Bahsi H., Korõtko T. Threat modeling of industrial control systems: A systematic literature review. Computers & Security. 2024. Vol. 136. N 103543. DOI: 10.1016/j.cose.2023.103543
- Kaixing Huang, Chunjie Zhou, Yu-Chu Tian et al. Assessing the Physical Impact of Cyberattacks on Industrial Cyber-Physical Systems. IEEE Transactions on Industrial Electronics. 2018. Vol. 65. Iss. 10, p. 8153-8162. DOI: 10.1109/TIE.2018.2798605
- Tushkanova O., Levshun D., Branitskiy A. et al. Detection of Cyberattacks and Anomalies in Cyber-Physical Systems: Approaches, Data Sources, Evaluation. Algorithms. 2023. Vol. 16. Iss. 2. N 85. DOI: 10.3390/a16020085
- Conti M., Donadel D., Turrin F. A Survey on Industrial Control System Testbeds and Datasets for Security Research. IEEE Communications Surveys & Tutorials. 2021. Vol. 23. Iss. 4, p. 2248-2294. DOI: 10.1109/COMST.2021.3094360
- Renjie Wu, Keogh E.J. Current Time Series Anomaly Detection Benchmarks are Flawed and are Creating the Illusion of Progress. IEEE Transactions on Knowledge and Data Engineering. 2023. Vol. 35. Iss. 3, p. 2421-2429. DOI: 10.1109/TKDE.2021.3112126
- Kotenko I.V., Fedorchenko E.V., Novikova E.S. et al. Data collection methodology for security analysis of industrial cyber-physical systems. Voprosy kiberbezopasnosti. N 5 (57), p. 69-79 (in Russian). DOI: 10.21681/2311-3456-2023-5-69-79
- Antonova E.S. Modeling of Wastewater Treatment Process in a Flotation Setup with Ejection Aeration System Having a Disperser. Safety in Technosphere. Vol. 6. N 1, p. 43-50 (in Russian). DOI: 10.12737/article_590199b9952dc2.23575176
- Ivanov A., Strizhenok A., Borowski G. Treatment of methanol-containing wastewater at gas condensate production. Journal of Water and Land Development. 2022. Vol. 54, p. 84-93. DOI: 10.24425/jwld.2022.141558
- Bazgir O., Zhang R., Dhruba S.R. et al. Representation of features as images with neighborhood dependencies for compatibility with convolutional neural networks. Nature Communications. 2020. Vol. N 4391. DOI: 10.1038/s41467-020-18197-y
- Sharma A., Vans E., Shigemizu D. et al. DeepInsight: A methodology to transform a non-image data to an image for convolution neural network architecture. Scientific Reports. 2019. Vol. 9. N 11399. DOI: 10.1038/s41598-019-47765-6
- Zhu Y., Brettin T., Fangfang Xia et al. Converting tabular data into images for deep learning with convolutional neural networks. Scientific Reports. 2021. Vol. 11. N 11325. DOI: 10.1038/s41598-021-90923-y
- Jong-Ik Park, Sihoon Seong, JunKyu Lee, Cheol-Ho Hong. Vortex Feature Positioning: Bridging Tabular IIoT Data and Image-Based Deep Learning: ArXiv. 2024, p. 23 (preprint).
- Yuansheng Zhou, Sharpee T.O. Using Global t-SNE to Preserve Intercluster Data Structure. Neural Computation. 2022. Vol. 34. Iss. 8. P. 1637-1651. DOI: 10.1162/neco_a_01504
- Goh J., Adepu S., Junejo K.N., Mathur A. A Dataset to Support Research in the Design of Secure Water Treatment Systems. Critical Information Infrastructures Security. Cham: Springer, 2017, p. 88-99. DOI: 10.1007/978-3-319-71368-7_8